Computational Optimization for Fast and Robust Automatic Segmentation in Virtual Microscopy using Brute-force-based Feature Selection
PROCEEDINGS OF THE INTERNATIONAL CONFERENCE ON PATTERN RECOGNITION AND ARTIFICIAL INTELLIGENCE (ICPRAI 2018)(2018)
摘要
With the fast development of large computer infrastructures and the widening of their usage, the quantity of data produced and processed in all scientific fields is rapidly increasing. In histology, this increase is due to optical microscopes allowing generation of high-resolution images, below 1 mu m(2) per pixel. Quantification of relevant biomarkers in structures of interest is crucial to understand biological processes and can be performed through image segmentation. Segmentation methods are increasingly relying on machine learning techniques, which use multiple information, or features, extracted from each pixel of the raw data. As a result, the amount of data to handle is becoming an obstacle to process large series of histological images at the whole brain level. Feature selection algorithms provide a framework to reduce the required information, but lack of algorithmic stability when dealing with real datasets. This paper presents a methodology aiming at finding a small and stable set of features, to allow fast and robust segmentation of high-resolution histological whole sections. A brute-force searching is run to extract an exhaustive list of feature sets, and the quality of subsequent segmentations is evaluated using F-Score. The selection of features is performed among the extracted sets, minimizing a criterion based on the features yielding the best results. Our work led to select one optimal set of features composed of one color feature and one textural feature working in synergy, decreasing at the same time the computation load while preserving segmentation quality. The proposed methodology can be used as a potential benchmark to evaluate other feature selection algorithms in terms of computation load and stability.
更多查看译文
关键词
Virtual microscopy, feature selection, brute-force, segmentation, color, texture, machine learning
AI 理解论文
溯源树
样例
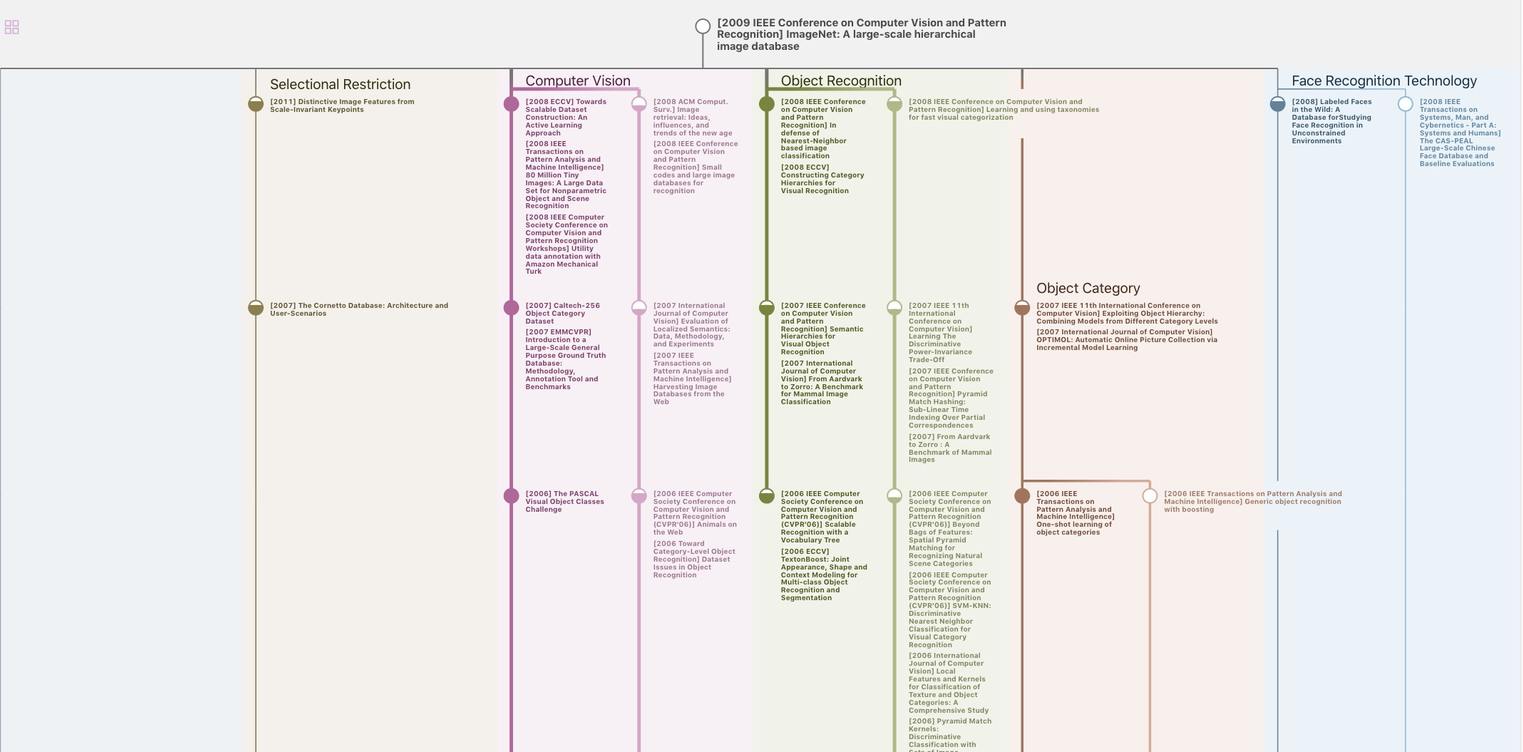
生成溯源树,研究论文发展脉络
Chat Paper
正在生成论文摘要