Face Attribute Classification with Evidential Deep Learning
FIFTEENTH INTERNATIONAL CONFERENCE ON MACHINE VISION, ICMV 2022(2023)
摘要
We address the problem of uncertainty quantification in the domain of face attribute classification, using Evidential Deep Learning (EDL) framework. The proposed EDL approach leverages the strength of Convolution Neural Networks (CNN), with the objective of representing the uncertainty in the output predictions. Predominantly, the softmax/sigmoid activation functions are applied to map the output logits of the CNN to target class probabilities in multi-class classification problems. By replacing the standard softmax/sigmoid output of a CNN with the parameters of the evidential distribution, EDL learns to represent the uncertainty in its predictions. The proposed approach is evaluated on CelebA and LFWA datasets. The quantitative and qualitative analysis demonstrate the suitability and strength of EDL to estimate the uncertainty in the output predictions without hindering the accuracy of CNN-based models.
更多查看译文
关键词
Uncertainty Estimation, Evidential Deep Learning, Face Attribute Classification, Deep Learning
AI 理解论文
溯源树
样例
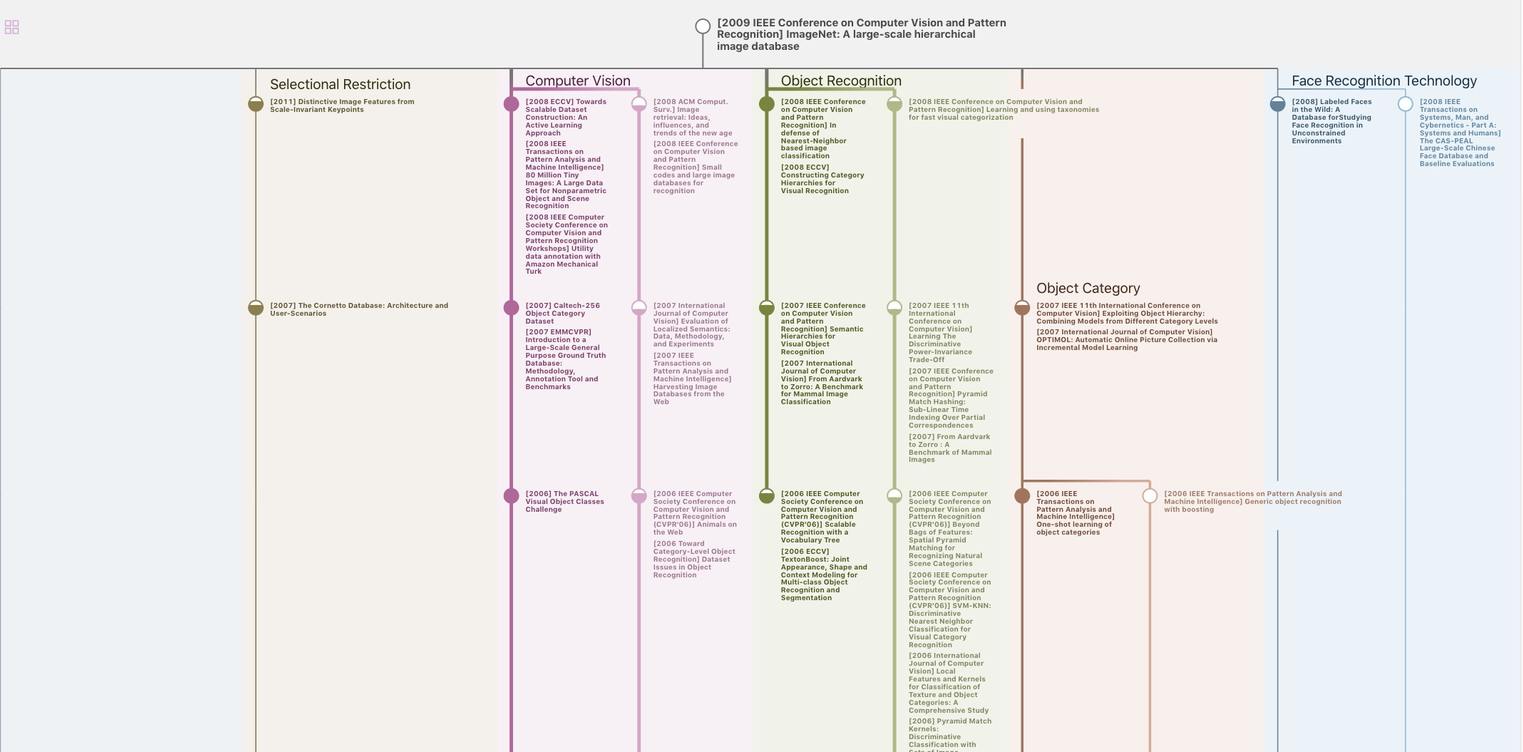
生成溯源树,研究论文发展脉络
Chat Paper
正在生成论文摘要