General fine-grained event detection based on fusion of multi-information representation and attention mechanism
INTERNATIONAL JOURNAL OF MACHINE LEARNING AND CYBERNETICS(2023)
摘要
Event extraction is an important field in information extraction, which aims to extract key information from unstructured text automatically. Event extraction is mainly divided into trigger identification and classification. The existing models are deficient in sentence representation in the initial word embeddings training process, which makes it difficult to capture the deep bidirectional representation and can’t handle the semantic information of the context well, thus affecting the performance of event detection. In this paper, a model BMRMC (BERT + Mean pooling layer + Relative position in multi-head attention + CRF) based on multi-information representation and attention mechanism is proposed. Firstly, the BERT pre-training model based on a bidirectional training transformer is used to embed words and extract word-level features. Then the sentence-level semantic representation is fused by mean pooling layer. In addition, relative position is combined with multi-head attention, which can strengthen the connection of contents. Finally, the sequences are labeled by CRF based on the BIO-labeling mechanism. The experimental results show that the proposed model BMRMC improves the performance of event detection, and the F value on the MAVEN dataset is 67.74%, which achieves state-of-the-art performance in the general fine-grained event detection task.
更多查看译文
关键词
Event detection,BERT,Multi-information representation,Attention mechanism
AI 理解论文
溯源树
样例
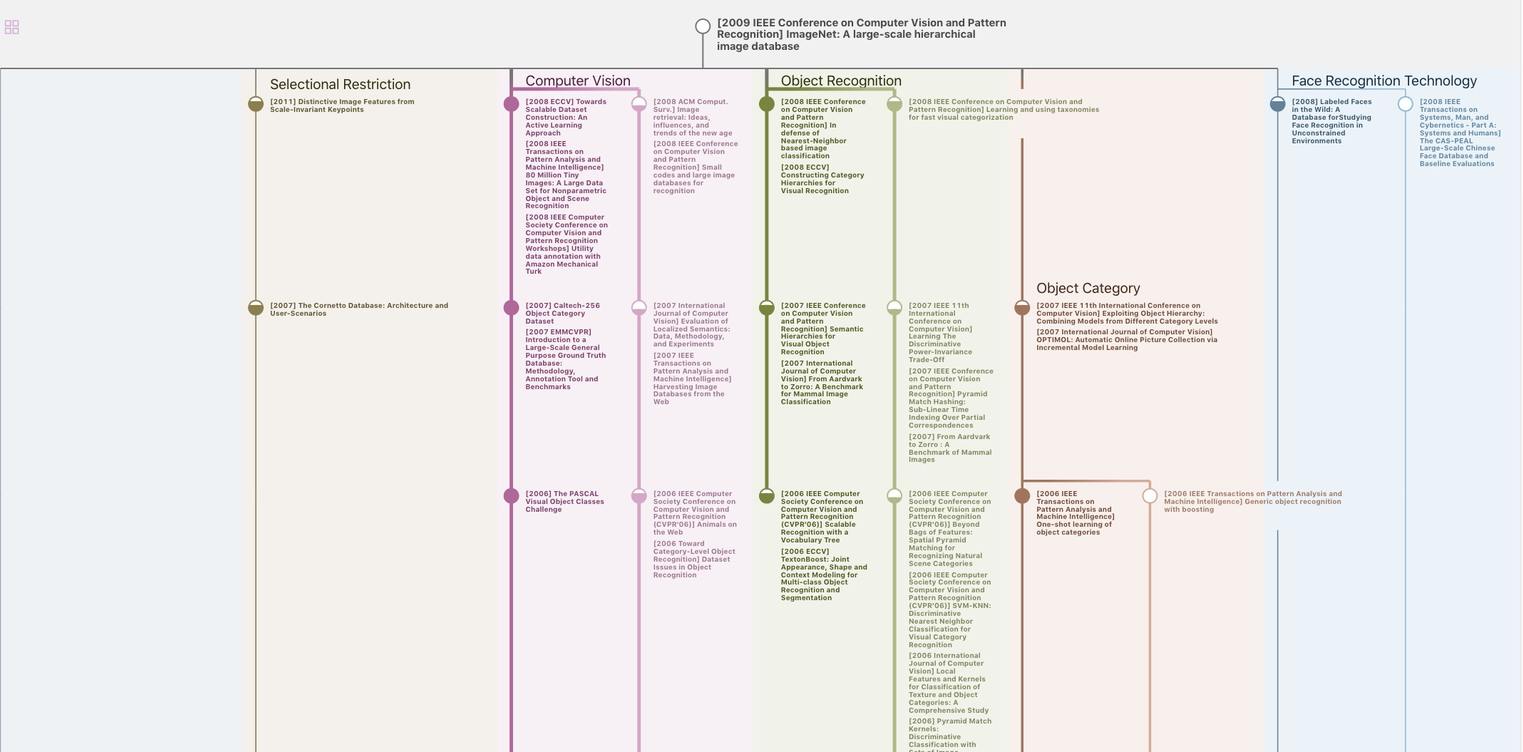
生成溯源树,研究论文发展脉络
Chat Paper
正在生成论文摘要