Delving deep into adversarial perturbations initialization on adversarial examples generation
JOURNAL OF ELECTRONIC IMAGING(2022)
摘要
Though deep neural networks ( DNNs) have achieved great success in the computer vision and pattern recognition community, studies show that they are vulnerable to adversarial examples. Adversarial perturbations, usually imperceptible to humans, can be added to benign images to form adversarial examples. Lots of gradient-based methods have been proposed to compute adversarial perturbations. However, these methods compute adversarial perturbations without initialization. A proper initialization of the perturbations is critical to the robustness of adversarial examples. To this end, we propose several adversarial perturbation initialization (API) methods for generating robust adversarial examples. Our work comprehensively analyzes the effect of adversarial perturbations initialization on several white-box attack methods. We conduct experiments on three benchmark datasets: MNIST, Cifar10, and ImageNet. Experimental results show that API improves the attack success rates of adversarial examples. The average recognition accuracy of the target model is reduced by about 3.4% when API is used to generate adversarial examples.
更多查看译文
关键词
adversarial example, perturbation initialization, adversarial attack, deep neural network
AI 理解论文
溯源树
样例
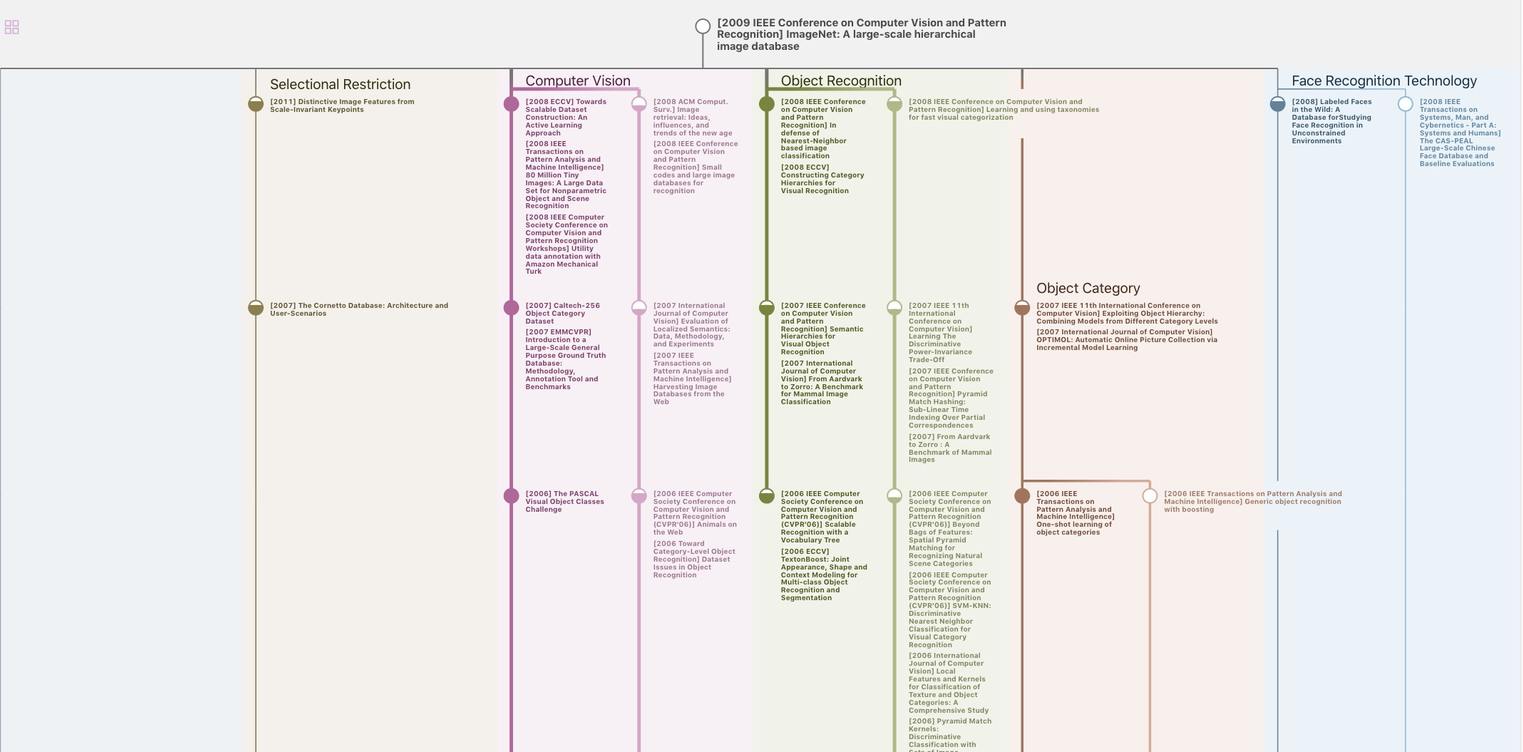
生成溯源树,研究论文发展脉络
Chat Paper
正在生成论文摘要