Accurate prediction and compensation of machining error for large components with time-varying characteristics combining physical model and double deep neural networks
JOURNAL OF MANUFACTURING PROCESSES(2023)
摘要
The accurate prediction and compensation of machining error for large components are always difficult due to the complex and time-varying intrinsic interactions during material removal process. The machining error generation of large components is directly related with localized tool-workpiece deformation, which is a function of the system stiffness and the machining force. Both system stiffness and machining force pattern can hardly be recognized and determined explicitly only by physical modeling due to the time-varying characteristics of machining process. Therefore, it is important to utilize the physical model to reflect the process fundamentals, and is necessary to calibrate these two critical variables by fusing the process data. In this paper, by investigating the large shaft precision grinding process, a hybrid 'physical model + artificial intelligence' approach is proposed. The physical model is based on the double-integral of material removal over time and space, and also considers the deformation induced by the grinding force and the grinding wheel wear evolution. Meanwhile, the double deep neural networks are built, in which a convolution neural network (CNN) is used to obtain the instant system stiffness based on process data, while the grinding force pattern of current pass is calibrated by the instant system stiffness. A long short-term memory (LSTM) network with two sub-networks is connected to CNN to predict the system stiffness and grinding force pattern for subsequent pass. The experimental results show that the proposed method can achieve higher prediction and compensation accuracy of machining error for large shaft grinding with different profiles. Furthermore, the quantitative output of the double neural networks reveals that the system stiffness decreases first and then stabilizes, and the grinding force evolution shows diverse patterns during grinding, which can be used to describe the time-varying characteristics of the grinding system during long-cycle machining process.
更多查看译文
关键词
Large components machining, Large shaft grinding, Machining error prediction and compensation, Time -varying characteristics, Physical model calibration, Double deep neural networks, Comparison of different methods
AI 理解论文
溯源树
样例
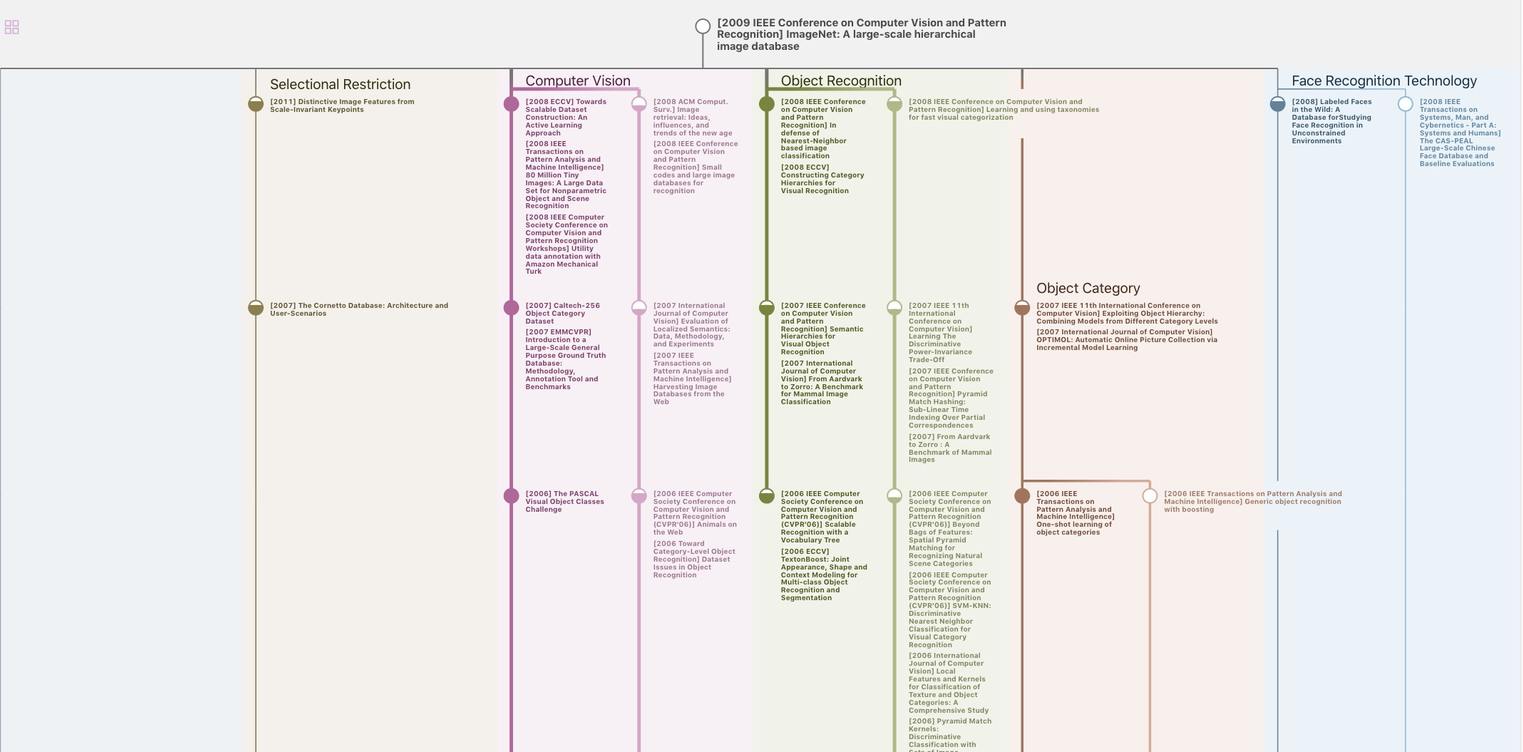
生成溯源树,研究论文发展脉络
Chat Paper
正在生成论文摘要