Multi-scale graph reasoning network for remote sensing image change detection
INTERNATIONAL JOURNAL OF REMOTE SENSING(2023)
摘要
The technology of change detection from a pair of registered satellite remote sensing images is of great importance for the study of landform changes and thus has attracted much attention. Recently, convolutional neural networks have been favoured in many applications due to their excellent feature extraction performance. However, for high-resolution satellite remote sensing images, previous methods are difficult to obtain finer details of change detection. There are many complex scenery and objects in satellite remote sensing images, which leads to the decline of the performance of the network model. In order to better solve the above problems, we propose a graph convolution-based multi-scale network with a channel attention mechanism. Specifically, we first project the initial features at different levels as vertex features, and there is a mutual relationship between the vertices. After that, we learn the correlations between these vertices through a graph reasoning. We then reproject the newly obtained vertex features to the original pixel space to obtain fine feature maps of the same size and fuse fine features of different scales through the attention fusion module. Finally, we realize the change detection of the input bi-temporal image through the feature difference module of the decoder. We separately conduct extensive experiments on three different high-resolution datasets to demonstrate that our method is effective.
更多查看译文
关键词
image change detection,graph,sensing,multi-scale
AI 理解论文
溯源树
样例
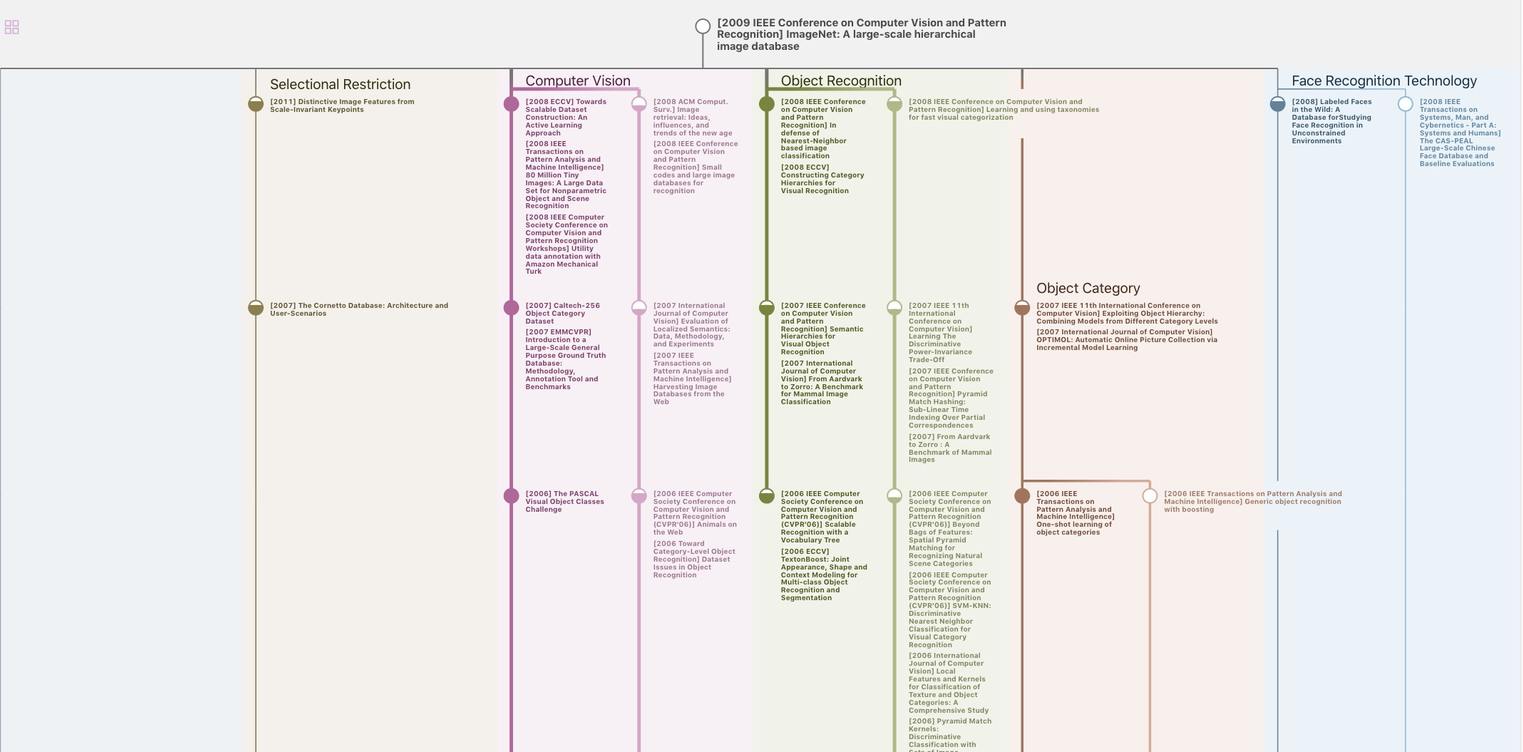
生成溯源树,研究论文发展脉络
Chat Paper
正在生成论文摘要