Large-scale system identification using self-adaptive penguin search algorithm
IET CONTROL THEORY AND APPLICATIONS(2023)
摘要
From an engineering point of view, non-linear systems are essential to the operation of control systems, because all systems actually have a non-linear state in nature. In reality, there are many different kinds of non-linear systems hidden by this negative definition. For successful analysis and control, the identification of non-linear systems using unknown models is typically necessary. Till now, numerous approaches are developed for identifying non-linear systems, but it cannot be employed with a large number of components. Moreover, system identification is typically restricted to output and input signals alone, also such systems are rarely used in reality. This is the primary justification for using non-linear systems in this research. So, this research proposed a non-linear model of system identification for large-scale systems under the consideration of two systems: bilinear system and Volterra system. Therefore, a novel algorithm named Self Adaptive Penguin Search Optimization (SAPeSO) is introduced to attain the system characteristics properly and minimize the output variation. Finally, the effectiveness of the proposed work is compared with existing works in terms of various error measures. This research mainly focuses on the application-oriented engineering problems. In particular, the Mean Absolute Error (MAE) of the proposed work for the Volterra system at 4000 samples is 18.83%, 14.05%, 8.88%, 29.72%, 19.91%, and 6.70% which is better than the existing bald eagle search (BES), arithmetic optimization algorithm (AOA), whale optimization algorithm (WOA), nonlinear autoregressive moving average with exogenous inputs- frequency response function + principal component analysis (NARMAX-FRF+PCA), Global Gravitational Search Algorithm-Assisted Kalman Filter (CGS-KF), and sparse regression and separable least squares method (SR-SLSM) methods, respectively. Finally, the error is minimum for the proposed model when compared with the other traditional approaches.
更多查看译文
关键词
adaptive systems, bilinear systems, non-linear systems, optimization
AI 理解论文
溯源树
样例
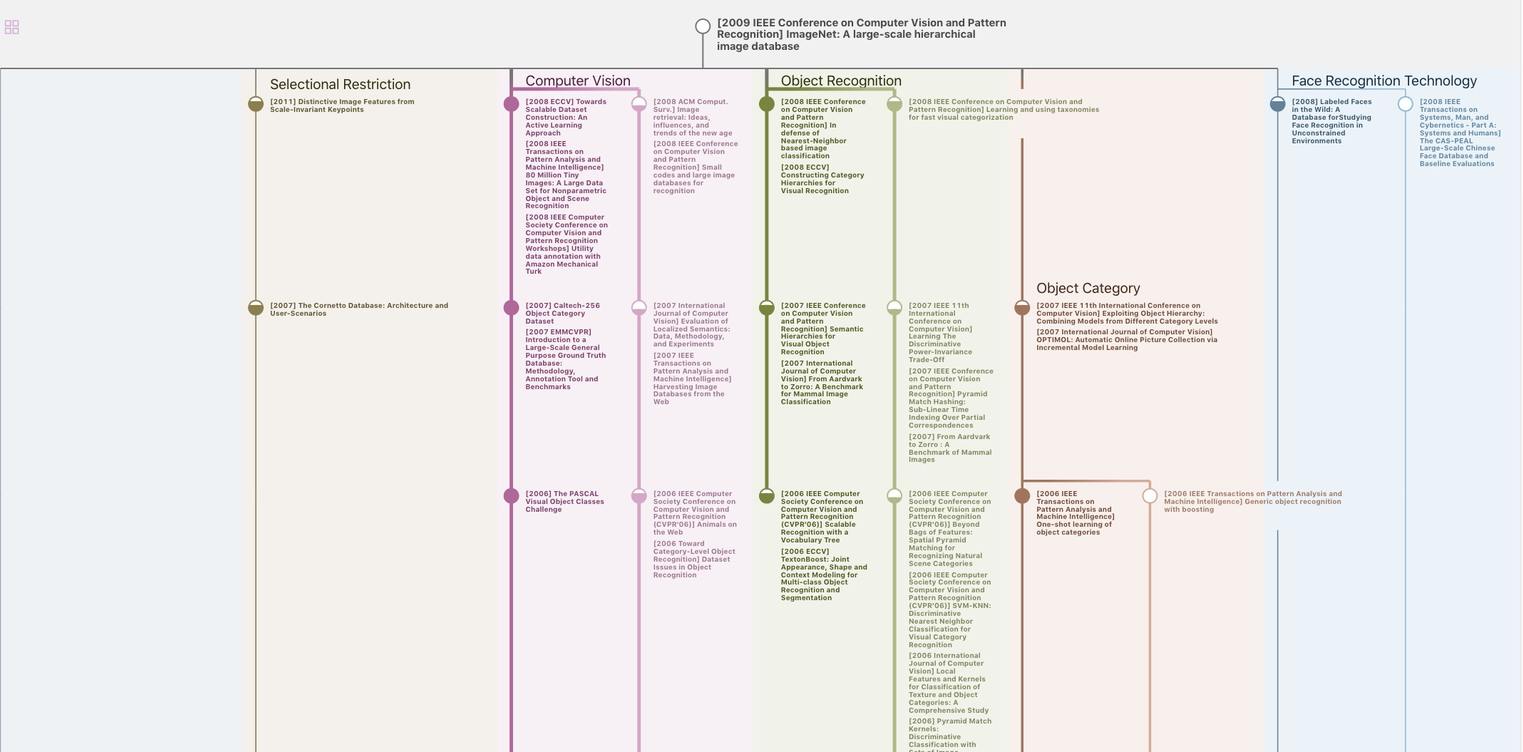
生成溯源树,研究论文发展脉络
Chat Paper
正在生成论文摘要