A Method for Predicting Surface Finish of Polylactic Acid Parts Printed Using Fused Deposition Modeling
PROCESSES(2023)
摘要
Accurately predicting the surface finish of fused deposition modeling (FDM) parts is an important task for the engineering application of FDM technology. So far, many prediction models have been proposed by establishing a mapping relationship between printing parameters and surface roughness. Each model can work well in its specific context; however, existing prediction models cannot meet the requirements of multi-factor and multi-category prediction of surface finish and cope with imbalanced data. Aiming at these issues, a prediction method based on a combination of the adaptive particle swarm optimization and K-nearest neighbor (APSO-KNN) algorithms is proposed in this paper. Seven input variables, including nozzle diameter, layer thickness, number of perimeters, flow rate, print speed, nozzle temperature, and build orientation, are considered. The printing values of each specimen are determined using an L27 Taguchi experimental design. A total of 27 specimens are printed and experimental data for the 27 specimens are used for model training and validation. The results indicate that the proposed method can achieve a minimum classification error of 0.01 after two iterations, with a maximum accuracy of 99.0%, and high model training efficiency. It can meet the requirements of predicting surface finish for FDM parts with multiple factors and categories and can handle imbalanced data. In addition, the high accuracy demonstrates the potential of this method for predicting surface finish, and its application in actual industrial manufacturing.
更多查看译文
关键词
fused deposition modeling, surface finish, adaptive particle swarm optimization algorithm, K-nearest neighbor algorithm, multi-category prediction
AI 理解论文
溯源树
样例
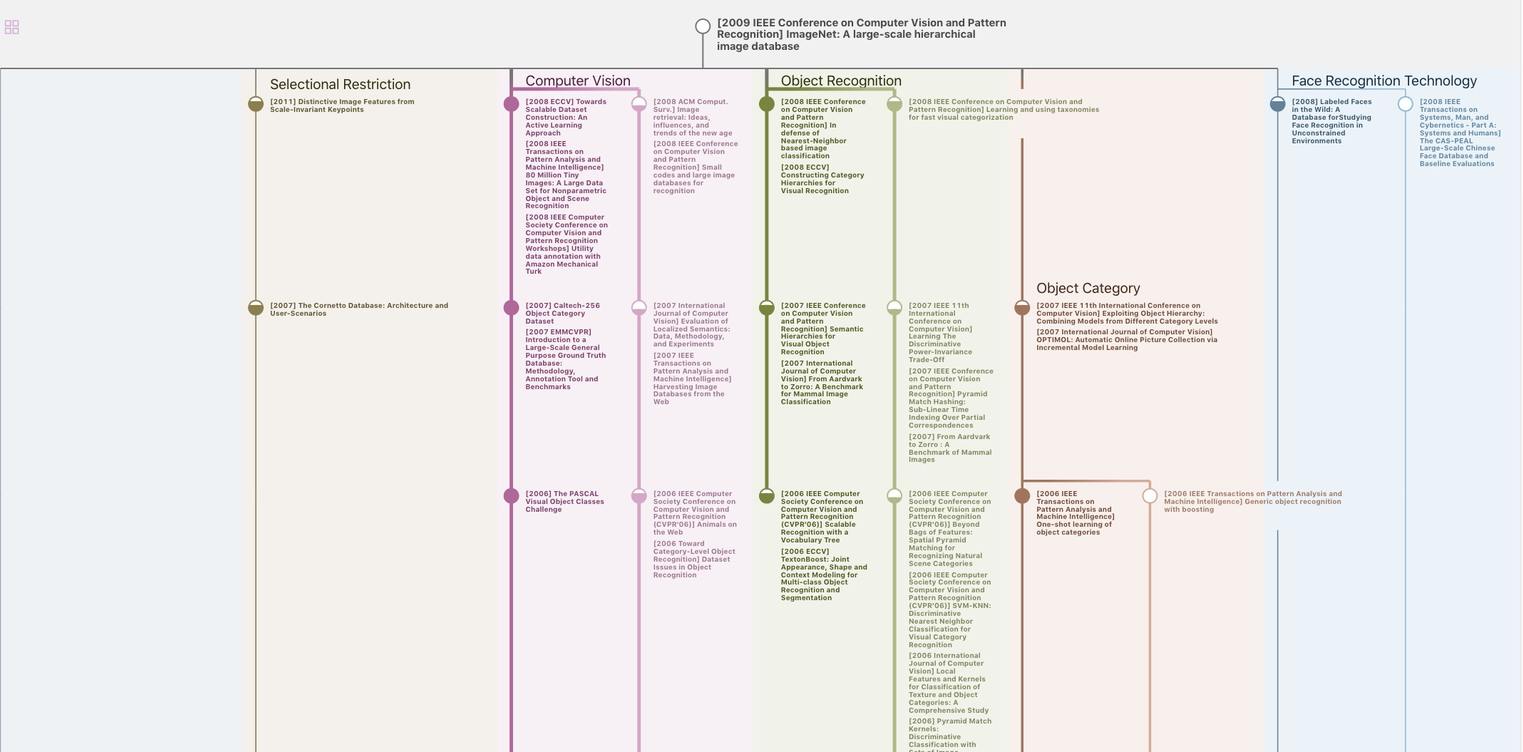
生成溯源树,研究论文发展脉络
Chat Paper
正在生成论文摘要