A Mobile Application-Classifying Method Based on a Graph Attention Network from Encrypted Network Traffic
ELECTRONICS(2023)
摘要
Classifying mobile applications from encrypted network traffic is a common and basic requirement in network security and network management. Existing works classify mobile applications from flows, based on which application fingerprints and classifiers are created. However, mobile applications often generate concurrent flows with varying degrees of ties, such as low discriminative flows across applications and application-specific flows. So flow-based methods suffer from low accuracy. In this paper, a novel mobile application-classifying method is proposed, capturing relationships between flows and paying attention to their importance. To capture the inter-flow relationships, the proposed method slices raw mobile traffic into traffic chunks to represent flows as nodes, embeds statistical features into nodes, and adds edges according to cross-correlations between the nodes. To pay different attention to the various flows, the proposed method builds a deep learning model based on graph attention networks, implicitly assigning importance values to flows via graph attention layers. Compared to recently developed techniques on a large dataset with 101 popular apps using the Android platform, the proposed method improved by 4-20% for accuracy, precision, recall, and F1 score, and spent much less time training.
更多查看译文
关键词
graph attention network,mobile,application-classifying
AI 理解论文
溯源树
样例
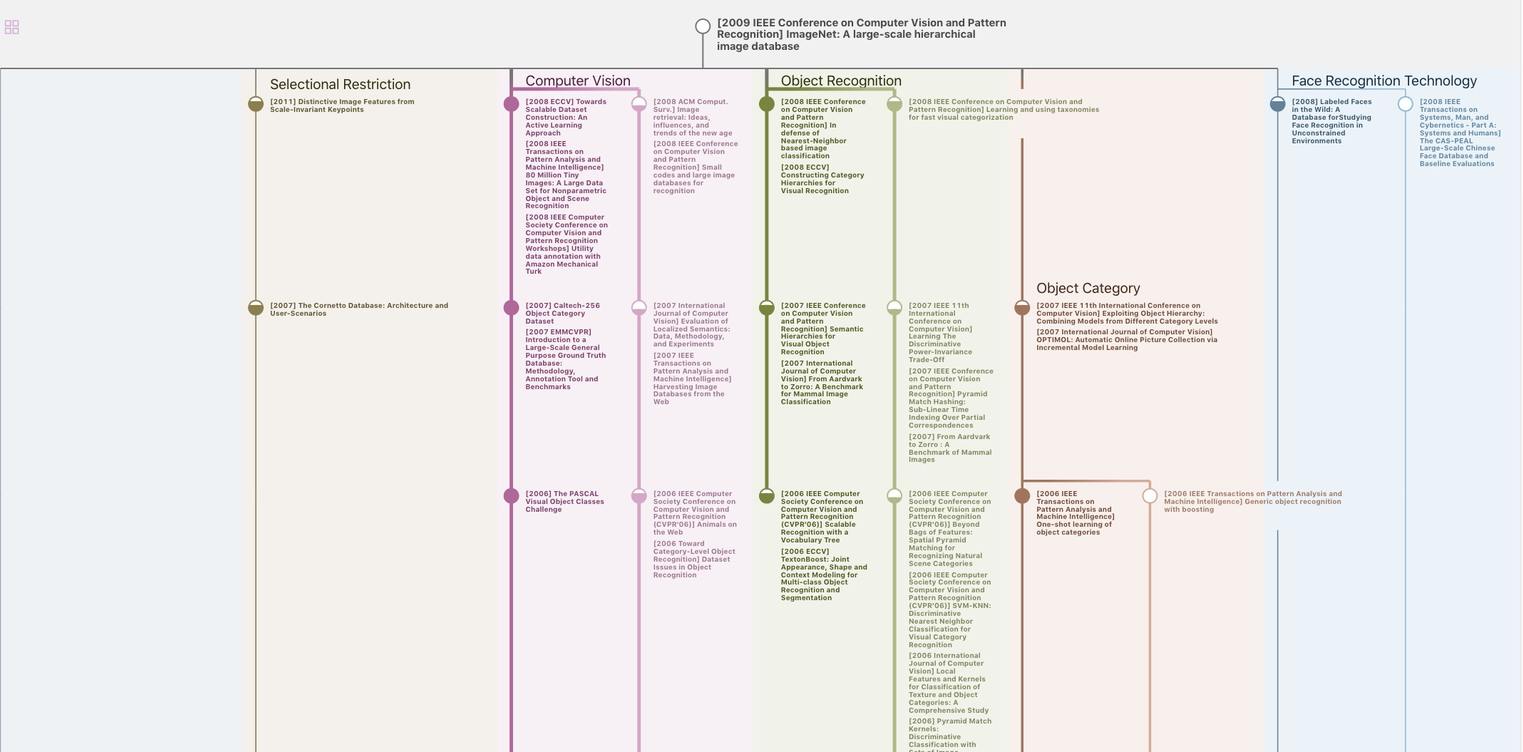
生成溯源树,研究论文发展脉络
Chat Paper
正在生成论文摘要