Detection of clinically significant prostate cancer on mri: a comparison of an artificial intelligence model versus radiologists
JOURNAL OF UROLOGY(2023)
摘要
You have accessJournal of UrologyCME1 Apr 2023MP09-01 DETECTION OF CLINICALLY SIGNIFICANT PROSTATE CANCER ON MRI: A COMPARISON OF AN ARTIFICIAL INTELLIGENCE MODEL VERSUS RADIOLOGISTS Simon John Christoph Soerensen, Richard E. Fan, Indrani Bhattacharya, David S. Lim, Sarir Ahmadi, Xinran Li, Sulaiman Vesal, Mirabela Rusu, and Geoffrey A. Sonn Simon John Christoph SoerensenSimon John Christoph Soerensen More articles by this author , Richard E. FanRichard E. Fan More articles by this author , Indrani BhattacharyaIndrani Bhattacharya More articles by this author , David S. LimDavid S. Lim More articles by this author , Sarir AhmadiSarir Ahmadi More articles by this author , Xinran LiXinran Li More articles by this author , Sulaiman VesalSulaiman Vesal More articles by this author , Mirabela RusuMirabela Rusu More articles by this author , and Geoffrey A. SonnGeoffrey A. Sonn More articles by this author View All Author Informationhttps://doi.org/10.1097/JU.0000000000003224.01AboutPDF ToolsAdd to favoritesDownload CitationsTrack CitationsPermissionsReprints ShareFacebookLinked InTwitterEmail Abstract INTRODUCTION AND OBJECTIVE: Automated detection of prostate cancer on magnetic resonance imaging (MRI) may help target biopsies and avoid unnecessary biopsies of false-positive lesions. We sought to develop an artificial intelligence (AI) model to rapidly and accurately identify cancer foci on MRI and compare its performance against radiologists. METHODS: A total of 1504 subjects underwent multiparametric MRI at more than 30 institutions in the US, followed by MRI-ultrasound fusion biopsy at one institution. We used T2-weighted images (T2WI), apparent diffusion coefficient maps (ADC), and diffusion-weighted imaging (DWI) from 1404 cases to train an AI model. MRI lesions were initially identified and outlined by a radiologist using T2WIs, DWIs, ADC, and contrast enhanced images (DCE). Cancers were confirmed by biopsy. Only lesions containing Gleason 3+3 (≥ 40% cancer core length) or Gleason ≥3+4 (any amount) in at least one targeted biopsy core were considered for training and testing the model. All other MRI lesions were removed. Systematic cores with significant cancer were also included for model training. We tested the AI model on 100 independent cases and compared it against trained radiologists. We computed lesion-level AUC, sensitivity, specificity, and negative predictive value (NPV). RESULTS: AI and radiologists had similar performances across all metrics, and all differences were non-significant. The AI model had an AUC of 0.79±0.22, NPV of 0.66±0.26, sensitivity of 0.34±0.41, and specificity of 0.99±0.05. Radiologists had an AUC of 0.81±0.22, NPV of 0.69±0.28, sensitivity of 0.37±0.45, and specificity of 0.99±0.05. CONCLUSIONS: In this study, we trained and tested an AI model on a large and diverse multi-institutional cohort of MRIs. The model matched the performance of radiologist experts. In future work, we envision comparing model performance against radiologists at different skill levels and comparing radiologist + AI vs radiologist performance alone. Source of Funding: Departments of Radiology and Urology, Stanford University, National Cancer Institute of the National Institutes of Health (R37CA260346 to M.R), and the generous philanthropic support of our patients (G.S.). The content is solely the responsibility of the authors and does not necessarily represent the official views of the National Institutes of Health © 2023 by American Urological Association Education and Research, Inc.FiguresReferencesRelatedDetails Volume 209Issue Supplement 4April 2023Page: e103 Advertisement Copyright & Permissions© 2023 by American Urological Association Education and Research, Inc.MetricsAuthor Information Simon John Christoph Soerensen More articles by this author Richard E. Fan More articles by this author Indrani Bhattacharya More articles by this author David S. Lim More articles by this author Sarir Ahmadi More articles by this author Xinran Li More articles by this author Sulaiman Vesal More articles by this author Mirabela Rusu More articles by this author Geoffrey A. Sonn More articles by this author Expand All Advertisement PDF downloadLoading ...
更多查看译文
关键词
prostate cancer,mri,artificial intelligence model,artificial intelligence
AI 理解论文
溯源树
样例
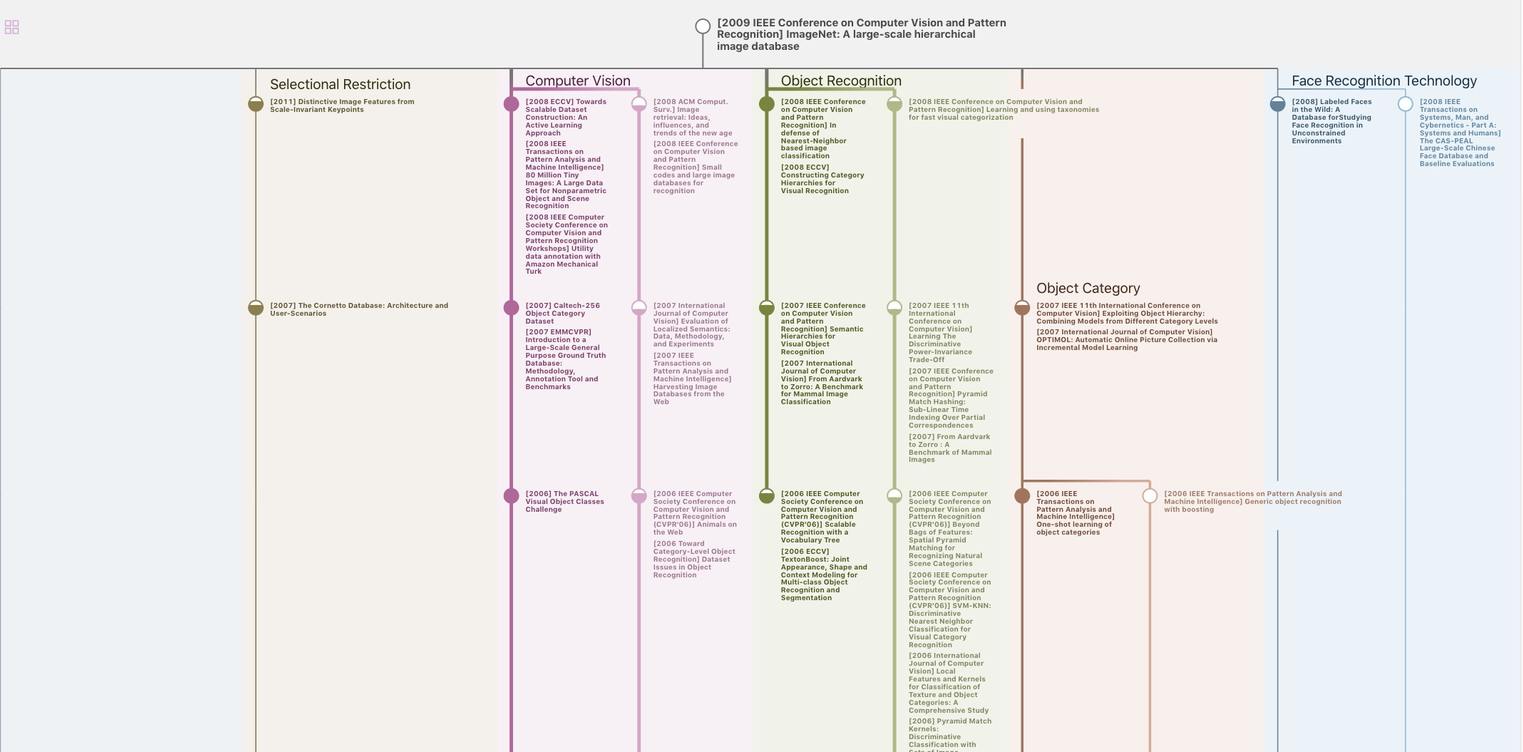
生成溯源树,研究论文发展脉络
Chat Paper
正在生成论文摘要