Adaptive Measurement Covariance Poisson Multi-Bernoulli Mixture Filter for Multi-target Tracking Under Glint Noise
INTERNATIONAL JOURNAL OF CONTROL AUTOMATION AND SYSTEMS(2023)
摘要
In this paper, we present a novel adaptive measurement covariance Poisson multi-Bernoulli mixture (PMBM) filter and develop its implementation to perform multi-target tracking (MTT) under glint noise. To accommodate the heavy-tailed feature of glint noise, we increase the measurement covariance adaptively in the iteration and construct the filter by applying the variational Bayesian (VB) method to the standard PMBM filter. The developed implementation uses a mixture of Gaussian-inverse Gamma terms to approximate the posterior density then recursive estimates the target state and the time-varying measurement covariance. Simulation results illustrate that the proposed AMC-PMBM filter can effectively estimate the multi-target state in the presence of glint noise and has a better performance than the other available filters in linear, nonlinear and real data cases.
更多查看译文
关键词
Gaussian-inverse Gamma distribution,glint noise,Poisson multi-Bernoulli mixture,variational Bayesian
AI 理解论文
溯源树
样例
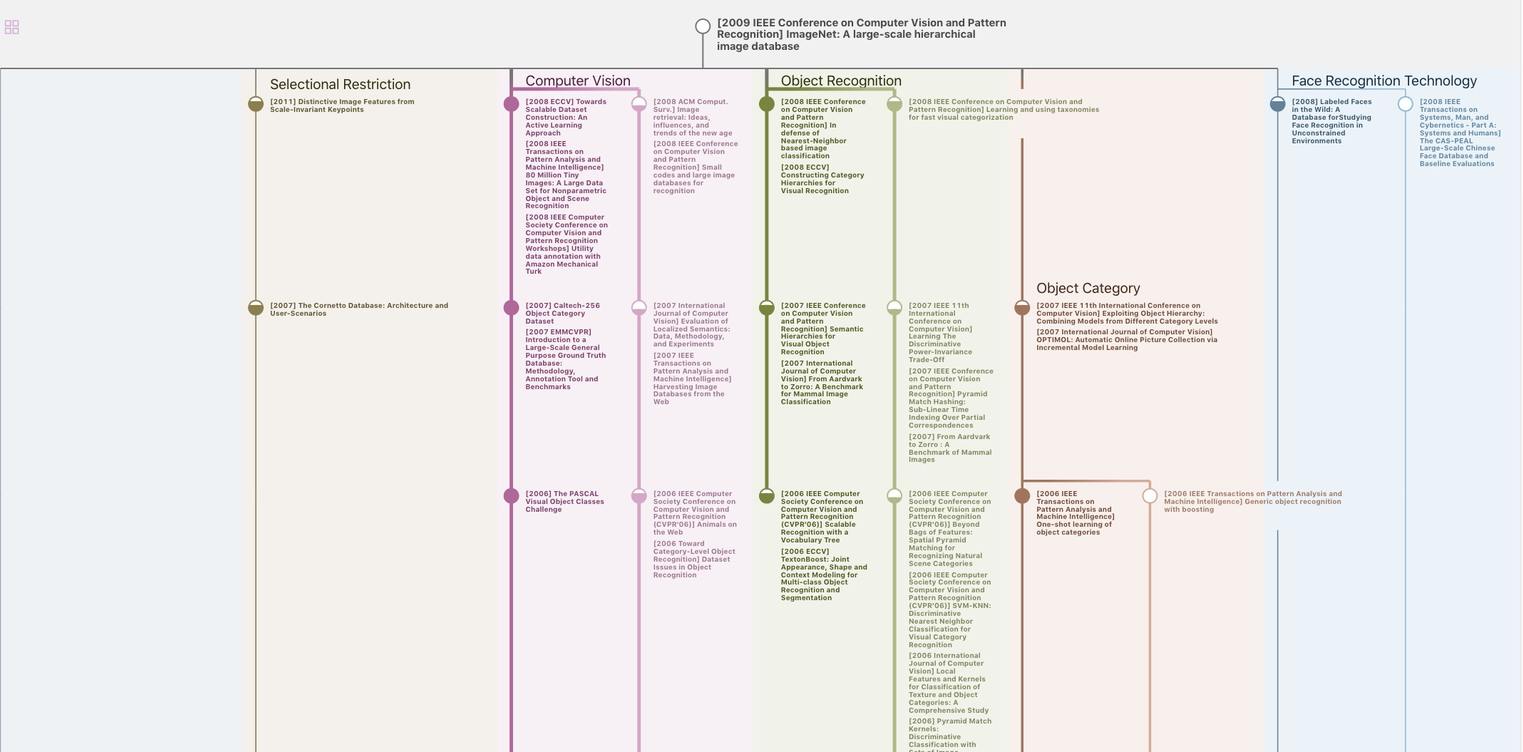
生成溯源树,研究论文发展脉络
Chat Paper
正在生成论文摘要