A prediction model for thermal conductivity of metallic nuclear fuel based on multiple machine learning models
JOURNAL OF NUCLEAR MATERIALS(2023)
摘要
In this work, the presence of the BCC phase and its thermal conductivity in uranium-based metallic nuclear fuels at higher temperatures were predicted by coupling a random forest classification model with a multilayer per-ceptron network model. To analyze the effect of phase constitution on thermal conductivity in uranium-based alloys, the pearson correlation coefficient was introduced. The data samples in the current small-sample data-base of metallic nuclear fuels, created by collecting experimental and computational data from the literature, were first pre-processed with the guide of professional knowledge before machine learning, involving data screening and data completion. Based on temperature and composition characteristics, the existence of the BCC phases and the thermal conductivities of the candidates were predicted by our models. The optimized thermal conductivities of the candidate alloys coincide well with the experimental results, which shows the reliability of our model.
更多查看译文
关键词
thermal conductivity,metallic nuclear fuel,machine learning models,prediction model,machine learning
AI 理解论文
溯源树
样例
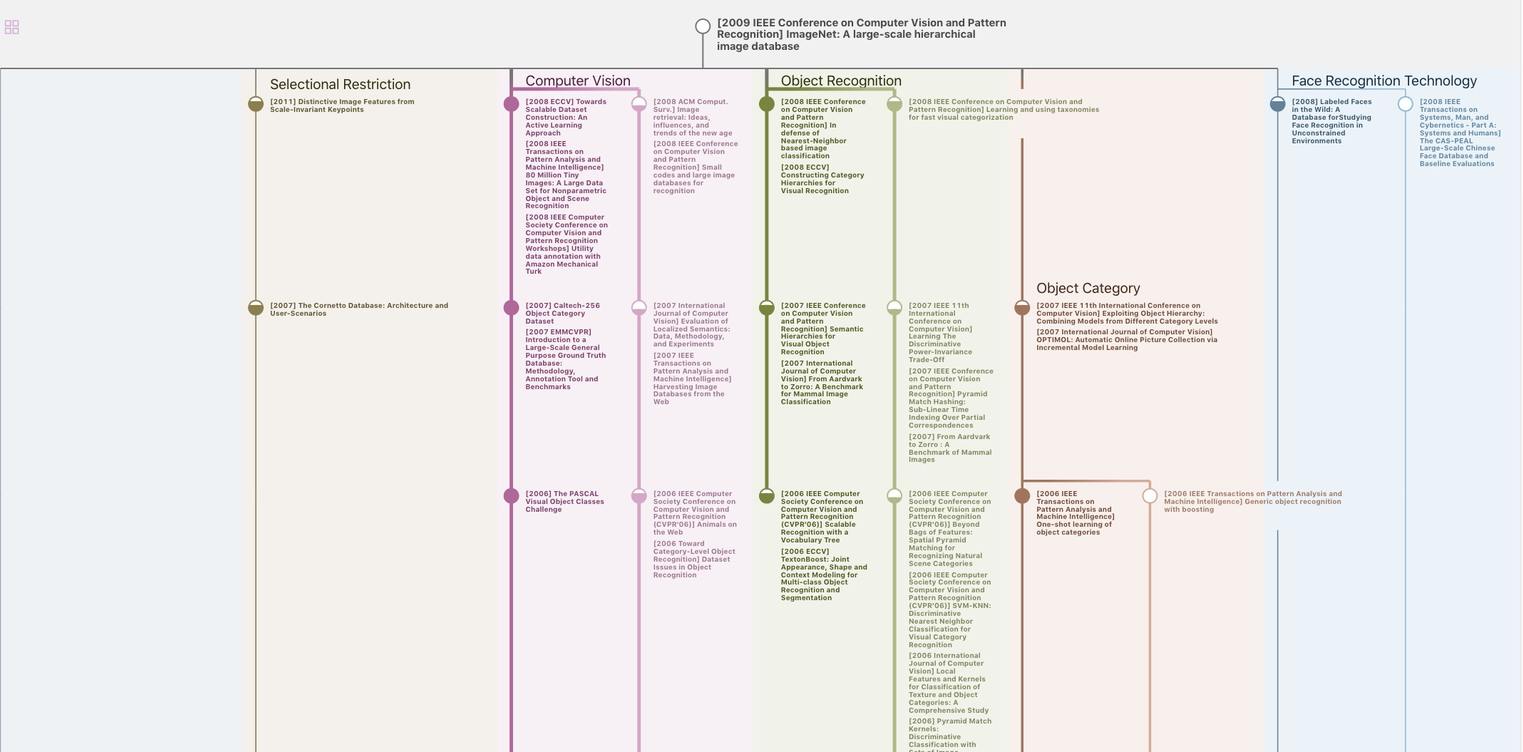
生成溯源树,研究论文发展脉络
Chat Paper
正在生成论文摘要