Research on Edge Diagnosis Method of UHVDC Commutation Failure Based on LSTM Neural Network
2022 4TH INTERNATIONAL CONFERENCE ON SMART POWER & INTERNET ENERGY SYSTEMS, SPIES(2022)
摘要
Commutation failure is one of the most common faults in UHVDC stations, which seriously endangers power transmission and safe operation. Therefore, this paper proposes a commutation failure diagnosis method based on wavelet energy entropy and long short-term memory (LSTM) neural network to diagnose and trace fault recording data at UHVDC station. Firstly, a +/- 1100kV UHVDC simulation model is established in this paper. In the case of commutation failure and short circuit fault, the model is compared with the recorded data to improve the accuracy of the model and provide sufficient data basis for LSTM training. By analyzing the accumulated commutation failure condition data in the field, the simulation model can be set to obtain a more suitable fault training data set for the actual UHVDC system, and improve the training accuracy of LSTM neural network. Then 11 field recording data were selected for wavelet energy entropy calculation to extract fault features and construct LSTM neural network fault algorithm, which was deployed in the field fault recording server to complete diagnostic function verification and accuracy test. The test results show that the proposed method can achieve 100% simulation diagnosis accuracy and 86% field diagnosis accuracy in tracing commutation failure.
更多查看译文
关键词
Commutation failure,Fault diagnosis,Long and short-term memory Neural network (LSTM-NN),Wavelet energy entropy
AI 理解论文
溯源树
样例
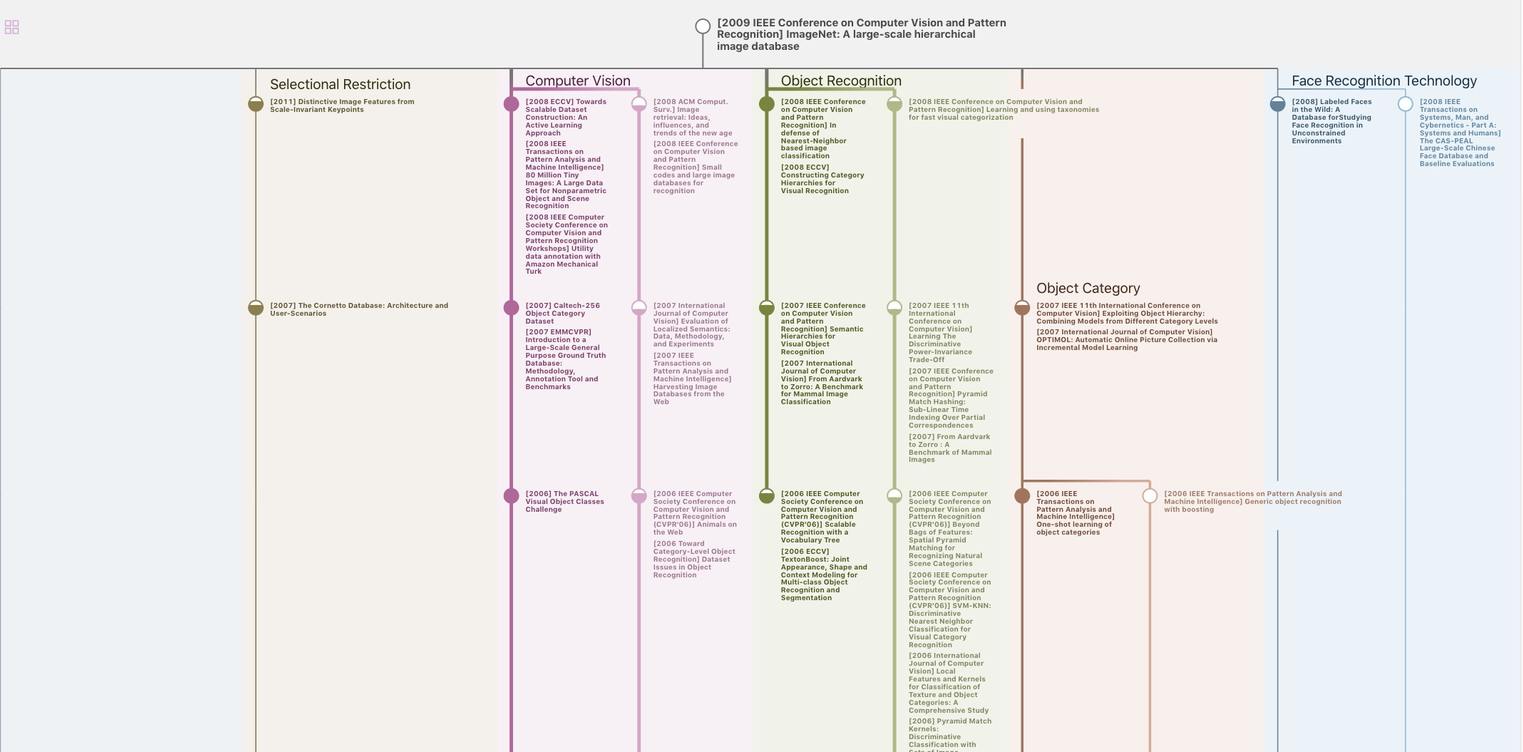
生成溯源树,研究论文发展脉络
Chat Paper
正在生成论文摘要