Learning to Topology Derivation of Power Electronics Converters with Graph Neural Network
2022 4TH INTERNATIONAL CONFERENCE ON SMART POWER & INTERNET ENERGY SYSTEMS, SPIES(2022)
摘要
This paper proposes a general learning framework to derive topology of power electronics converters. To increase flexibility, a circuit is represented by a graph. A Graph Neural Network extract features of the circuit graph, which is further used in the RL framework. The topology derivation process is regarded as a Markov Decision Process. In each step, the RL agent selects and connects a new block to the initial block until a complete topology is made. To ensure that the derived circuits are feasible, basic circuit constraints are taken into consideration in the reward function. By using this framework, many new six-port, eight-port and ten-port converters are derived. Simulation results show that the derived circuits satisfy given constraints well.
更多查看译文
关键词
multiport DC–DC converters,topology derivation,Graph Neural Network (GNN),Reinforcement Learning (RL)
AI 理解论文
溯源树
样例
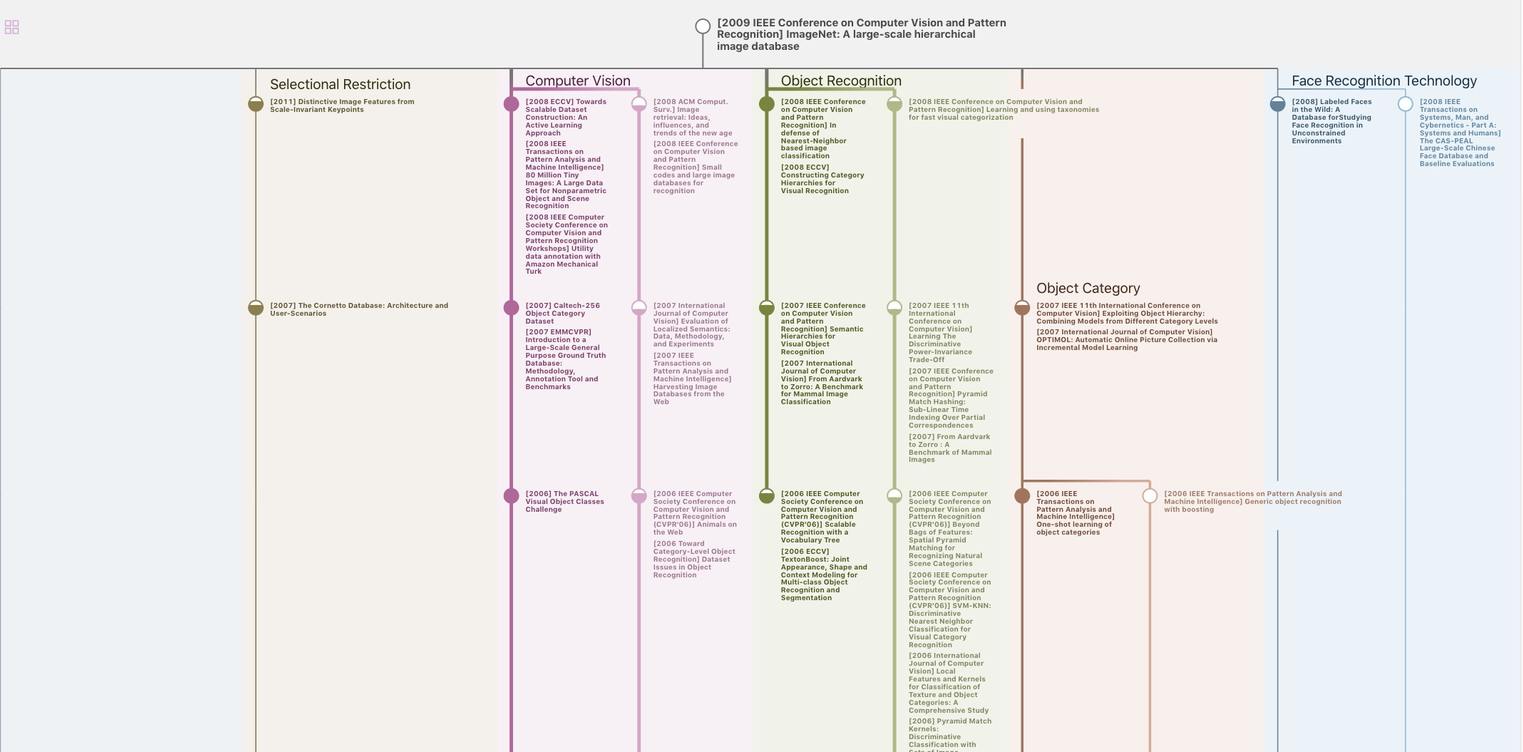
生成溯源树,研究论文发展脉络
Chat Paper
正在生成论文摘要