In silico design of copper-based alloys for ammonia synthesis from nitric oxide reduction accelerated by machine learning
JOURNAL OF MATERIALS CHEMISTRY A(2023)
摘要
The NO electroreduction reaction (NORR) has been recognized as a promising strategy for NO removal and NH3 synthesis, while current NORR electrocatalysts suffer from limited activity and selectivity. Here, we comprehensively investigate the NORR performance of copper alloys by virtue of first-principles calculations and machine learning (ML). It is identified that the adsorption energy of N atoms E-ads(*N) is an effective catalytic descriptor for the NORR. As a result of screening 140 copper alloys, we discover Cu@Cu3Ni and Cu2Ni2@Cu3Ni with extremely low limiting potentials and reasonably low kinetic barriers. Then, we construct a highly accurate ML model for predicting the E-ads(*N) and clarify the local elemental features as critical factors. By predicting the E-ads(*N) on similar to 2 000 000 bimetallic alloy surfaces, we reveal that Ni is the optimal alloy non-noble-metal element to enhance the NORR activity. Our work not only opens a new avenue for the design of efficient alloy catalysts but also paves the way toward the ML-accelerated discovery of novel NORR catalysts.
更多查看译文
AI 理解论文
溯源树
样例
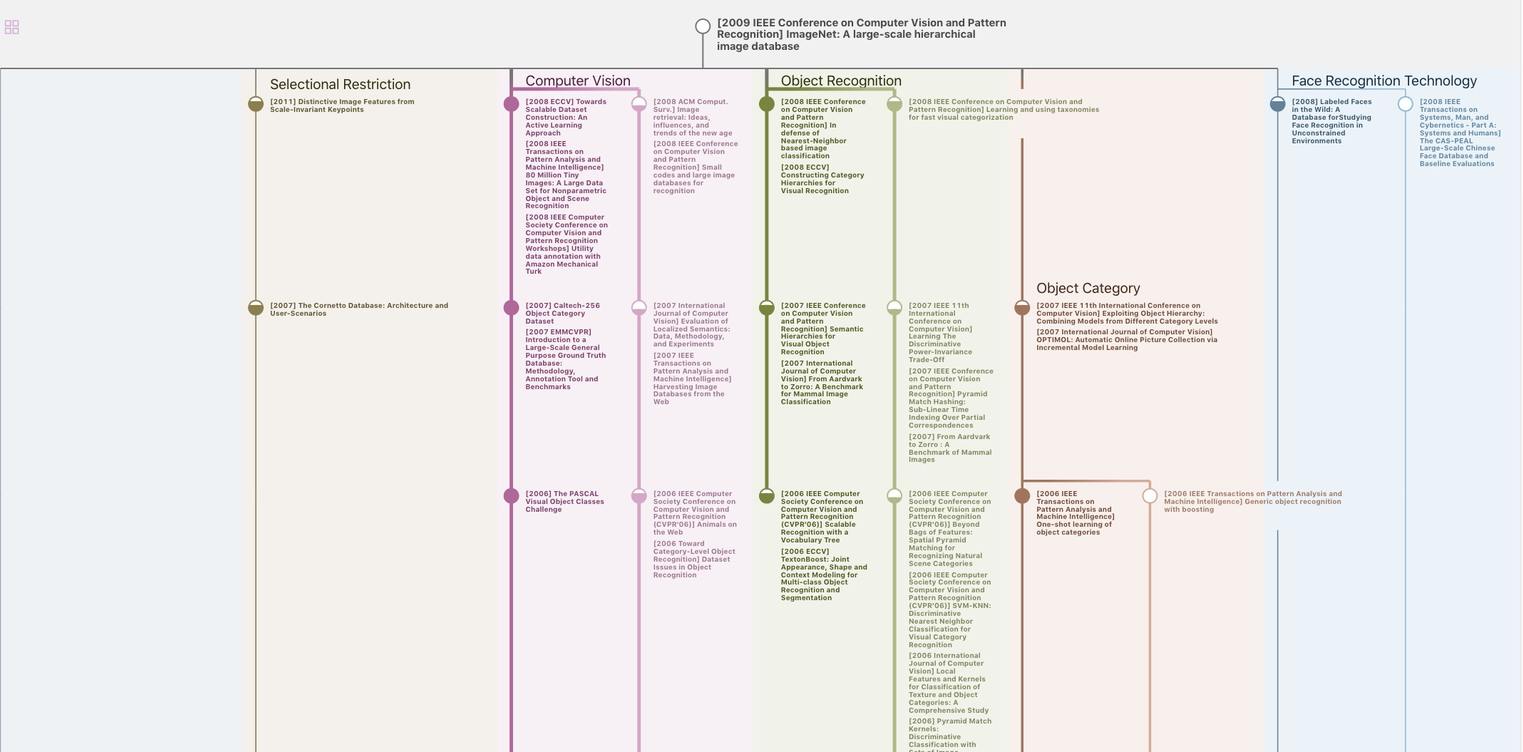
生成溯源树,研究论文发展脉络
Chat Paper
正在生成论文摘要