Early fault diagnosis in rolling element bearings: comparative analysis of a knowledge-based and a data-driven approach
JOURNAL OF INTELLIGENT MANUFACTURING(2023)
摘要
The early identification of a defect that is developing in a bearing is crucial for avoiding failures in rotating machinery. Frequency domain analysis of the vibration signals has been shown to contribute to a better understanding of the nature of a developing defect. Early signs of degradation might be more noticeable in certain frequency bands. The advantages in identifying and monitoring these bandwidths are several: prevention of serious machinery damages, reduction of the loss of investments, and improvement of the accuracy in failure predicting models. This paper presents and compares two approaches for the diagnosis of bearing faults. The first approach was knowledge-based. It relied on principles of mechanics to interpret the measured vibration signals and utilized prior knowledge of the bearing characteristics and testing parameters. The second approach was data-driven whereby data were acquired exclusively from the vibration signal. Both approaches were successfully applied for fault diagnosis by identifying the frequencies of the vibration spectra characteristic for the bearing under study. From this, bandwidths of interest for early fault detection could be determined. The diagnostic abilities of both approaches were studied to analyze and compare their individual strengths regarding the aspects of implementation time, domain knowledge, data processing associated knowledge, data requirements, diagnostic performance, and practical applicability. The advantages, apparent limitations as well as avenues for further improvement of both approaches are discussed.
更多查看译文
关键词
Fault diagnosis,Data-driven,Knowledge-based,Rolling elements bearings,Vibration,Degradation
AI 理解论文
溯源树
样例
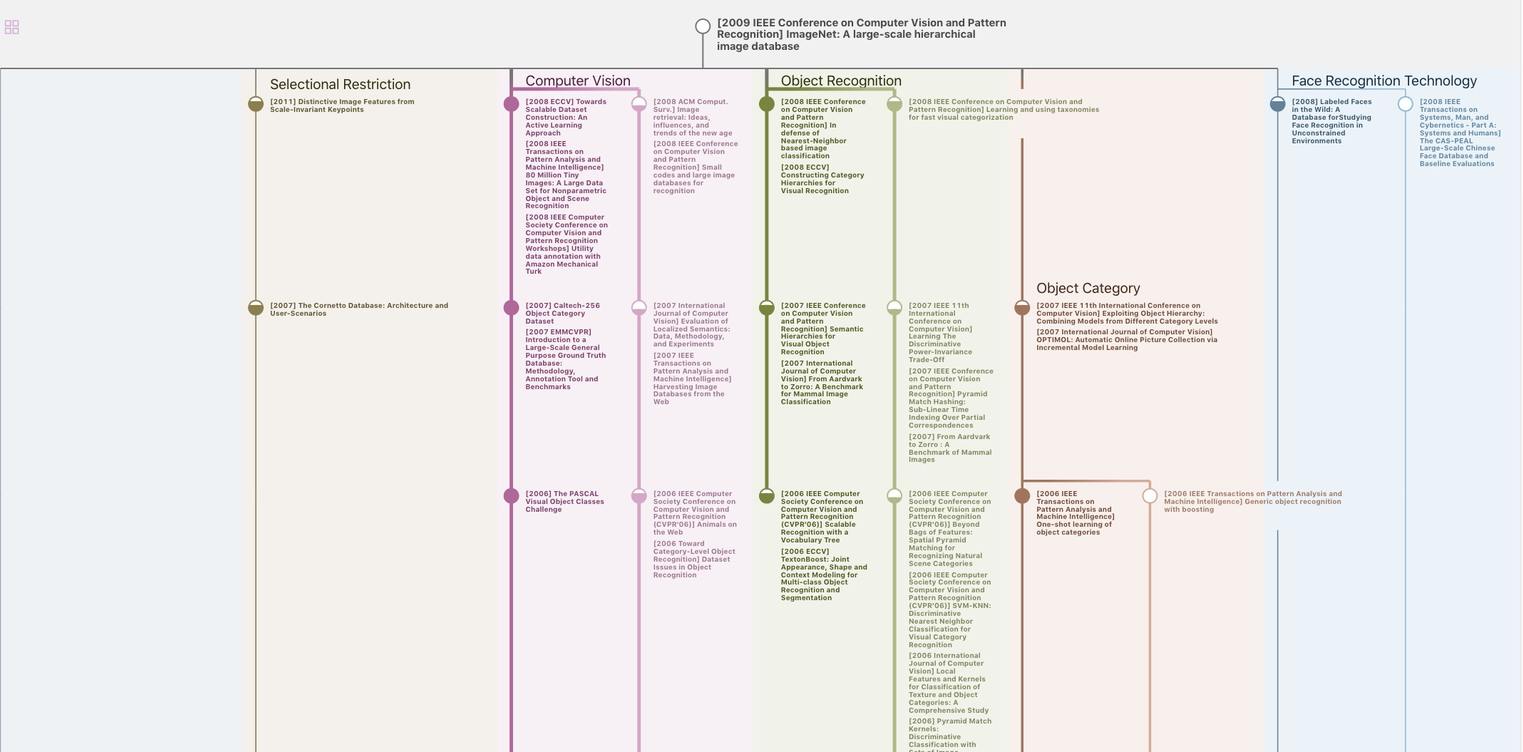
生成溯源树,研究论文发展脉络
Chat Paper
正在生成论文摘要