A novel split selection of a logistic regression tree for the classification of data with heterogeneous subgroups
INTERNATIONAL JOURNAL OF INDUSTRIAL ENGINEERING-THEORY APPLICATIONS AND PRACTICE(2023)
摘要
A logistic regression tree (LRT) is a hybrid machine learning method that combines a decision tree model and logistic regression models. An LRT recursively partitions the input data space through splitting and learns multiple logistic regression models optimized for each subpopulation. The split selection is a critical procedure for improving the predictive performance of the LRT. In this paper, we present a novel separability-based split selection method for the construction of an LRT. The separability measure, defined on the feature space of logistic regression models, evaluates the performance of potential child models without fitting, and the optimal split is selected based on the results. Heterogeneous subgroups that have different class-separating patterns can be identified in the split process when they exist in the data. In addition, we compare the performance of our proposed method with the benchmark algorithms through experiments on both synthetic and real-world datasets. The experimental results indicate the effectiveness and generality of our proposed method.
更多查看译文
关键词
Model Tree, Logistic Regression Tree, Subgroup Identification, Class Separability
AI 理解论文
溯源树
样例
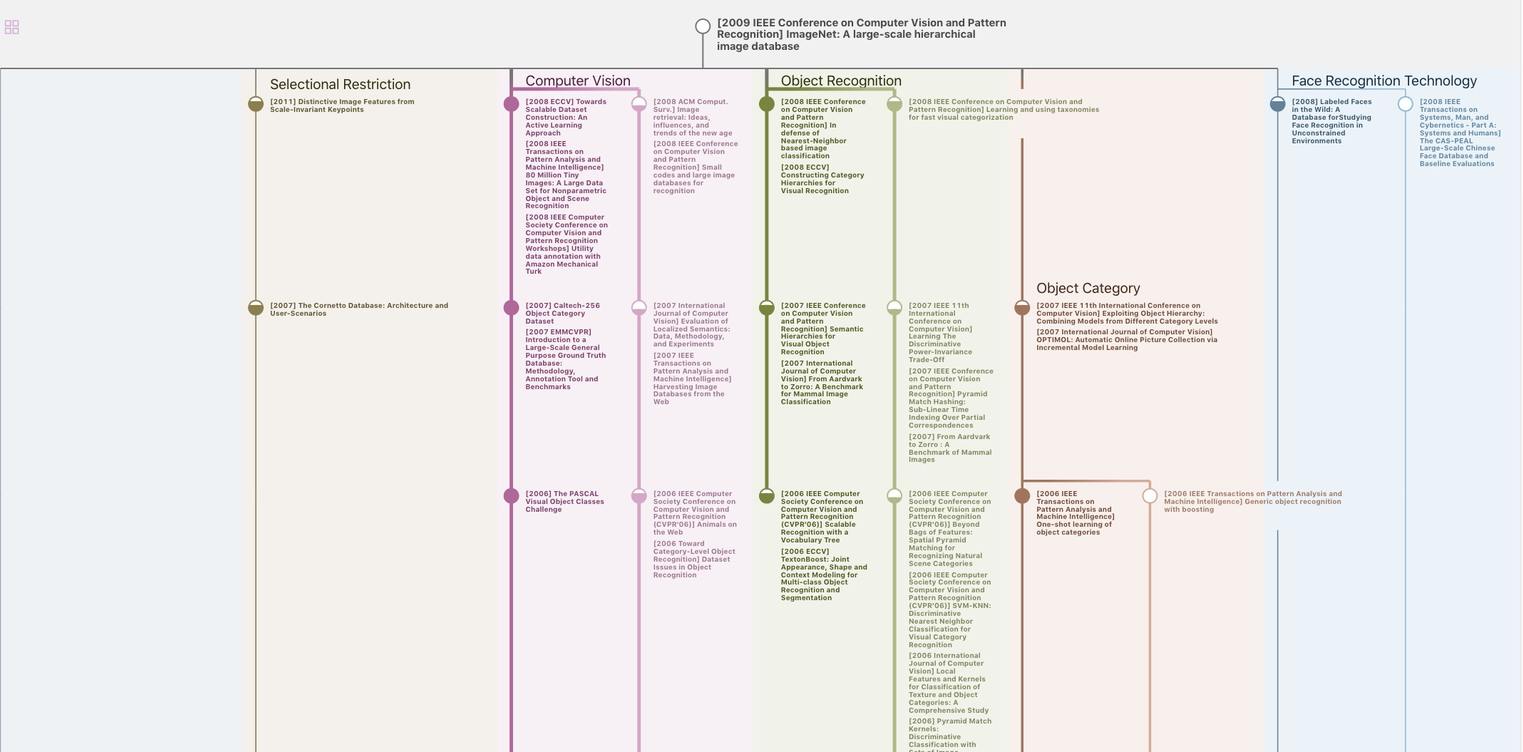
生成溯源树,研究论文发展脉络
Chat Paper
正在生成论文摘要