Canonical Correlation Analysis based Bi-Graph Convolutional Network for Stock Price Movement Prediction
2022 INTERNATIONAL CONFERENCE ON IMAGE PROCESSING, COMPUTER VISION AND MACHINE LEARNING (ICICML)(2022)
摘要
Stock price movement prediction is a very challenging task due to the high volatility and complexity of uncertain financial market. Obviously, the price fluctuations of a target stock is inevitably affected by the price of other related stocks. However, the cross interaction effect among a collection of stocks is not fully explored in the literature. In this paper, we develop a deep learning based novel framework, which combines graph convolutional network, gated recurrent unit and canonical correlation analysis to perform stock movement prediction. Specifically, multiple relationships among stocks are represented by two graphs, namely, industry graph and topicality graph, to model the cross effect problem. Furthermore, gated recurrent unit is utilized to detect the temporal relationships among stocks. In addition, the canonical correlation analysis method is employed to enhance the inter-view correlation between industry factor and topicality factor. Experiments on the well known China Securities Index 300 demonstrate the performance and effectiveness of the proposed approach.
更多查看译文
关键词
canonical correlation analysis, gated recurrent unit, graph convolutional network
AI 理解论文
溯源树
样例
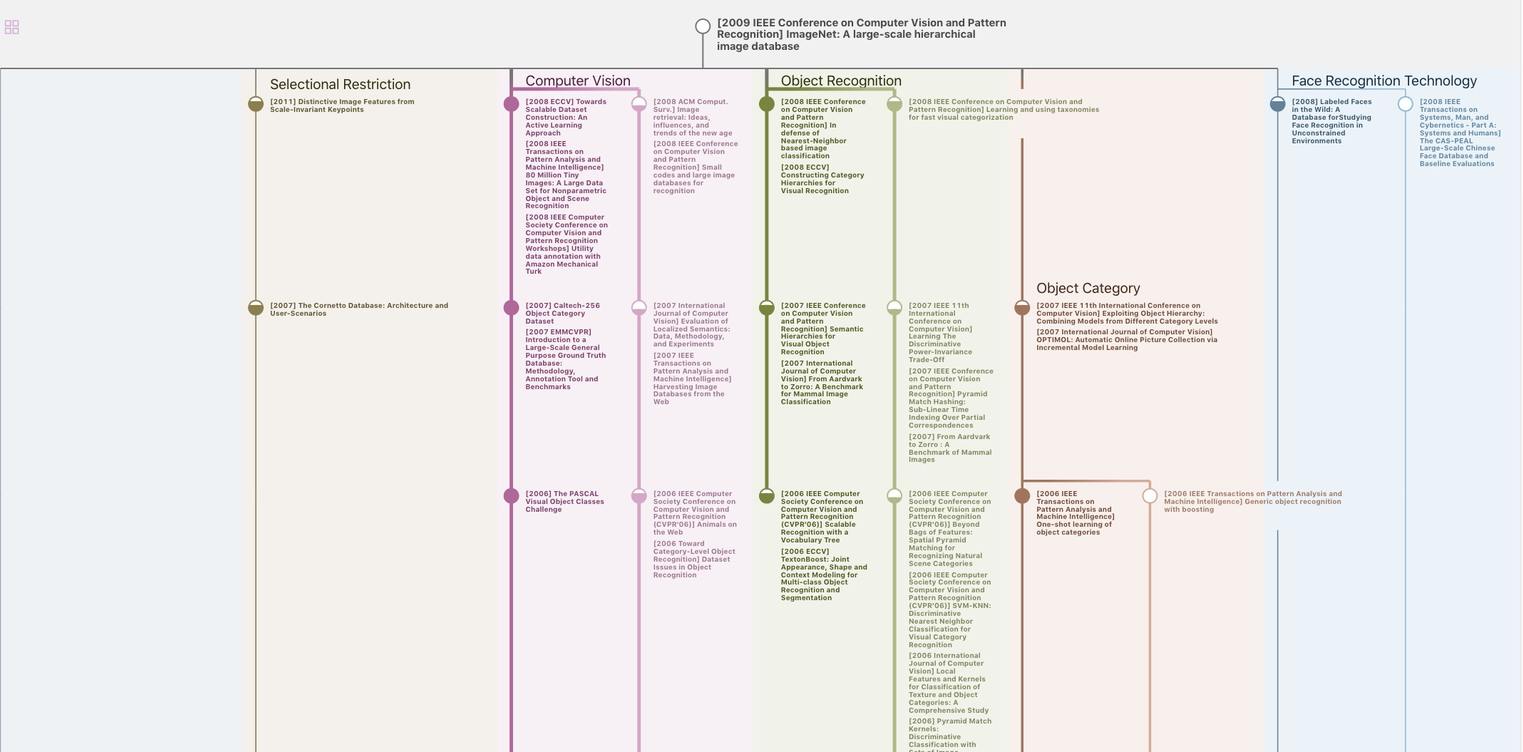
生成溯源树,研究论文发展脉络
Chat Paper
正在生成论文摘要