Style and content separation network for remote sensing image cross-scene generalization
ISPRS JOURNAL OF PHOTOGRAMMETRY AND REMOTE SENSING(2023)
摘要
Domain shift is the problem in which trained models fail to maintain their performance when they confront new test domains. Cross-scene classification, a technique developed to overcome this challenge, has attracted significant research interest in the field of remote sensing (RS). By exploring the correlation between the source domain and the target domain, relevant models can have better generalization ability regarding the target domain. Nevertheless, Domain Adaptation (DA), as the main technique for cross-scene classification, necessarily requires access to the target samples to assist the model training, a condition that is difficult to satisfy in real-world applications. Domain Generalization (DG) has attracted increasing research attention in recent years. Given one or several source domain(s), DG tends to learn models that can perform well when dealing with unseen (inaccessible) target domains. DG can better deal with out-of-distribution generalization with fewer restrictions, making it a good fit for cross-scene classification. Notably, little research has been conducted on domain generalization in the field of remote sensing. Thus, we refer to this type of method as cross-scene generalization. Recent studies have shown that convolutional neural networks have a strong bias towards recognizing textures rather than shapes. Accordingly, we proposed a Style and Content Separation Network (SCSN) for RS image cross-scene generalization in this paper, which can improve the generalizability and discriminative capability. The Style and Content Separation (SCS) module uses instance normalization to obtain the content information and thereby ensure better generalization ability. Moreover, the residual feature, which contains the style information, can supplement the feature representations after refinement. We further proposed a separation loss to constrain the style and content separation process. Experimental results and relevant analysis demonstrate the effectiveness of the proposed SCSN on cross-scene generalization tasks. Code is available at https://github.com/WHUzhusihan96/SCSN.
更多查看译文
关键词
content separation network,remote sensing,cross-scene
AI 理解论文
溯源树
样例
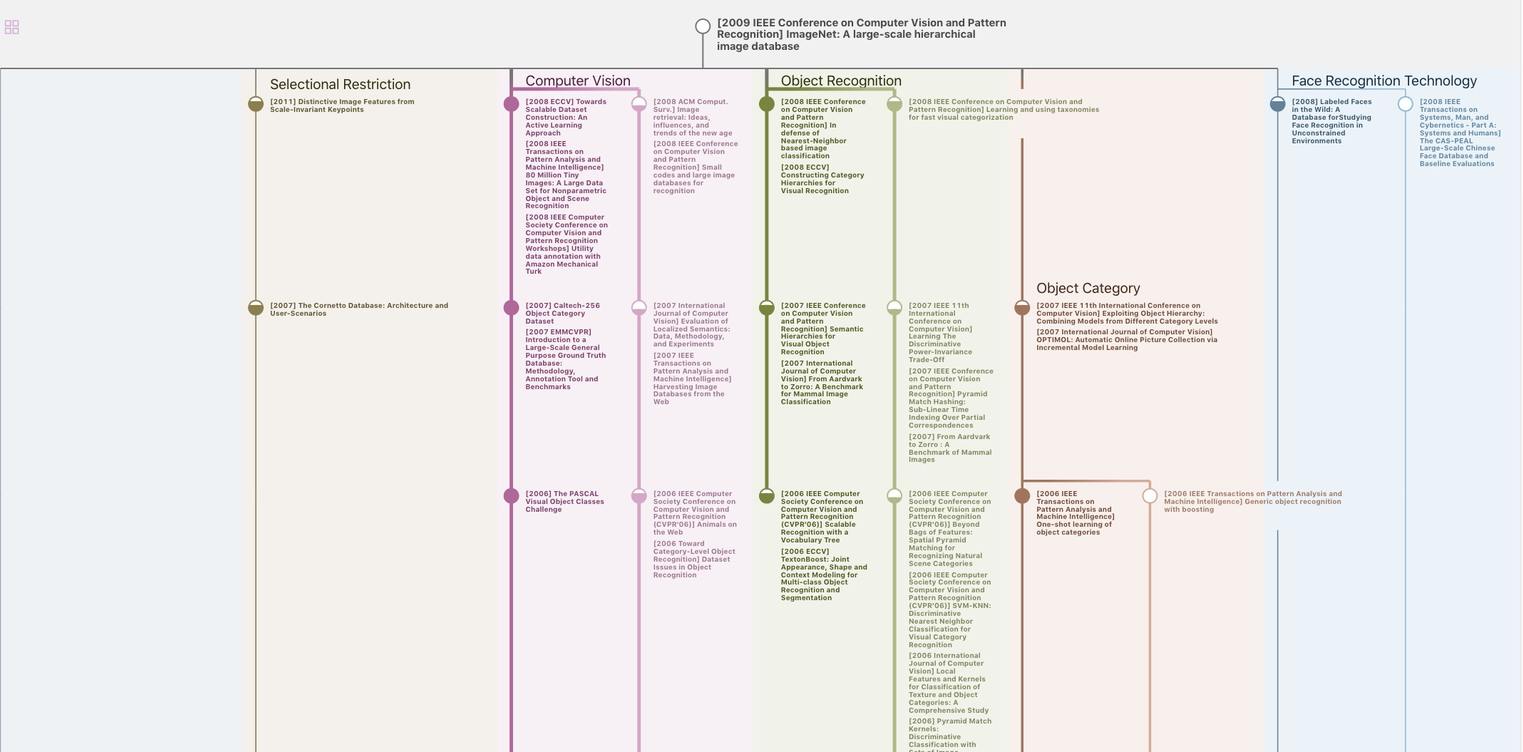
生成溯源树,研究论文发展脉络
Chat Paper
正在生成论文摘要