Dynamic adaptive spatio-temporal graph network for COVID-19 forecasting
CAAI TRANSACTIONS ON INTELLIGENCE TECHNOLOGY(2023)
摘要
Appropriately characterising the mixed space-time relations of the contagion process caused by hybrid space and time factors remains the primary challenge in COVID-19 forecasting. However, in previous deep learning models for epidemic forecasting, spatial and temporal variations are captured separately. A unified model is developed to cover all spatio-temporal relations. However, this measure is insufficient for modelling the complex spatio-temporal relations of infectious disease transmission. A dynamic adaptive spatio-temporal graph network (DASTGN) is proposed based on attention mechanisms to improve prediction accuracy. In DASTGN, complex spatio-temporal relations are depicted by adaptively fusing the mixed space-time effects and dynamic space-time dependency structure. This dual-scale model considers the time-specific, space-specific, and direct effects of the propagation process at the fine-grained level. Furthermore, the model characterises impacts from various space-time neighbour blocks under time-varying interventions at the coarse-grained level. The performance comparisons on the three COVID-19 datasets reveal that DASTGN achieves state-of-the-art results with a maximum improvement of 17.092% in the root mean-square error and 11.563% in the mean absolute error. Experimental results indicate that the mechanisms of designing DASTGN can effectively detect some spreading characteristics of COVID-19. The spatio-temporal weight matrices learned in each proposed module reveal diffusion patterns in various scenarios. In conclusion, DASTGN has successfully captured the dynamic spatio-temporal variations of COVID-19, and considering multiple dynamic space-time relationships is essential in epidemic forecasting.
更多查看译文
关键词
adaptive, COVID-19 forecasting, dynamic, intervention, spatio-temporal graph neural networks
AI 理解论文
溯源树
样例
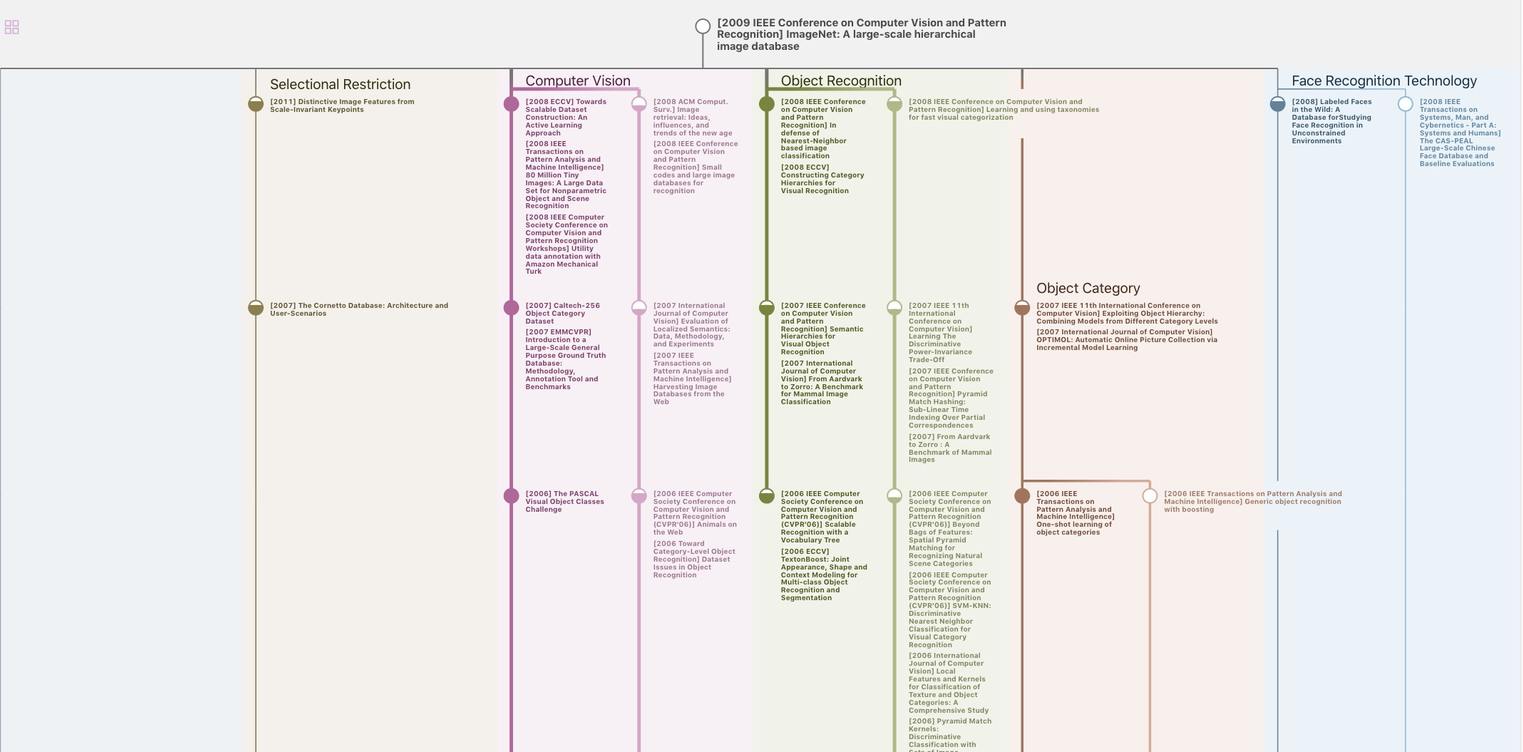
生成溯源树,研究论文发展脉络
Chat Paper
正在生成论文摘要