Learning distribution of video captions using conditional GAN
MULTIMEDIA TOOLS AND APPLICATIONS(2024)
摘要
Automatic video captioning aims to generate captions with textual descriptions to express video content in natural language by a machine. This is a difficult task as the videos contain dynamic challenges. Most of the available approaches for video captioning are often focused on providing a single descriptive sentence. Encoder-decoder is the most popular architecture developed for video captioning. The proposed method in this research aims to learn the distribution of captions to generate more relevant and diverse captions and increase generalizability. A novel architecture was developed based on conditional SeqGAN to learn the distribution for video captioning and increase the generalizability. This architecture consists of two modules: encoding and caption generation. The goal of encoding is to obtain encoded rich spatial-temporal features. The encoding vector is fed as the input of conditional SeqGAN to generate captions. The main novelty of this paper lies in the use of an adversarial approach to learn the distribution of captions and generate diverse captions that fit the characteristics of the video. Experimental results from two popular datasets, MSVD and MSRVTT, showed that the proposed approach achieved more relevant video captions than other state-of-the-art methods.
更多查看译文
关键词
Video captioning,Captions distribution,Conditional GAN,Encoder-decoder,Captions generation,Adversarial Video Captioning
AI 理解论文
溯源树
样例
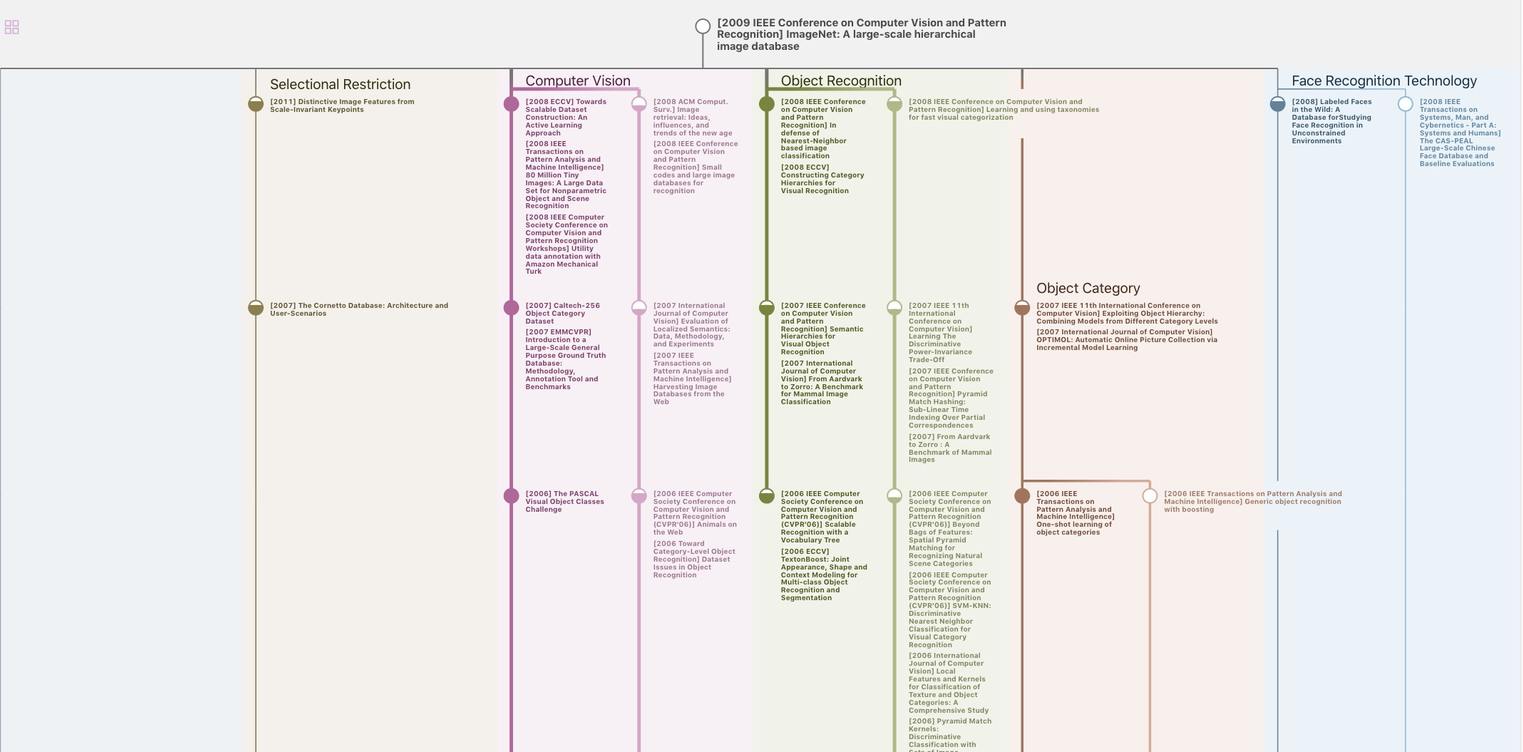
生成溯源树,研究论文发展脉络
Chat Paper
正在生成论文摘要