Performance Evaluation of Deep Dense Layer Neural Network for Diabetes Prediction
CMC-COMPUTERS MATERIALS & CONTINUA(2023)
摘要
Diabetes is one of the fastest-growing human diseases worldwide and poses a significant threat to the population's longer lives. Early prediction of diabetes is crucial to taking precautionary steps to avoid or delay its onset. In this study, we proposed a Deep Dense Layer Neural Network (DDLNN) for diabetes prediction using a dataset with 768 instances and nine variables. We also applied a combination of classical machine learning (ML) algorithms and ensemble learning algorithms for the effective prediction of the disease. The classical ML algorithms used were Support Vector Machine (KNN), and Naive Bayes (NB). We also constructed ensemble models such as bagging (Random Forest) and boosting like AdaBoost and Extreme Gradient Boosting (XGBoost) to evaluate the performance of prediction models. The proposed DDLNN model and ensemble learning models were trained and tested using hyperparameter tuning and K-Fold cross-validation to determine the best parameters for predicting the disease. The combined ML models used majority voting to select the best outcomes among the models. The efficacy of the proposed and other models was evaluated for effective diabetes prediction. The investigation concluded that the proposed model, after hyperparameter tuning, outperformed other learning models with an accuracy of 84.42%, a precision of 85.12%, a recall rate of 65.40%, and a specificity of 94.11%.
更多查看译文
关键词
Diabetes prediction,hyperparameter tuning,k-fold validation,machine learning,neural network
AI 理解论文
溯源树
样例
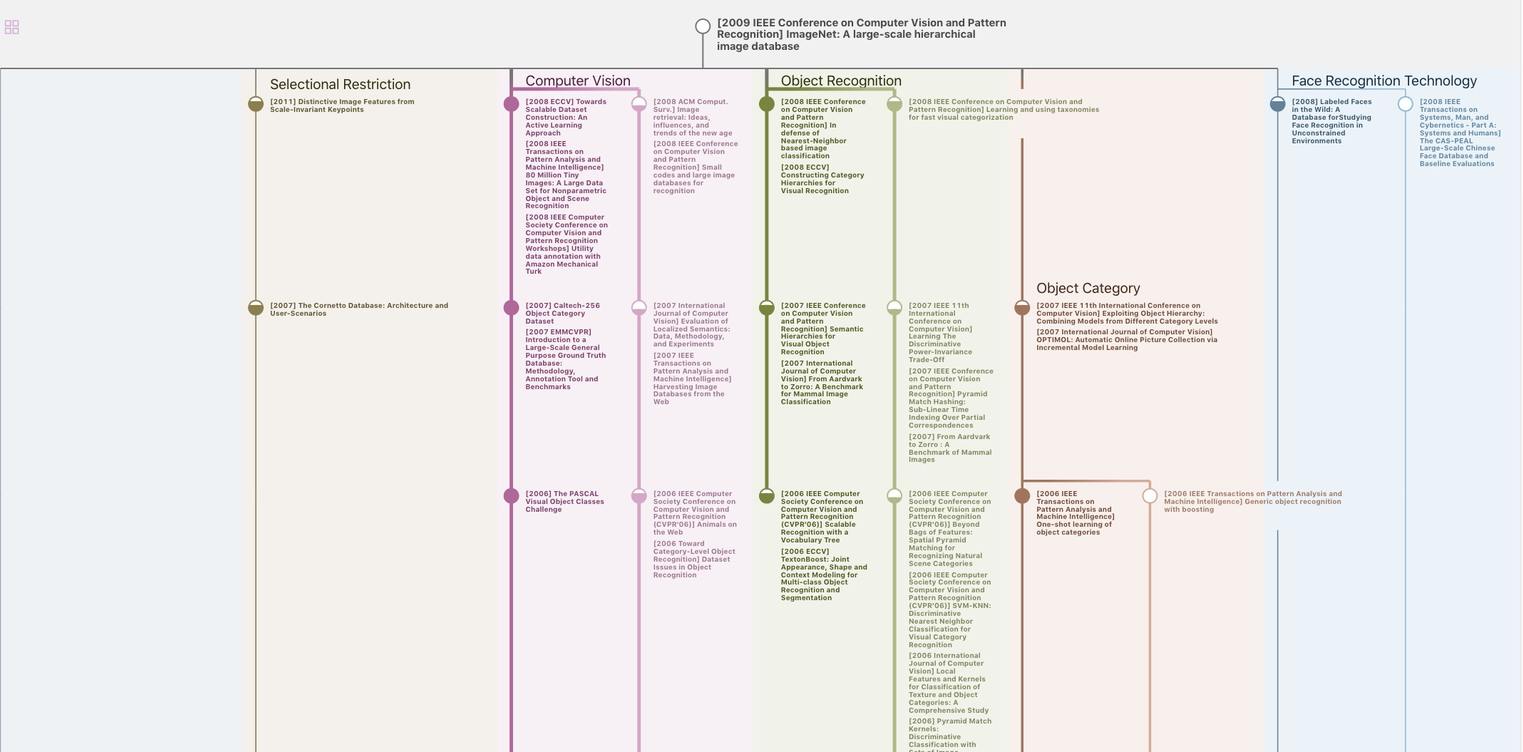
生成溯源树,研究论文发展脉络
Chat Paper
正在生成论文摘要