Automatic Multi-class Sleep Staging Method Based on Novel Hybrid Features
JOURNAL OF ELECTRICAL ENGINEERING & TECHNOLOGY(2024)
摘要
To address the problems of various sleep staging criteria and poor accuracy of sleep staging, we proposed an effective method with novel hybrid features. Besides the common signal of Heart Rate Variability (HRV), we also propose the R Peak (RP) signal which can better reflect the essence of the ECG signal, and then extract the time domain, frequency domain, and nonlinear features from them. The features are processed and selected as novel hybrid features. The best-performing classifier was optimized to evaluate sleep under different criteria. There are four staging criteria, including 2-stages sleep (SW), Sleep and Wake; 3-stages sleep (NRW), Non-rapid Eye Movement (NREM), Rapid Eye Movement (REM), and Wake; 4-stages sleep (DLRW), Deep Sleep, Light Sleep, REM and Wake; and 5-stages sleep (N3RW), N3, N2, N1, REM, and Wake. The experiment was tested on the St. Vincent's University Hospital/University College Dublin Sleep Apnea Database (UCDDB) and validated on the MIT-BIH Polysomnographic Database (MITBPD). The optimized Gradient Boosting Decision Tree (GBDT) was employed as the sleep staging classifier. Compared with other state-of-the-art methods, the proposed method achieved better performance: Under SW, NRW, DLRW, and N3RW, the average accuracy rates of the two databases are 91.34%, 89.56%, 87.15%, and 82.02%, respectively. The average values of Cohen’s Kappa statistics are 0.7518, 0.7742, 0.7718, and 0.7288. The experimental results verified that the proposed method could be used as a versatility, convenience, and reliability method for automatic sleep staging.
更多查看译文
关键词
Novel hybrid features,Sleep staging,R Peak signal,Feature selection
AI 理解论文
溯源树
样例
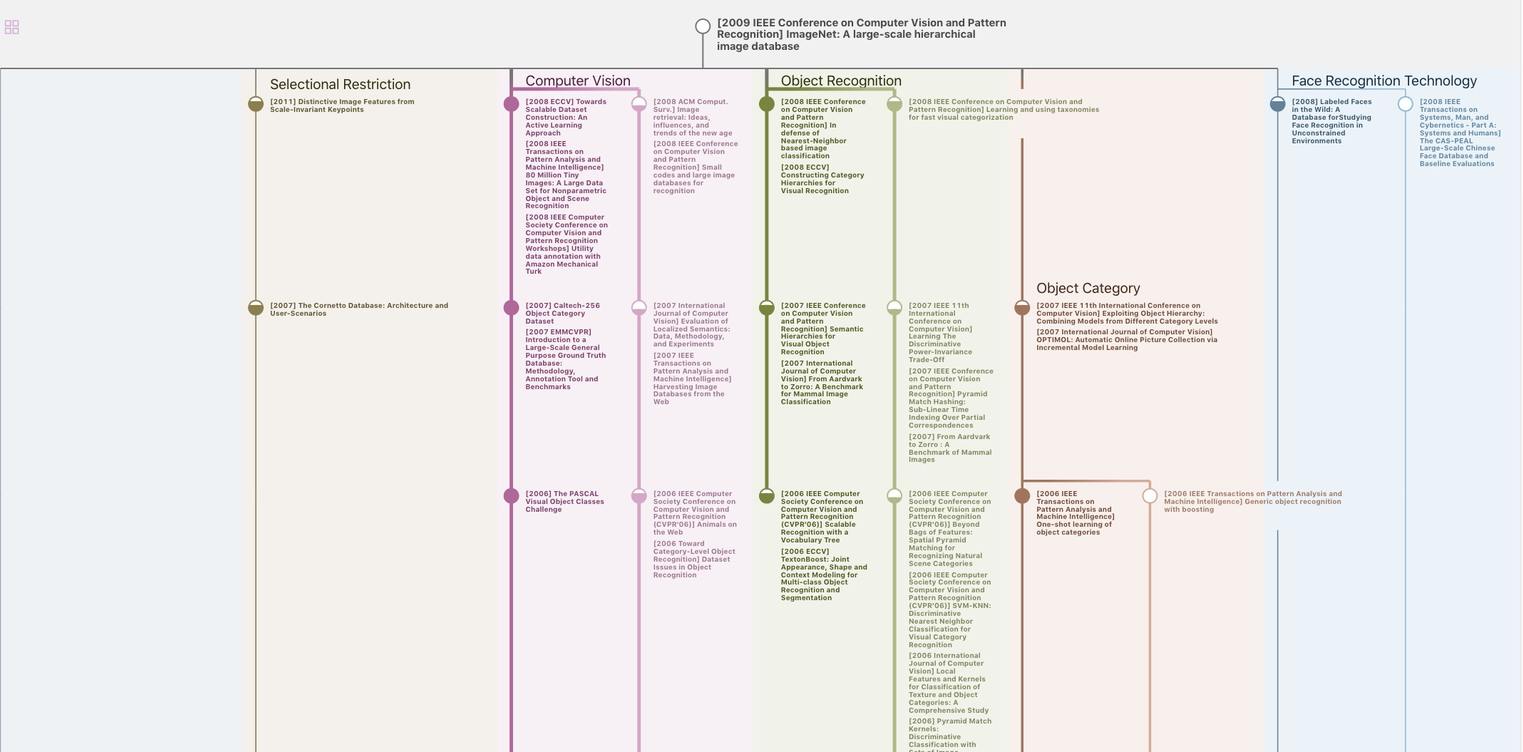
生成溯源树,研究论文发展脉络
Chat Paper
正在生成论文摘要