An efficient quantification of COVID-19 in chest CT images with improved semantic segmentation using U-Net deep structure
INTERNATIONAL JOURNAL OF IMAGING SYSTEMS AND TECHNOLOGY(2023)
摘要
The worldwide spread of the coronavirus (COVID-19) outbreak has proven devastating to public health. The severity of pneumonia relies on a rapid and accurate diagnosis of COVID-19 in CT images. Accordingly, a computed tomography (CT) scan is an excellent screening tool for detecting COVID-19. This paper proposes a deep learning-based strategy for recognizing and segmenting a COVID-19 lesion from chest CT images, which would introduce an accurate computer aided decision criteria for the physicians about the severity rate of the patients. Two main stages have been proposed for detecting COVID-19; first, a convolutional neural network (CNN) deep structure recognizes and classifies COVID-19 from CT images. Second, a U-Net deep structure segments the COVID-19 regions in a semantic manner. The proposed system is trained and evaluated on three different CT datasets for COVID-19, two of which are used to illustrate the system's segmentation performance and the other is to demonstrate the system's classification ability. Experiment results reveal that the proposed CNN can achieve classification accuracy greater than 0.99, and the proposed U-Net model outperforms the state-of-the-art in segmentation with an IOU greater than 0.92.
更多查看译文
关键词
semantic segmentation,chest,images
AI 理解论文
溯源树
样例
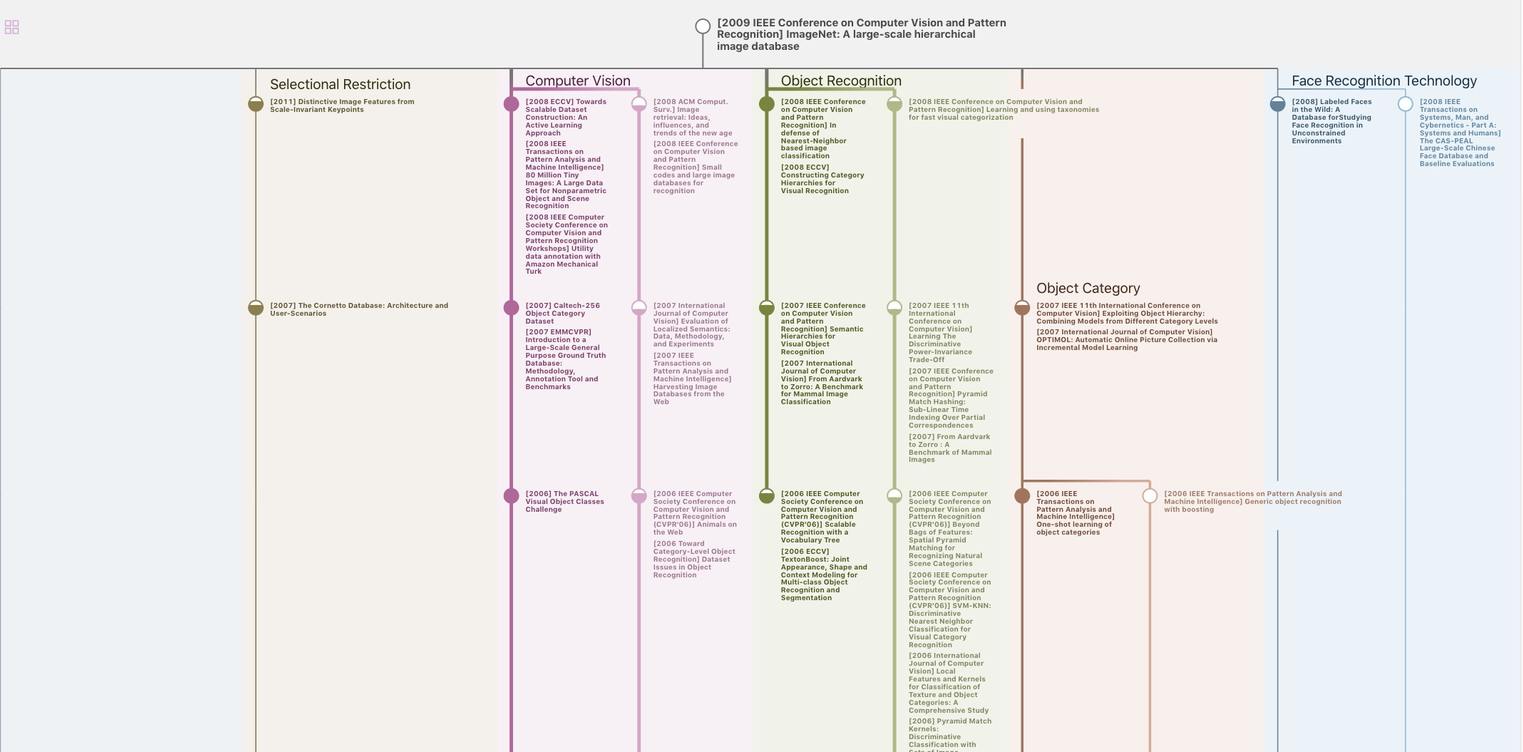
生成溯源树,研究论文发展脉络
Chat Paper
正在生成论文摘要