Hybrid graph transformer networks for multivariate time series anomaly detection
JOURNAL OF SUPERCOMPUTING(2024)
摘要
Anomaly detection for multivariate time series has been widely used in industry and has become one of the hot research problems in the field of data mining. However, the existing anomaly detection methods still have the following limitations: (1) The topological relationship between sensors is complex and nonlinear, and it is difficult to effectively model the inter-sensor dependencies. (2) Most anomaly detection models tend to ignore the critical temporal information in different time steps when capturing the temporal dependencies. To address these problems, we propose a hybrid graph transformer network for multivariate time series anomaly detection (HGTMAD), which combines the transformer with graph convolution to predict multivariate time series-based anomalies. In the time domain, we design a new sparse metric transformer network to capture the time dependence, where the Wasserstein distance is used to measure out significant dot product pairs to learn a better time series representation. In the spatial domain, we design a new channel convolution transformer network fused with a graph convolution network to learn accurately the complex dependencies of multivariate time series in spatial and on feature dimensions based on a combination of local and global approaches. Extensive experiments on publicly available datasets further demonstrate that the proposed HGTMAD significantly outperforms the mainstream state-of-the-art methods.
更多查看译文
关键词
Transformer,Anomaly detection,Multivariate time series,Graph neural network
AI 理解论文
溯源树
样例
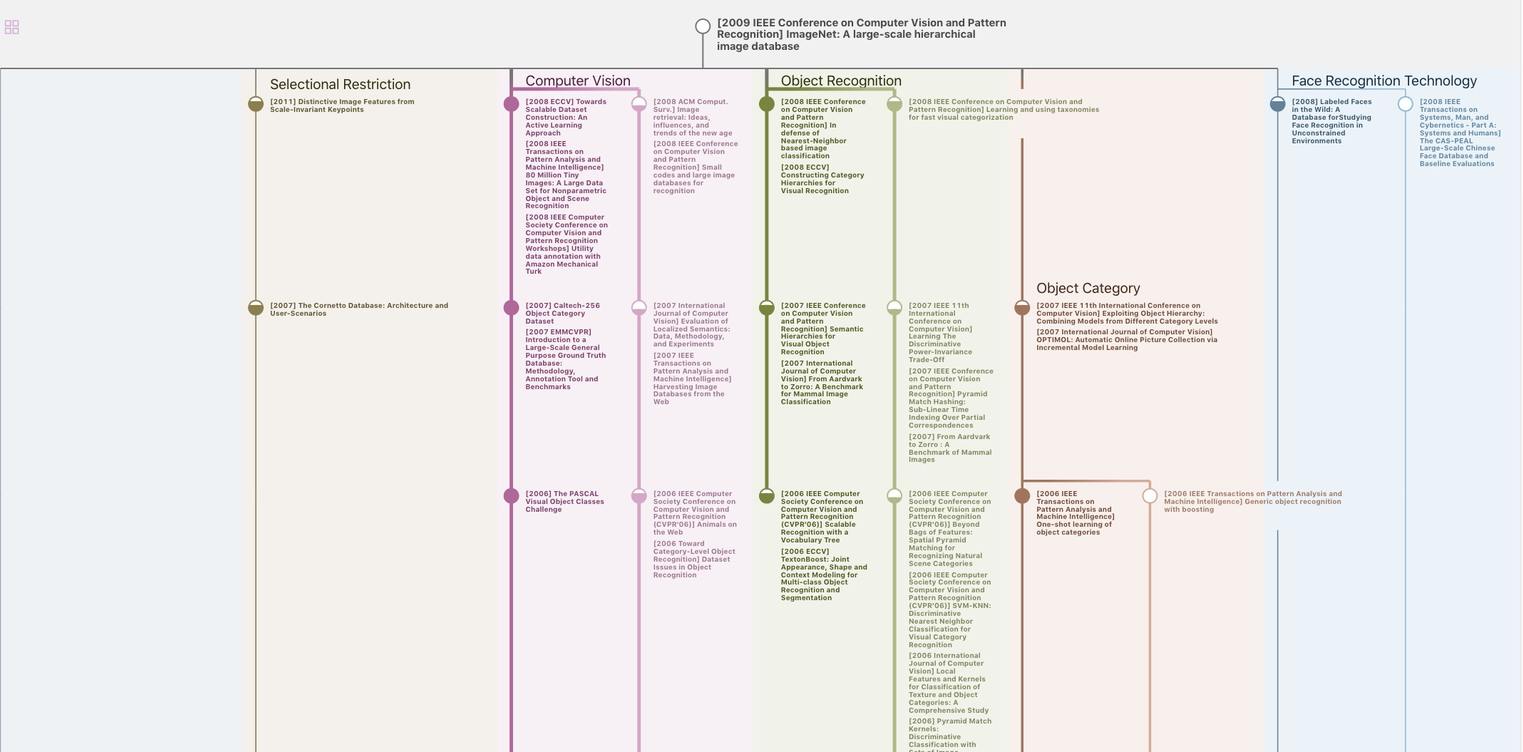
生成溯源树,研究论文发展脉络
Chat Paper
正在生成论文摘要