Analyzing and predicting job failures from HPC system log
JOURNAL OF SUPERCOMPUTING(2024)
摘要
In this paper, we analyze the scheduler log of a production supercomputer that contains complete job information, which is in contrast to many existing (publicly available) HPC logs that only have largely limited job information. We not only provide an in-depth statistical analysis of failed jobs from the scheduler log, but also demonstrate how the scheduler log, which is available in a detailed form, can be leveraged to predict job failures. For the latter, we first conduct a feature analysis based on the framework of 'weight of evidence' and 'information value' to uncover the impact of each workload attribute (feature) on the failure or success of a job, thereby enabling us to identify key features. We then conduct a comparative performance study of six data-driven machine learning models for predicting job failures in a HPC system based on the scheduler log. Our experiment results show that tree-based models exhibit superior performance in terms of both prediction accuracy and computational cost. We also demonstrate that our feature analysis improves the computational efficiency of each machine learning model without losing its prediction performance.
更多查看译文
关键词
HPC systems,Job scheduler log,Job failures,Statistical analysis,Predictive modeling,Feature analysis,Machine learning
AI 理解论文
溯源树
样例
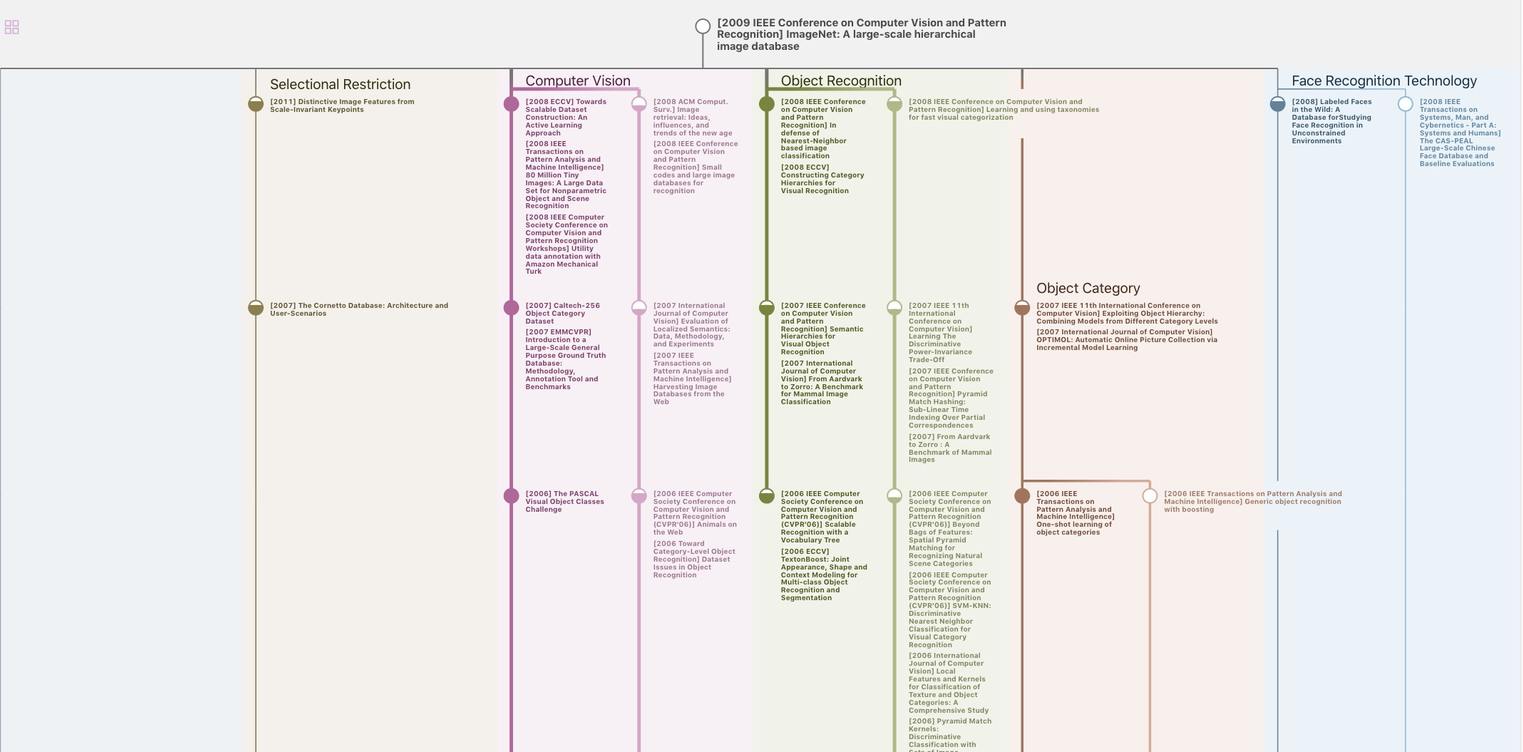
生成溯源树,研究论文发展脉络
Chat Paper
正在生成论文摘要