Representations of Materials for Machine Learning
ANNUAL REVIEW OF MATERIALS RESEARCH(2023)
摘要
High-throughput data generation methods and machine learning (ML) algorithms have given rise to a new era of computational materials science by learning the relations between composition, structure, and properties and by exploiting such relations for design. However, to build these connections, materials data must be translated into a numerical form, called a representation, that can be processed by an ML model. Data sets in materials science vary in format (ranging from images to spectra), size, and fidelity. Predictive models vary in scope and properties of interest. Here, we review context-dependent strategies for constructing representations that enable the use of materials as inputs or outputs for ML models. Furthermore, we discuss how modern ML techniques can learn representations from data and transfer chemical and physical information between tasks. Finally, we outline high-impact questions that have not been fully resolved and thus require further investigation.
更多查看译文
关键词
machine learning,materials,representations
AI 理解论文
溯源树
样例
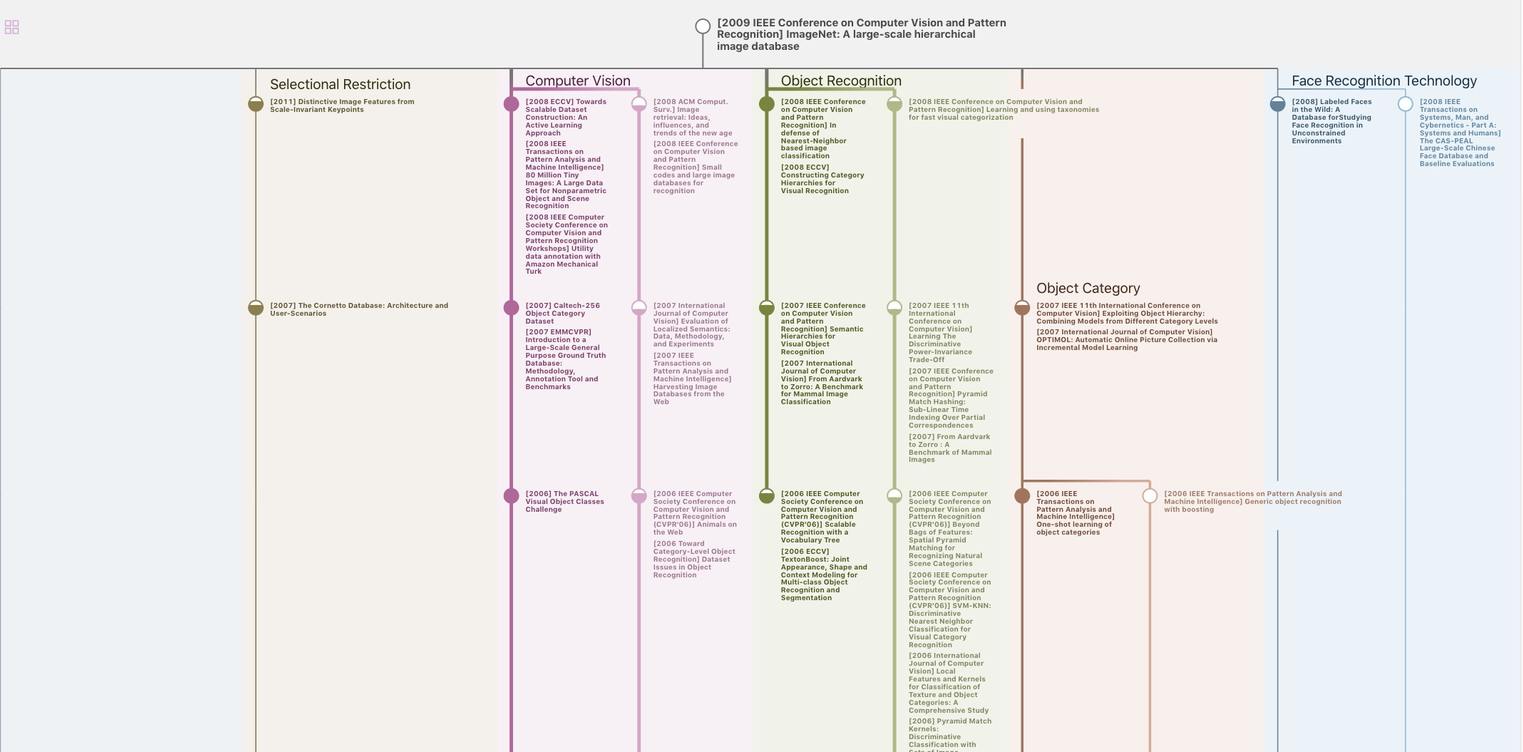
生成溯源树,研究论文发展脉络
Chat Paper
正在生成论文摘要