Shear design of recycled aggregate concrete beams using a data-driven optimization method
STRUCTURES(2023)
摘要
Under the umbrella of sustainable development, recycled aggregate concrete (RAC) is a building material that has attracted a lot of interest. This study proposes a data-driven methodology for shear capacity prediction and design optimization for RAC beams. A comprehensive test dataset with 232 samples was gathered based on clear criteria. Geometric and materials factors are identified as inputs to develop four machine learning (ML) prediction models including artificial neural network, Gaussian process regression, random forest, and categorical gradient boosting (CGB). The winner of ML models is combined with the nondominated sorting genetic algorithm II (NSGA-II) to establish a dual-objective design optimization of RAC beams subjected to shear. Results show that the CGB model achieved the highest accuracy (R2 = 0.972, RMSE = 14.909, and MAPE = 0.086) among four ML models and its standard deviation was more than 80% lower than the five existing equations. The SHapley Additive exPlanations interpreted the CGB model and identified the beam width, nominal strength of stirrups, shear-span ratio, and nominal strength of longitudinal reinforcement are the four main features affecting the shear capacity of a RAC beam. Finally, the Pareto front was created by combining the CGB model with the NSGAII algorithm. The suggested framework provides a novel route for the structural design of RAC beams subject to shear.
更多查看译文
关键词
Recycled aggregate concrete,Beams,Shear strength,Machine learning,Optimization design
AI 理解论文
溯源树
样例
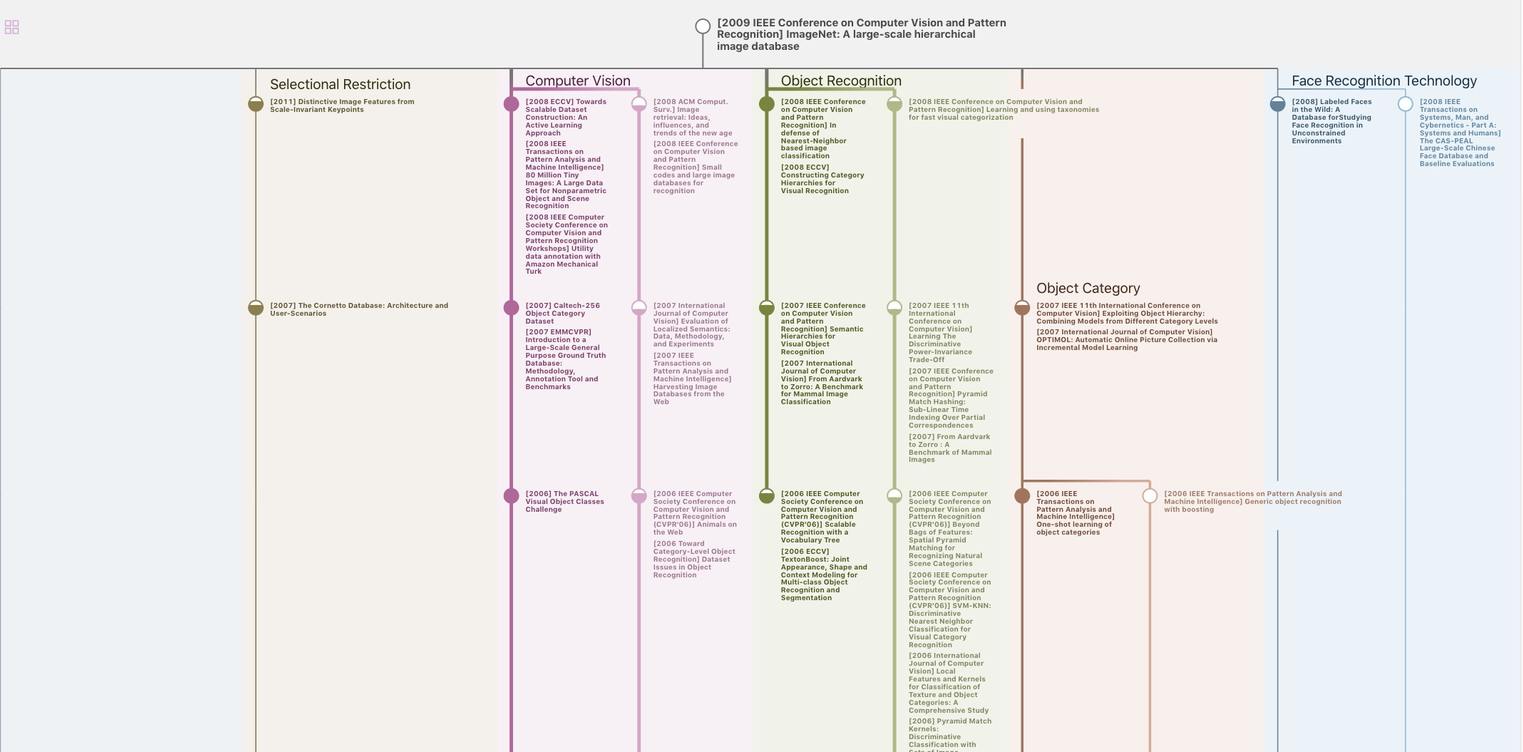
生成溯源树,研究论文发展脉络
Chat Paper
正在生成论文摘要