Study on strawberry fruit detection using lightweight algorithm
MULTIMEDIA TOOLS AND APPLICATIONS(2024)
摘要
A quicker and easier decision-making detection algorithm is important for mobile-phone application to support strawberry management. However, lightweight is a big challenge, including accuracy, speed, and complex conditions confronted by the fruit detection algorithm. For this reason, a lightweight YOLOStrawberry was proposed in this paper based on modified YOLOv5 architecture and compared to other YOLO-lightweight algorithms. YOLOStrawberry incorporated Conv_Maxpool, Shuffle_Block, ResNet, SElayer and SPPF as Backbone network, FPN as Neck network, and CIoU loss function to improve the detection performance of strawberry fruit. The obtained weight-size of YOLOStrawberry is 3.37 MB, which is in third position compared to 1.57 MB for v5lite-e, 3.19 MB for v5lite-s, 3.56 MB for YOLOv5n, 8.60 MB for v5lite-c and 10.7 MB for v5lite-g. Nevertheless, the required trained time for YOLOStrawberry is 0.93%, 0.69%, 0.62%, 0.71%, 1.14% less than v5lite-c, v5lite-e, v5lite-g, v5lite-s and YOLOv5n, respectively. Meanwhile, the average precision (AP) detection level is measured as 89.7% of YOLOStrawberry is 0.4%, 0.7%, 3%, 3.4% and 9.1% more accurate than v5lite-g, YOLOv5n, v5lite-c, v5lite-s and v5lite-e, respectively. Furthermore, the tested speed time of YOLOStrawberry is 7.30 ms, faster compared to 11.7 ms for YOLOv5n, 13.2 ms for v5lite-g, 13.3 ms for v5lite-e, 14.0 ms for v5lite-s, and 16.2 ms for v5lite-c. Therefore, YOLOStrawberry algorithm is lightweight, robust, accurate, fast, applicable to mobile-phone for real-time and extendable to other fruits for detection.
更多查看译文
关键词
YOLOStrawberry,Fruit detection,Lightweight,Detection performance,Mobile-phone application
AI 理解论文
溯源树
样例
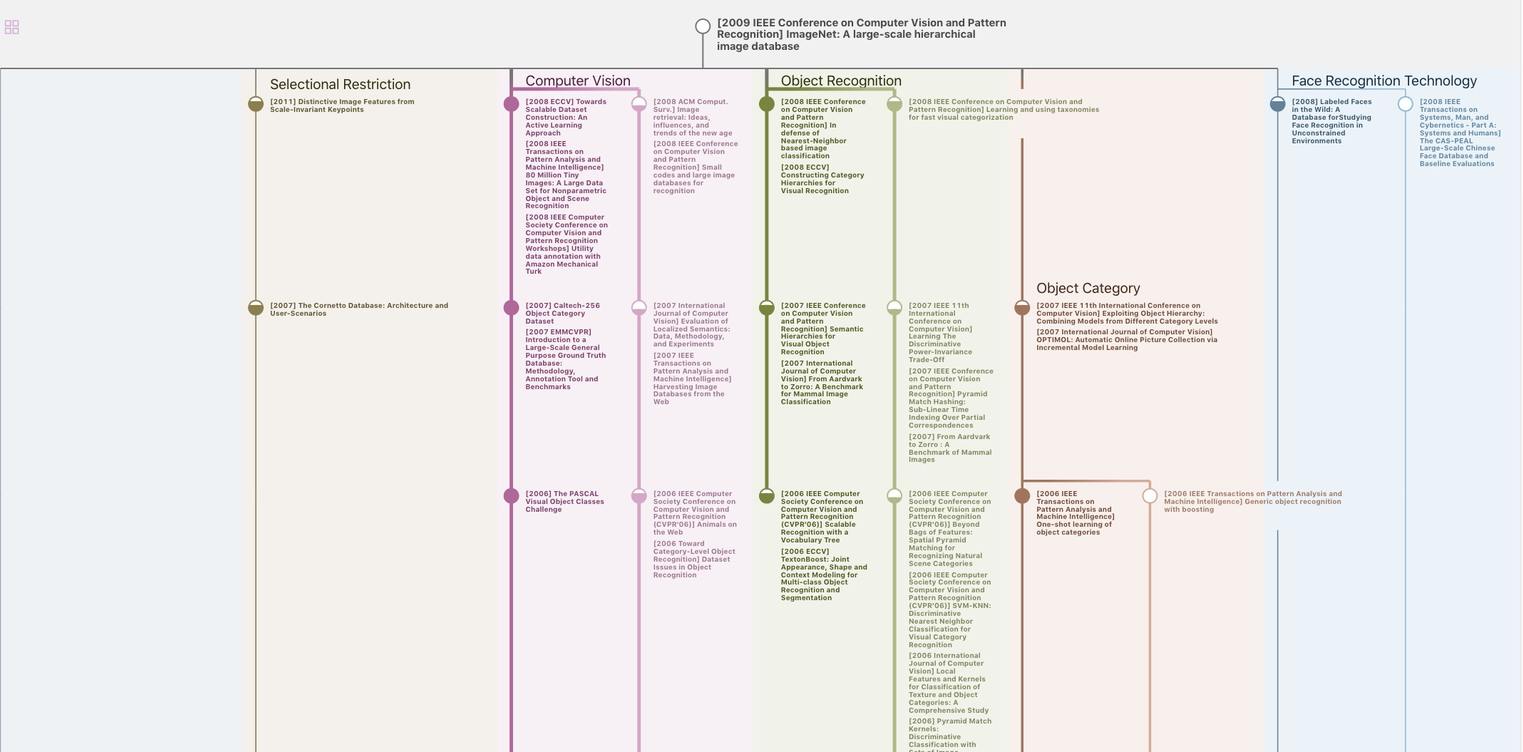
生成溯源树,研究论文发展脉络
Chat Paper
正在生成论文摘要