Epipolar Transformer for Multi-view Human Pose Estimation
2020 IEEE/CVF CONFERENCE ON COMPUTER VISION AND PATTERN RECOGNITION WORKSHOPS (CVPRW 2020)(2020)
摘要
A common way to localize 3D human joints in a synchronized and calibrated multi-view setup is a two-step process: (1) apply a 2D detector separately on each view to localize joints in 2D, (2) robust triangulation on 2D detections from each view to acquire the 3D joint locations. However, in step 1, the 2D detector is constrained to solve challenging cases which could be better resolved in 3D, such as occlusions and oblique viewing angles, purely in 2D without leveraging any 3D information. Therefore, we propose the differentiable "epipolar transformer", which empowers the 2D detector to leverage 3D-aware features to improve 2D pose estimation. The intuition is: given a 2D location p in the reference view, we would like to first find its corresponding point p' in the source view, then combine the features at p' with the features at p, thus leading to a more 3D-aware feature at p. Inspired by stereo matching, the epipolar transformer leverages epipolar constraints and feature matching to approximate the features at p'. The key advantages of the epipolar transformer are: (1) it has minimal learnable parameters, (2) it can be easily plugged into existing networks, moreover (3) it is interpretable, i.e., we can analyze the location p' to understand whether matching over the epipolar line was successful. Experiments on Human3.6M [9] show that our approach has consistent improvements over the baselines. Specifically, in the condition where no external data is used, our Human3.6M model trained with ResNet-50 and image size 256x256 outperforms state-of-the-art by a large margin and achieves MPJPE 26.9 mm. Code is available1. This is the workshop version of our CVPR 2020 paper [8]
更多查看译文
关键词
oblique viewing angles,differentiable epipolar transformer,2D pose estimation,3D-aware feature,stereo matching,epipolar line,3D human joint locations,multiview human pose estimation,2D detector,Human3.6M model,ResNet-50,feature matching,size 26.9 mm
AI 理解论文
溯源树
样例
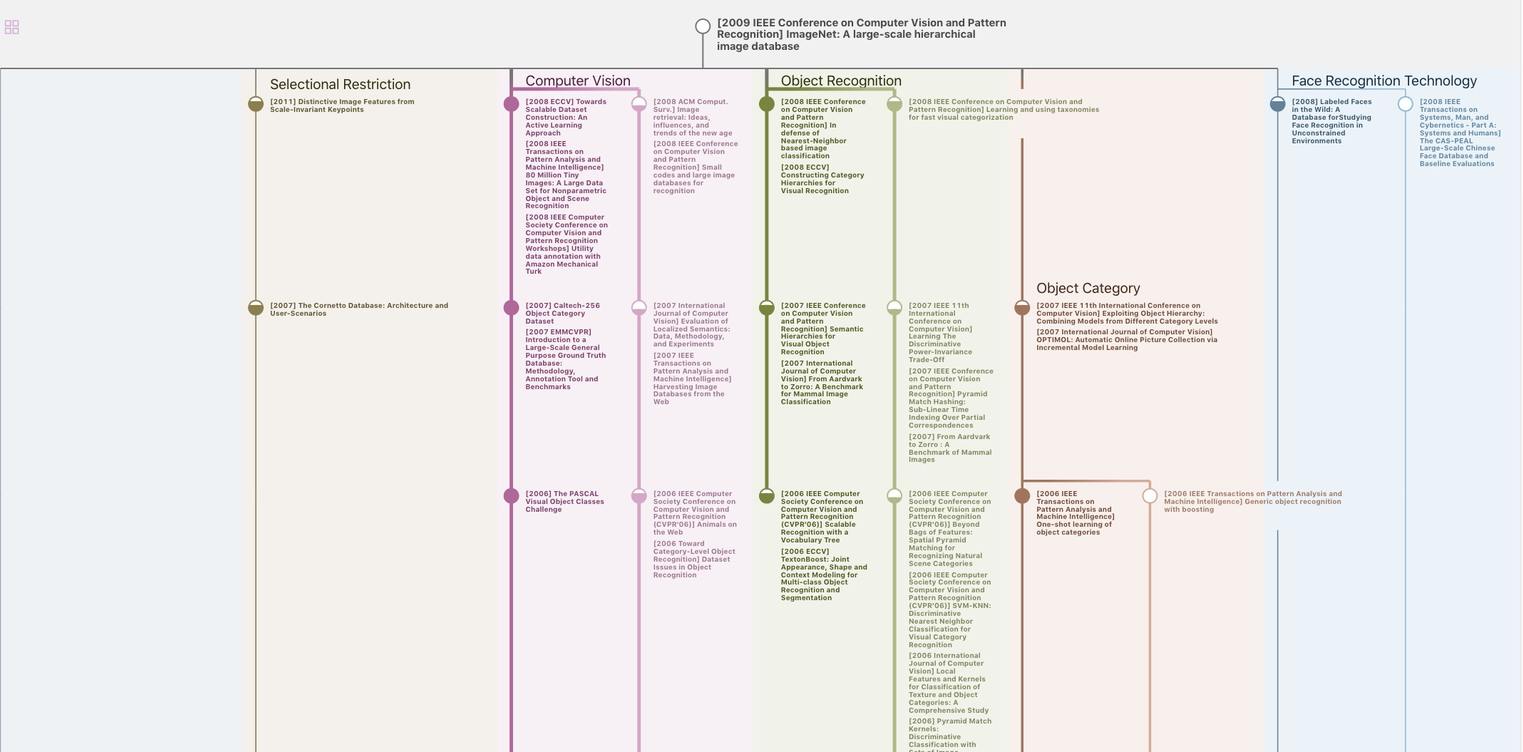
生成溯源树,研究论文发展脉络
Chat Paper
正在生成论文摘要