Learning of discrete models of variational PDEs from data
CHAOS(2024)
摘要
We show how to learn discrete field theories from observational data of fields on a space-time lattice. For this, we train a neural network model of a discrete Lagrangian density such that the discrete Euler-Lagrange equations are consistent with the given training data. We, thus, obtain a structure-preserving machine learning architecture. Lagrangian densities are not uniquely defined by the solutions of a field theory. We introduce a technique to derive regularizers for the training process which optimize numerical regularity of the discrete field theory. Minimization of the regularizers guarantees that close to the training data the discrete field theory behaves robust and efficient when used in numerical simulations. Further, we show how to identify structurally simple solutions of the underlying continuous field theory such as traveling waves. This is possible even when traveling waves are not present in the training data. This is compared to data-driven model order reduction based approaches, which struggle to identify suitable latent spaces containing structurally simple solutions when these are not present in the training data. Ideas are demonstrated on examples based on the wave equation and the Schrodinger equation.
更多查看译文
关键词
variational pdes,discrete models,learning,data
AI 理解论文
溯源树
样例
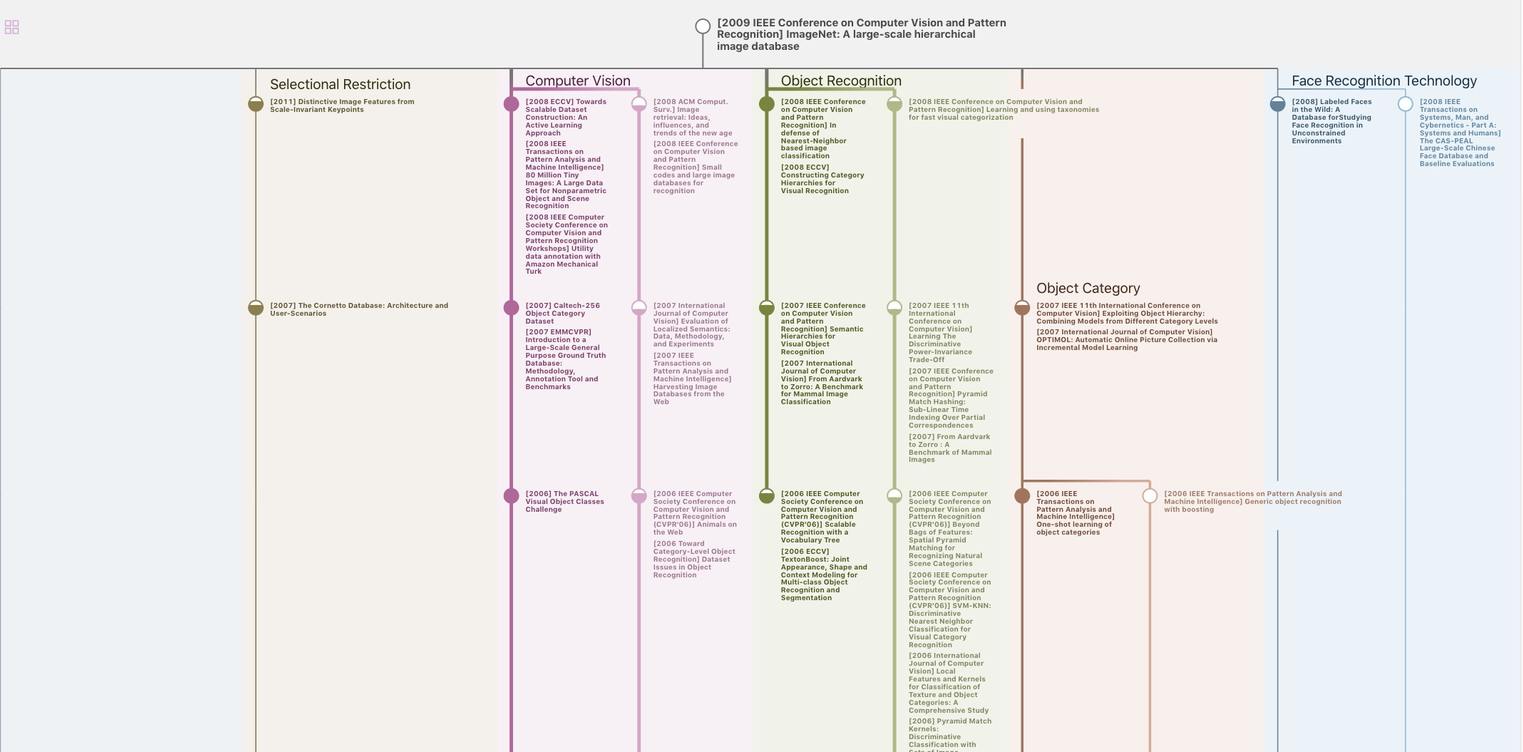
生成溯源树,研究论文发展脉络
Chat Paper
正在生成论文摘要