Competitions in AI -- Robustly Ranking Solvers Using Statistical Resampling
CoRR(2023)
摘要
Solver competitions play a prominent role in assessing and advancing the state of the art for solving many problems in AI and beyond. Notably, in many areas of AI, competitions have had substantial impact in guiding research and applications for many years, and for a solver to be ranked highly in a competition carries considerable weight. But to which extent can we expect competition results to generalise to sets of problem instances different from those used in a particular competition? This is the question we investigate here, using statistical resampling techniques. We show that the rankings resulting from the standard interpretation of competition results can be very sensitive to even minor changes in the benchmark instance set used as the basis for assessment and can therefore not be expected to carry over to other samples from the same underlying instance distribution. To address this problem, we introduce a novel approach to statistically meaningful analysis of competition results based on resampling performance data. Our approach produces confidence intervals of competition scores as well as statistically robust solver rankings with bounded error. Applied to recent SAT, AI planning and computer vision competitions, our analysis reveals frequent statistical ties in solver performance as well as some inversions of ranks compared to the official results based on simple scoring.
更多查看译文
关键词
robustly ranking solvers,ai
AI 理解论文
溯源树
样例
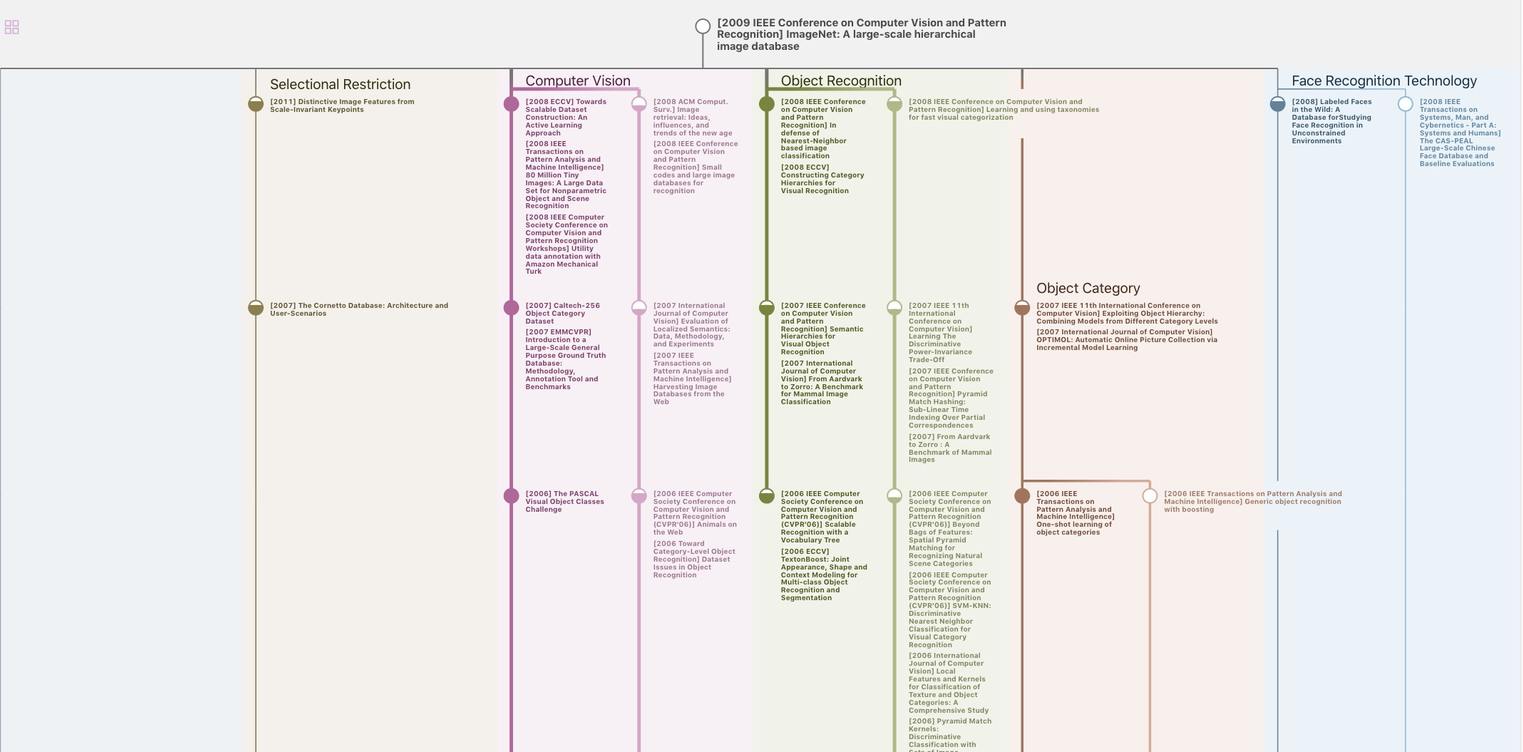
生成溯源树,研究论文发展脉络
Chat Paper
正在生成论文摘要