Dual Intents Graph Modeling for User-centric Group Discovery
PROCEEDINGS OF THE 32ND ACM INTERNATIONAL CONFERENCE ON INFORMATION AND KNOWLEDGE MANAGEMENT, CIKM 2023(2023)
摘要
Online groups have become increasingly prevalent, providing users with space to share experiences and explore interests. Therefore, user-centric group discovery task, i.e., recommending groups to users can help both users' online experiences and platforms' long-term developments. Existing recommender methods can not deal with this task as modeling user-group participation into a bipartite graph overlooks their item-side interests. Although there exist a few works attempting to address this task, they still fall short in fully preserving the social context and ensuring effective interest representation learning. In this paper, we focus on exploring the intents that motivate users to participate in groups, which can be categorized into different types, like the social-intent and the personal interest-intent. The former refers to users joining a group affected by their social links, while the latter relates to users joining groups with like-minded people for interest-oriented self-enjoyment. To comprehend different intents, we propose a novel model, DiRec, that first models each intent separately and then fuses them together for predictions. Specifically, for social-intent, we introduce the hypergraph structure to model the relationship between groups and members. This allows for more comprehensive group information preservation, leading to a richer understanding of the social context. As for interest-intent, we employ novel structural refinement on the interactive graph to uncover more intricate user behaviors, item characteristics, and group interests, realizing better representation learning of interests. Furthermore, we also observe the intent overlapping in real-world scenarios and devise a novel self-supervised learning loss that encourages such alignment for final recommendations. Extensive experiments on three public datasets show the significant improvement of DiRec over the state-of-the-art methods.
更多查看译文
关键词
Recommender Systems,Graph Neural Networks,Group Recommendation,Data Mining
AI 理解论文
溯源树
样例
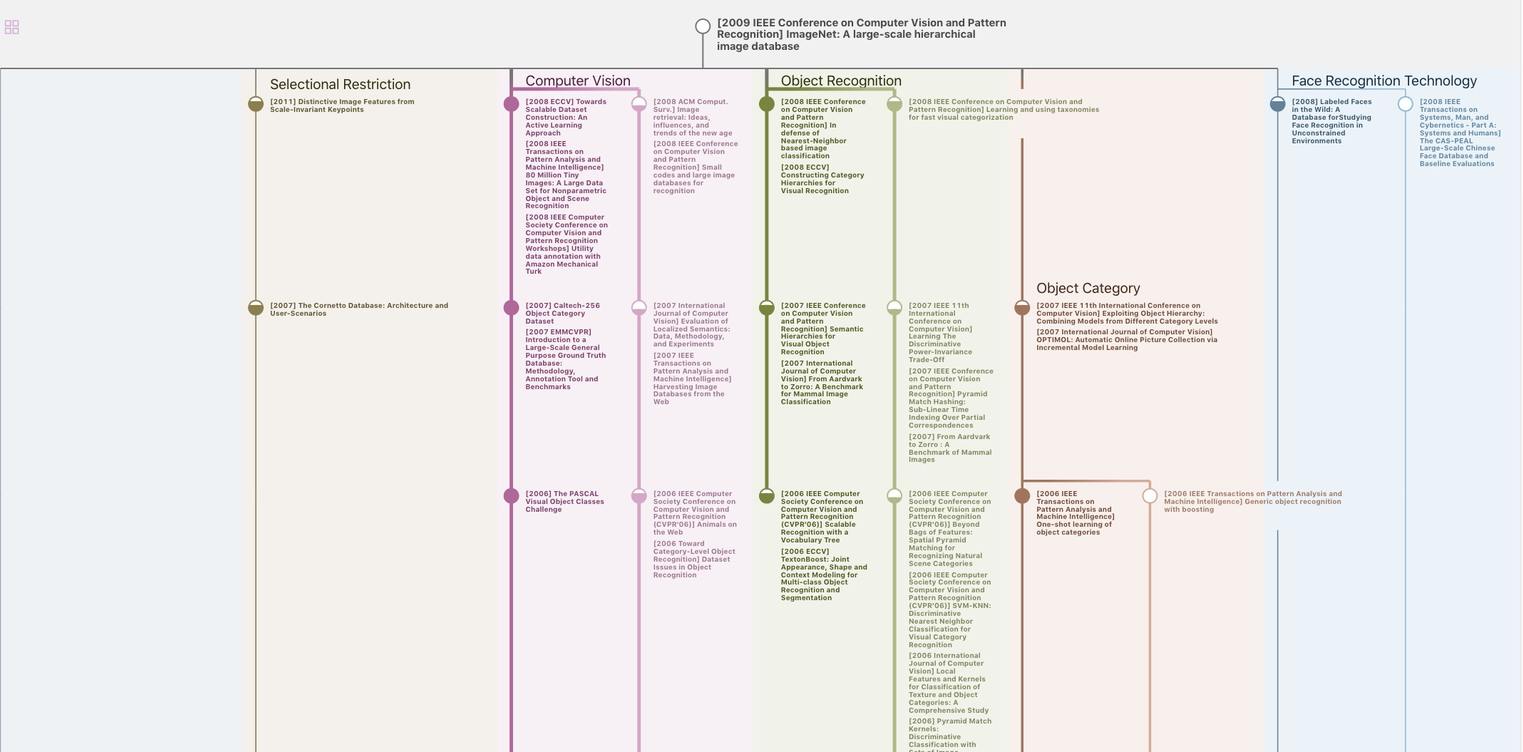
生成溯源树,研究论文发展脉络
Chat Paper
正在生成论文摘要