Representation Learning for Audio Privacy Preservation using Source Separation and Robust Adversarial Learning
CoRR(2023)
摘要
Privacy preservation has long been a concern in smart acoustic monitoring systems, where speech can be passively recorded along with a target signal in the system's operating environment. In this study, we propose the integration of two commonly used approaches in privacy preservation: source separation and adversarial representation learning. The proposed system learns the latent representation of audio recordings such that it prevents differentiating between speech and non-speech recordings. Initially, the source separation network filters out some of the privacy-sensitive data, and during the adversarial learning process, the system will learn privacy-preserving representation on the filtered signal. We demonstrate the effectiveness of our proposed method by comparing our method against systems without source separation, without adversarial learning, and without both. Overall, our results suggest that the proposed system can significantly improve speech privacy preservation compared to that of using source separation or adversarial learning solely while maintaining good performance in the acoustic monitoring task.
更多查看译文
关键词
audio privacy preservation
AI 理解论文
溯源树
样例
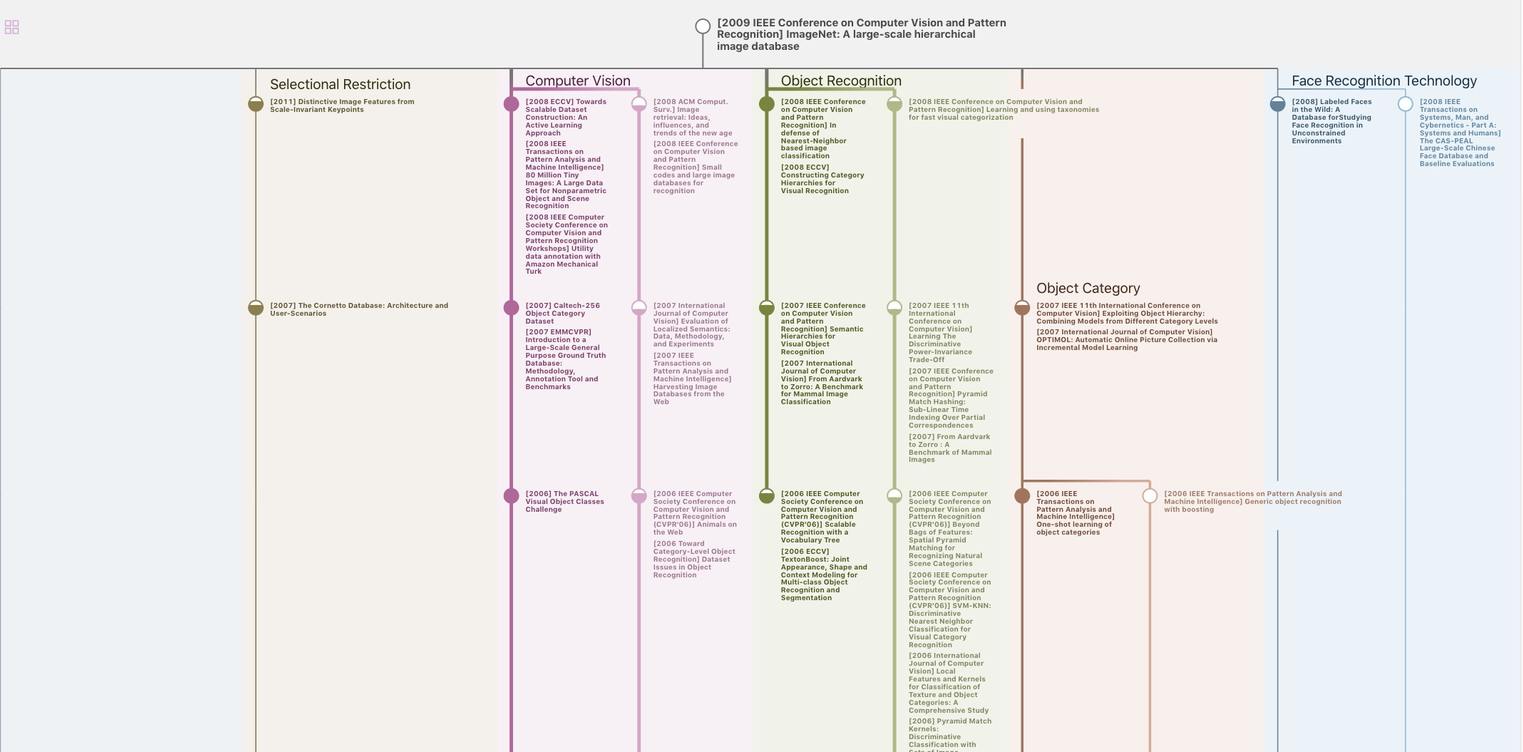
生成溯源树,研究论文发展脉络
Chat Paper
正在生成论文摘要