A deep-learning method using single phantom to enhance megavoltage image quality for patient positioning in chest radiotherapy: a feasibility study
JOURNAL OF THE KOREAN PHYSICAL SOCIETY(2023)
摘要
Image-guided radiation treatment (IGRT) is essential for verifying patient positioning during modern radiotherapy. Although megavoltage digital radiographs (MV-DR) are available on most therapeutic linear accelerators and can be used for checking treatment beam shapes, they are much inferior to kilovoltage digital radiographs (KV-DR) in terms of image quality. As it is generally challenging to obtain a well-aligned MV − KV training dataset of patients in clinical scenarios, there is a lack of sufficient information on the accuracy of KV-DR synthesized using supervised training. Therefore, we aimed to synthesize pseudo KV-DR (pKV-DR) from MV-DR using a training dataset developed with a single anthropomorphic chest phantom. The phantom was adopted to obtain MV − KV image pairs at various gantry angles because these image pairs of patients are highly difficult to acquire and exactly align with each other. A deep-learning model based on U-net architecture was trained with the phantom image pairs using the mean absolute error (MAE) and structure similarity (SSIM) indices as loss functions. The mean MAEs of MV-DR and pKV-DR against KV-DR as the ground truth were 0.1152 and 0.0169, respectively, and their mean SSIM values were 0.9693 and 0.9942, respectively. Finally, pKV-DR showed a relatively high image similarity to that of KV-DR with smaller MAE (14.7%) and higher SSIM (2.5%), compared with MV-DR. The image contrast was also improved by 37.1% in clinical cases. The proposed method is expected to enable the implementation of improved IGRT with high image quality of KV-DR level, even in clinics where MV-DR is only available.
更多查看译文
关键词
Deep learning,Megavoltage image,Kilovoltage image,Radiotherapy,Patient setup
AI 理解论文
溯源树
样例
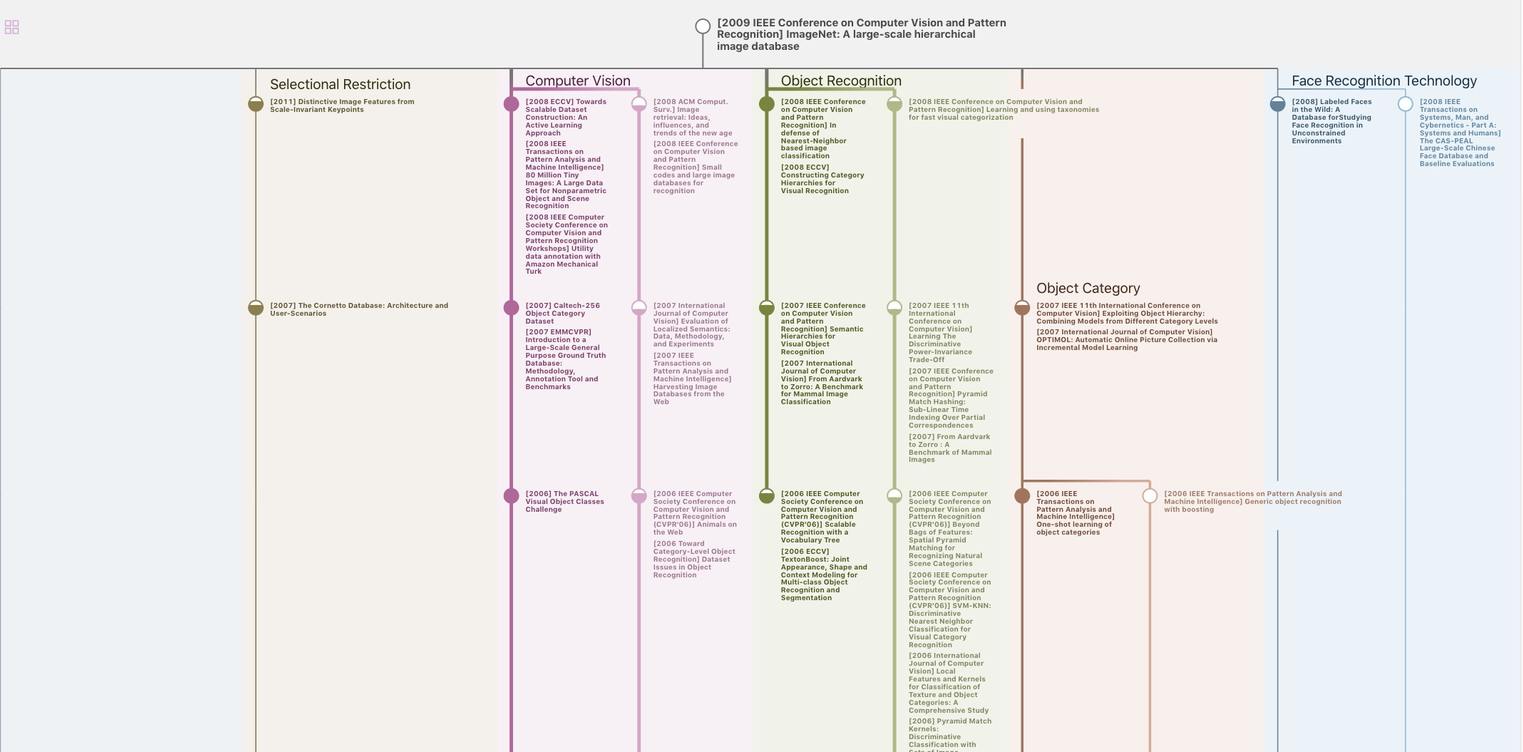
生成溯源树,研究论文发展脉络
Chat Paper
正在生成论文摘要