Hyperspectral Image Classification Aided by LiDAR Data
FOURTEENTH INTERNATIONAL CONFERENCE ON GRAPHICS AND IMAGE PROCESSING, ICGIP 2022(2022)
摘要
Spectral variability and shadow effects can limit the hyperspectral image (HSI) classification performance. Compared with HSI, the LiDAR data is an excellent complement with its abundant elevation information. In this study, a procedure including pre-processing, deep residual network classification and post-processing is investigated for classification of HSI aided by the LiDAR data to release the problem of identifying shaded objects and spectral variability. Specifically, three aspects with respect to spectral band selection using Archetypal Analysis (AA), feature level fusion based classification by deploying a residual network associated approach and label correction utilizing the elevation information, are explored to realize more accurate classification. Experiments on three public multi-source (hyperspectral and LiDAR) remote sensing datasets show more promising classification can be achieved via fusion two-source of remote sensing data than that using only independent hyperspectral image. In particular, on the Houston 2017 dataset, OA and Kappa achieved significant gains of 2.47% and 2.79% respectively after incorporating LiDAR information. Moreover, the results demonstrate the elevation information used independently in the post-processing stage can help with effective refinement of classification results.
更多查看译文
关键词
Deep learning,hyperspectral classification,LiDAR,shadow effect,spectral variability
AI 理解论文
溯源树
样例
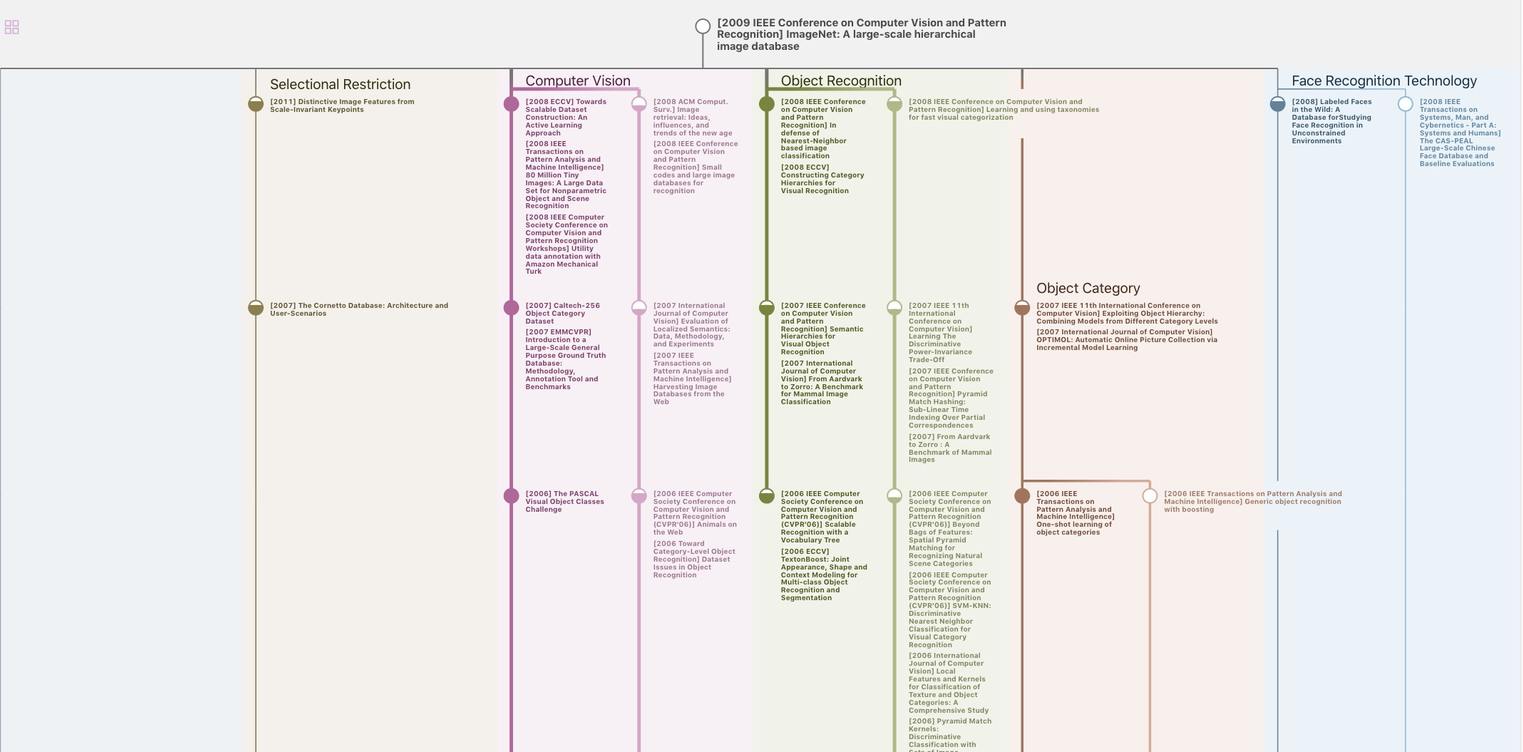
生成溯源树,研究论文发展脉络
Chat Paper
正在生成论文摘要