Multiscale Fusion and Convolution Spatial Propagation Networks for Deep Complementation of Outdoor Scenes
FOURTEENTH INTERNATIONAL CONFERENCE ON GRAPHICS AND IMAGE PROCESSING, ICGIP 2022(2022)
摘要
In autonomous driving and other robotics, rich depth perception is critical for 3D reconstruction tasks of outdoor landscapes. Many neural networks combine sparse depth maps with high-quality RGB images to generate a dense effect, resulting in dense depth maps. However, they frequently combine LiDAR and RGB image data by conducting feature concatenation or element addition, which results in the loss of some features as well as changes to the depth values of the original sparse data. To address these issues, this article proposes that the relationship between spatial and channel attention be used to link local and global features in order to accurately complete and correct sparse input so that the RGB images can better lead the depth completion job. We also use an affinity matrix to keep the original depth values in order to make the RGB image simply act as a guide without modifying the original pixel depth. We tested and assessed our algorithm on the KITTI dataset, and it outperformed the existing network in outdoor situations.
更多查看译文
关键词
Depth completion, Feature extraction, Model refinement, Multi-modal fusion, Convolution neural networks
AI 理解论文
溯源树
样例
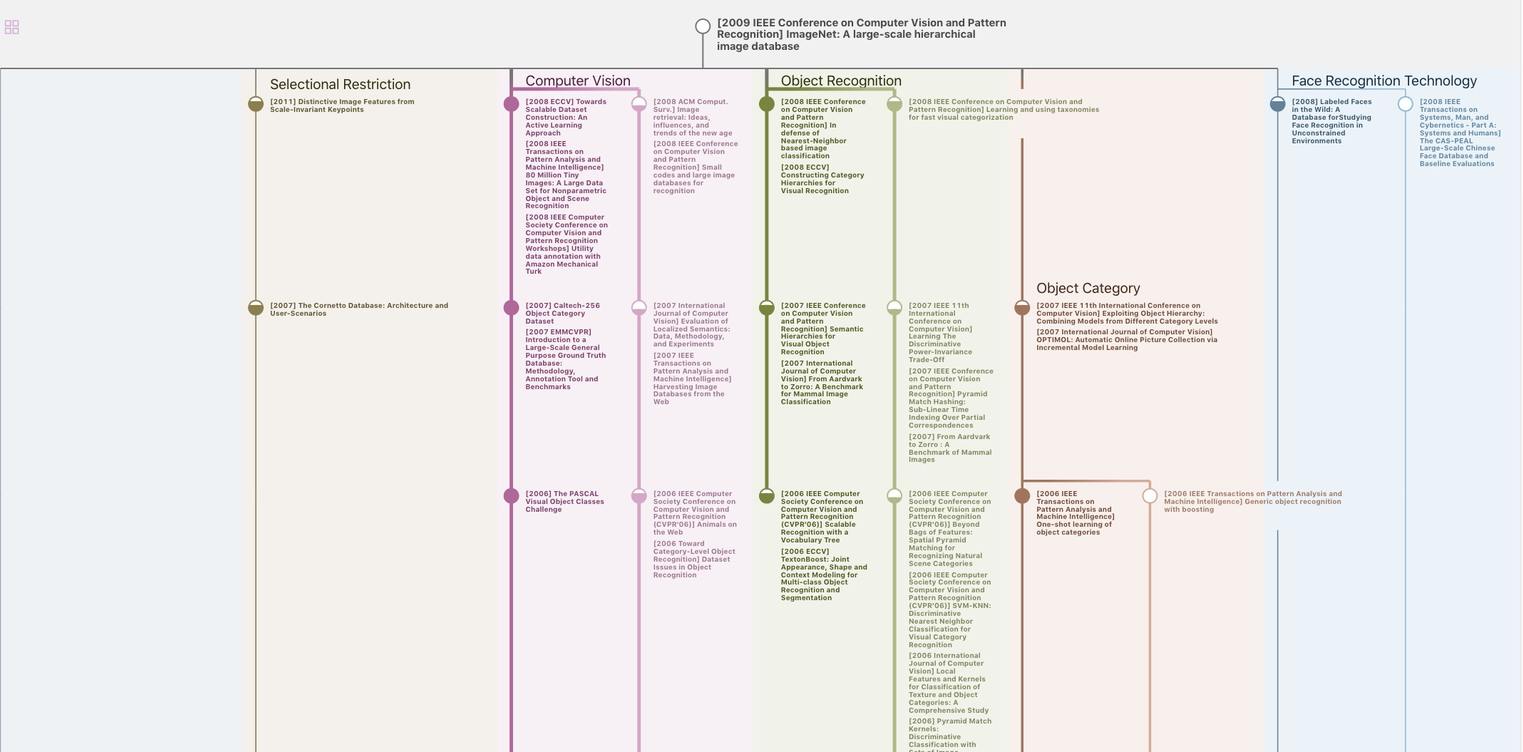
生成溯源树,研究论文发展脉络
Chat Paper
正在生成论文摘要