Global accurate Multi-view Point Cloud Registration Based on Manifold Clustering and Thermal Gradient Method
FOURTEENTH INTERNATIONAL CONFERENCE ON GRAPHICS AND IMAGE PROCESSING, ICGIP 2022(2022)
摘要
Distances are the basic measures used in data clustering. The selection of the distance has a significant impact on the final result. Euclidean distances do not accurately reflect the actual geometrical properties of complex shape and structure, while the geodesic distance may give full consideration to their geometry. Therefore, in the registration process, geodesic distance is more appropriate in such cases. In this study, a multi-view point cloud registration method is proposed to solve the problem of low registration accuracy of objects with complex shape and structure. The method transforms the registration problem into a clustering problem, and the rigid transformation is updated while updating the centroids. Clustering and rigid transformation are alternately applied to the point cloud to achieve final registration results. The proposed method is validated by experiments using data from Stanford University's public datasets. Experimental results show better accuracy and robustness of the proposed method.
更多查看译文
关键词
Multi-view registration,Manifold clustering,Geodesic distance,Thermal gradient,Neighborhood feature points
AI 理解论文
溯源树
样例
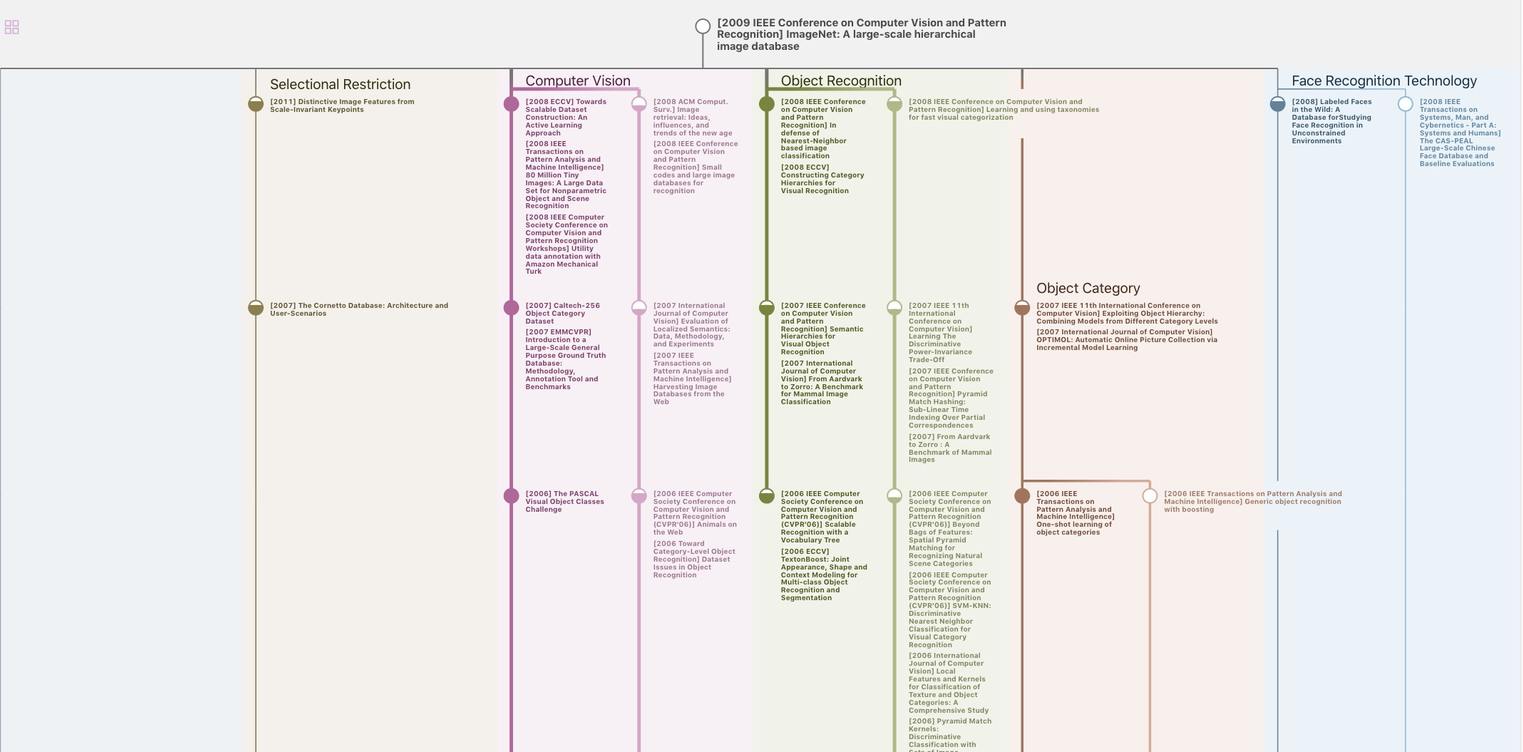
生成溯源树,研究论文发展脉络
Chat Paper
正在生成论文摘要