SEFWaM–deep learning based smart ensembled framework for waste management
ENVIRONMENT DEVELOPMENT AND SUSTAINABILITY(2023)
摘要
Waste generation has seen a significant surge in the last decade, presenting an urgent need for efficient and sustainable waste management strategies. The mounting piles of landfill waste underscore the criticality of segregating recyclable from non-recyclable waste, a measure that could alleviate numerous global environmental challenges. The increasing necessity to differentiate waste into specific categories such as paper, plastic, and metal is becoming evident; these categories demand distinct disposal and recycling methods. Addressing this automatic detection and segregation issue calls for an automated garbage classification system, achievable through computer vision. This paper introduces a deep learning and computer vision-based Smart Ensembled Framework for Waste Management (SEFWaM) to categorize garbage into various classes for improved waste management. This innovative model employs a fusion of transfer learning—a deep learning technique and boosting to devise an ensembled approach for efficient waste classification. Our proposed approach, trained on the Trashnet 2.0 dataset, has demonstrated superior performance over competing algorithms in terms of Weighted Macro Precision (WMP), Weighted Macro Recall (WMR), Weighted Macro F1-Score (WMF), and test accuracy. The proposed SEFWaM model achieves an accuracy of 94.2% for the considered dataset, proving its superior efficiency over other deep learning-based models. This research thereby contributes to a pressing environmental issue by offering an automated, efficient solution for waste management.
更多查看译文
关键词
sefwam–deep,smart
AI 理解论文
溯源树
样例
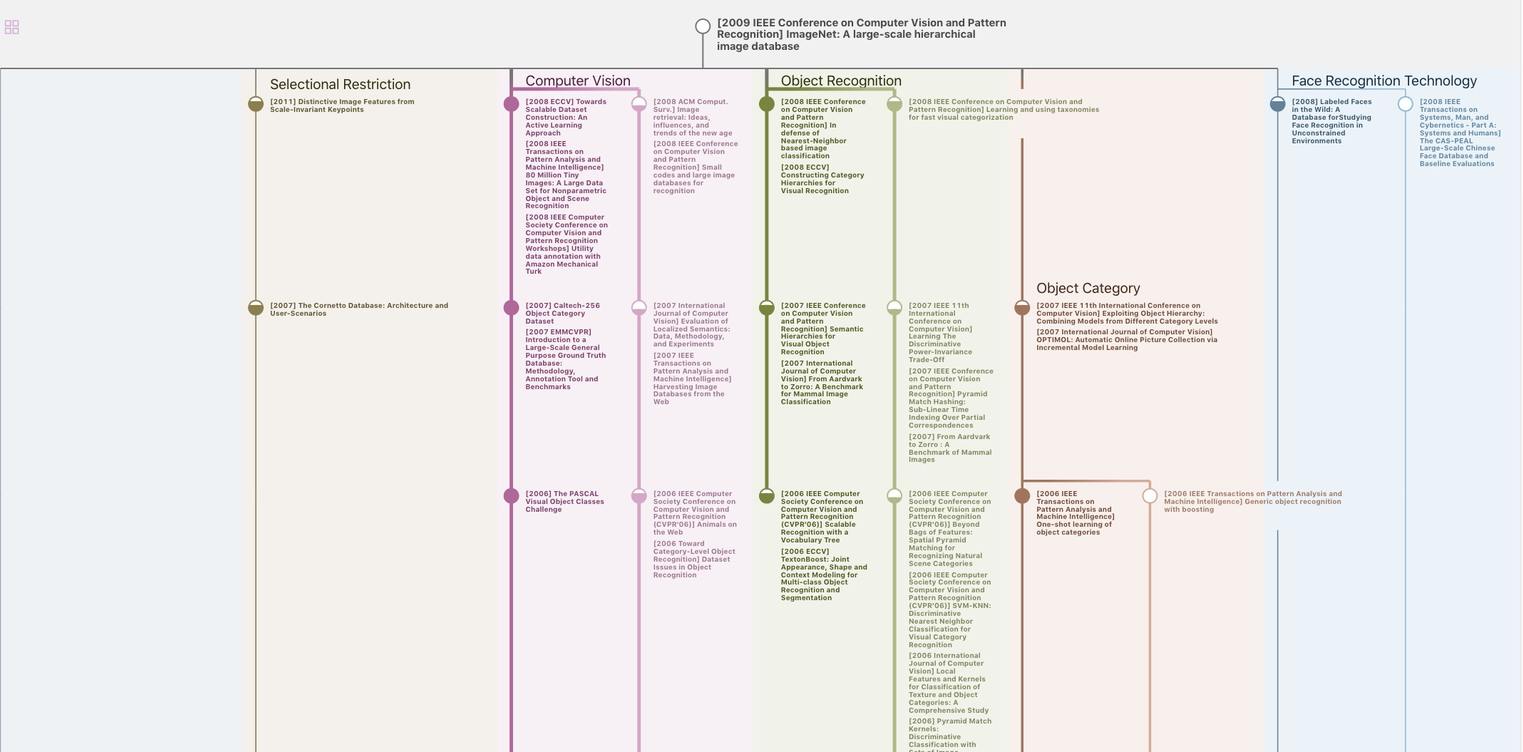
生成溯源树,研究论文发展脉络
Chat Paper
正在生成论文摘要