Adaptive scale based U-shape transformer network for ischemic stroke lesion segmentation in CTP images
FOURTEENTH INTERNATIONAL CONFERENCE ON GRAPHICS AND IMAGE PROCESSING, ICGIP 2022(2022)
摘要
Ischemic stroke lesion segmentation in Computed Tomography Perfusion (CTP) images is crucial for the quantitative diagnosis of stroke. However, it remains a challenging problem due to the poor image quality of CTP and the complex appearance of the lesions. In this study, we develop a U-shape transformer network with an adaptive scale for ischemic stroke lesion segmentation in CTP images. The state-of-the-art nnU-Net structure is used as the backbone, and a transformer block with self-adapting scale is introduced. The proposed network adopts the advantage of transformer in capturing global information and retains the advantage of convolutional neural network (CNN) in extracting local correlation features. In order to obtain better adaptation of transformer block to ischemic stroke segmentation task, we propose a self-adapting scale selection strategy that offer better patch size and window size to assist the transformer block capture more global information and avoid semantic information being corrupted. Five-fold cross-validation was used in training of the networks, and nnUNet was used as a baseline model in the performance evaluation. The results showed that after involving the proposed method, the mean DICE of the segmentation improved from 0.72 to 0.78 in the ISLES public dataset. For the independent test set, the proposed method achieved a mean DICE of 0.48, a mean precision of 0.60, and a mean recall of 0.46, compared to 0.46, 0.57 and 0.43 by the baseline model. The proposed framework has the potential for improving diagnosis and treatment of ischemic stroke in CTP.
更多查看译文
关键词
Ischemic Stroke Segmentation,Transformer,Adaptive Scale,CTP
AI 理解论文
溯源树
样例
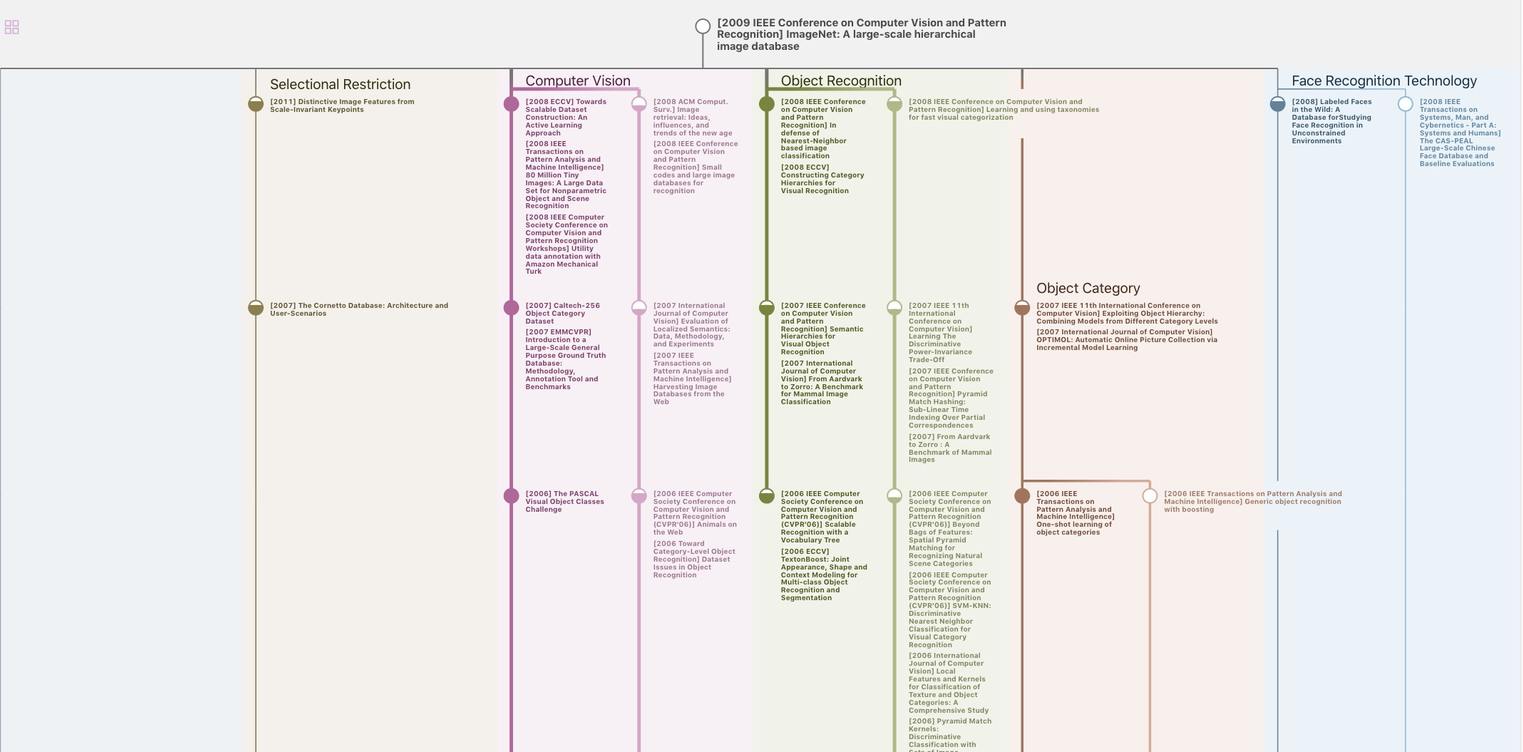
生成溯源树,研究论文发展脉络
Chat Paper
正在生成论文摘要