HetGNN-SF: Self-supervised learning on heterogeneous graph neural network via semantic strength and feature similarity
APPLIED INTELLIGENCE(2023)
摘要
Heterogeneous graph neural networks (HGNNs) can effectively model multiple node types and complex interactions in real networks and solve problems in various practical applications. Self-supervised Learning-based HGNNs (SL-HGNNs) have become the current research focus in this field because they can solve the problem of difficult label acquisition in practical scenarios. These methods usually split heterogeneous graphs into multiple subgraphs based on meta-paths for separate study. However, they ignore the complex interactions between the different semantics of the graphs. In addition, they use node features as auxiliary information for heterogeneous graph representation learning and ignore the importance of features. To solve the above problems, we propose a Self-supervised Learning on Heterogeneous Graph Neural Network via Semantic strength and Feature similarity (HetGNN-SF) model. This model innovatively implements a Feature- and Topology-based Comparative optimization (FTC) method to generate weights for different meta-paths and then splits the original heterogeneous graph into different semantic subgraphs based on meta-paths. Thereafter, the different subgraphs are fused by the FTC to generate semantic fusion graphs that capture the interactions between different semantics. Semantic strength and feature similarity perspectives generate node embeddings from the semantic fusion graphs. Finally, the FTC positive and negative samples are used for contrastive learning from the two perspectives to yield the final node embeddings. Extensive experiments are conducted on three real datasets using the proposed HetGNN-SF model; the results reveal that HetGNN-SF outperforms state-of-the-art models. Our data and code are available on GitHub ( https://github.com/LiuXMaa/HetGNN-SF-.git ).
更多查看译文
关键词
Heterogeneous graph neural network,Contrastive learning,Self-supervised learning
AI 理解论文
溯源树
样例
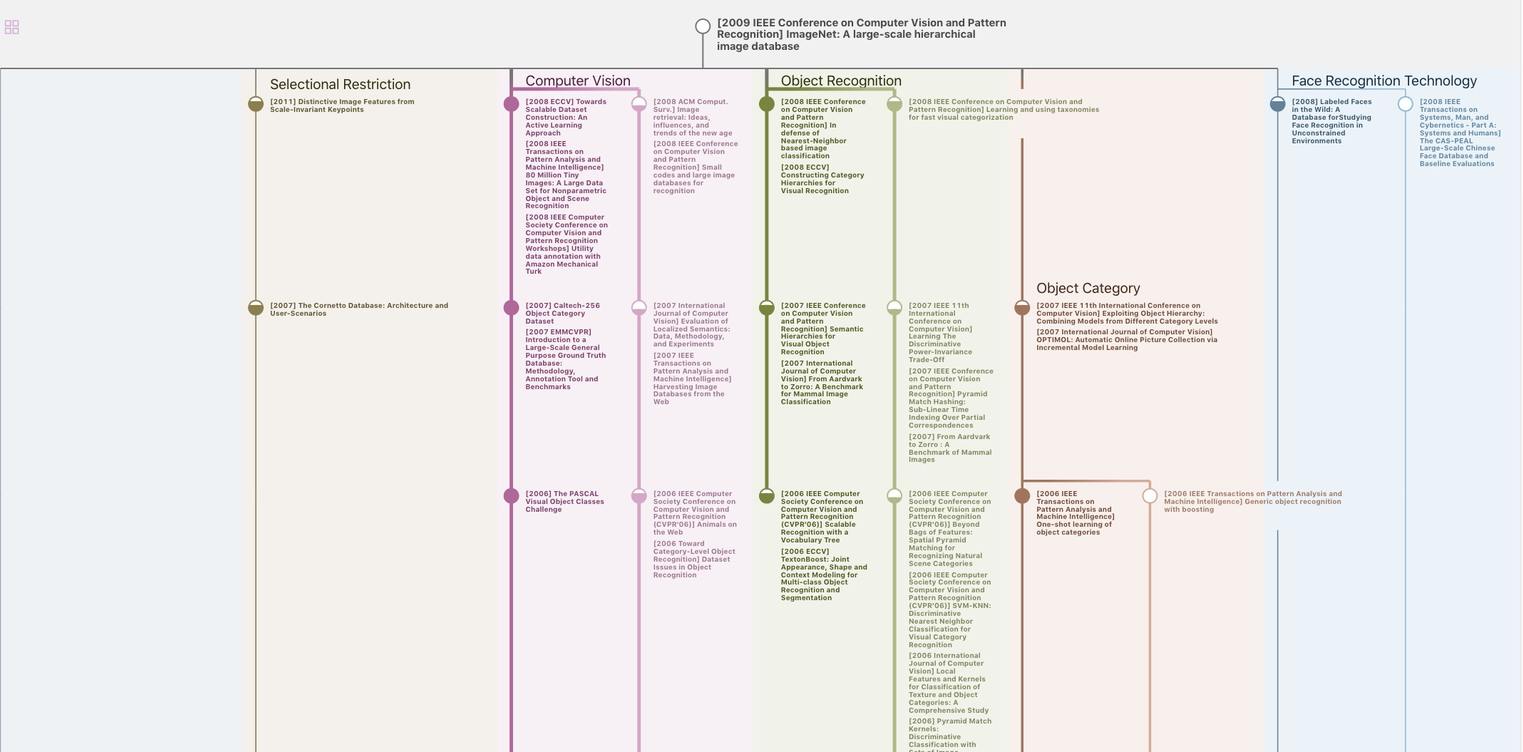
生成溯源树,研究论文发展脉络
Chat Paper
正在生成论文摘要