Machine Learning-Directed Predictive Models: Deciphering Complex Energy Transfer in Mn-Doped CsPb(Cl1-y Br y )3 Perovskite Nanocrystals
CHEMISTRY OF MATERIALS(2023)
摘要
Leadhalide perovskite nanocrystals with inclusion ofa transition-metaldopant of Mn2+ offer a substantial degree of freedom tomodulate the optoelectronic and magnetic properties owing to the introduceddopant in the host lattices. However, complexity as a result of theexcited interactions between the exciton and dopant, involving dynamicsof exciton recombination, competing forward and backward energy transfer(and vice versa), and Mn recombination, makes it difficult to understandand predict the Mn sensitization. Here, we have created machine learning-directedmodels using different nonlinear algorithms with initial 86 samplesto decipher the complex energy transfer by navigating the reactiondesign space of various concentrations of Mn along with differenthalide compositions (band gap) in Mn-doped CsPb(Cl1-y Br y )(3) nanocrystals.K-nearest neighbor-based predictive models coupled with time-correlatedsingle photon counting measurements allow for fully elucidating thecomplex and competing energy transfer kinetics occurring in two differentMn concentration regimes. Importantly, forward exciton-to-Mn energytransfer is more governed by the Mn concentration, while the backwardMn-to-exciton energy transfer is strongly dependent on the energygap difference between the exciton and Mn energy state. This machinelearning-guided approach and modeling can not only provide an efficientmeans for navigating the vast reaction design space but also providesignificant insight into understanding and elucidating the complexphysical phenomena throughout analyzing and predicting the datasettrend.
更多查看译文
关键词
perovskite,complex energy transfer,predictive models,learning-directed,mn-doped
AI 理解论文
溯源树
样例
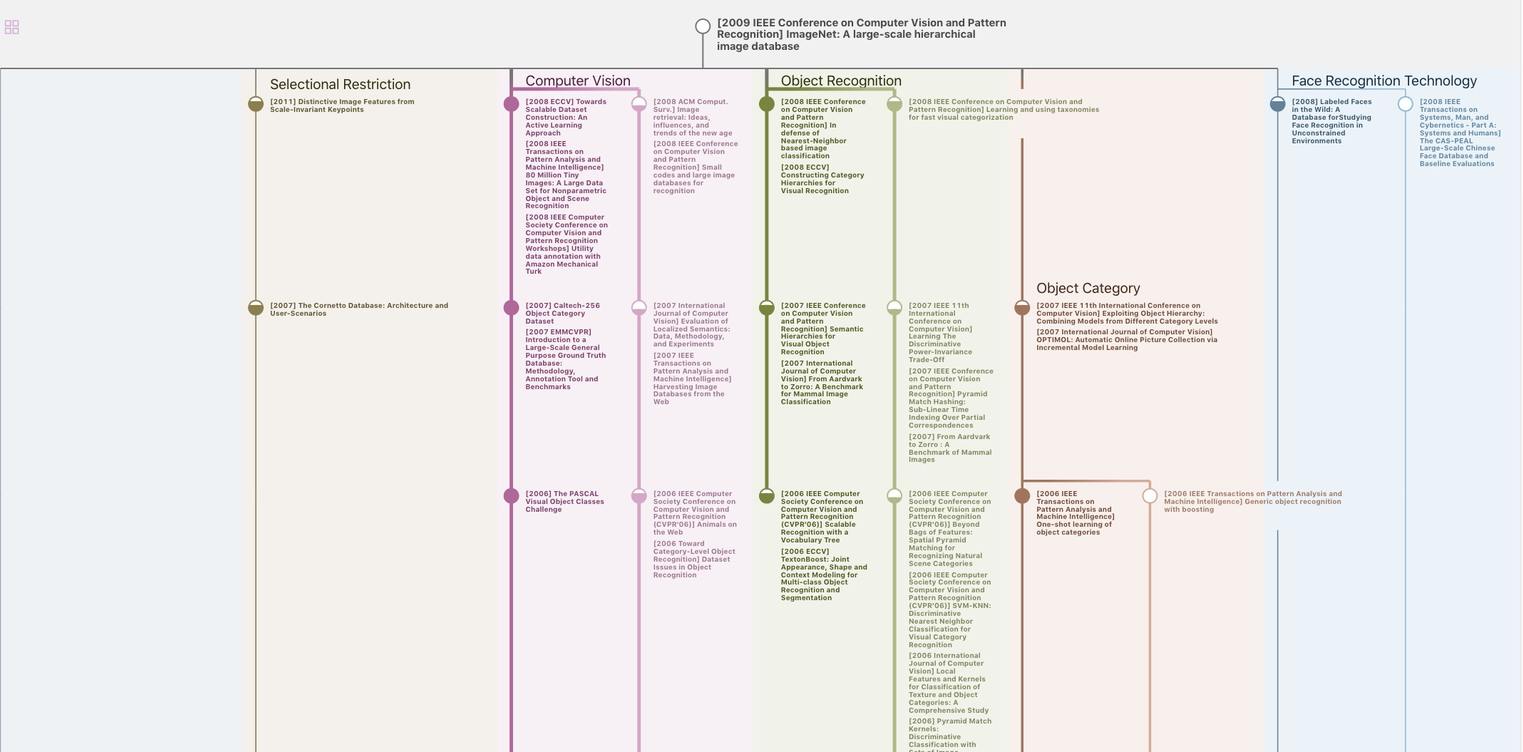
生成溯源树,研究论文发展脉络
Chat Paper
正在生成论文摘要