f-divergence and parametric regression models for compositional data
PAKISTAN JOURNAL OF STATISTICS AND OPERATION RESEARCH(2022)
摘要
The paper considers the class of f-divergence regression models as alternatives to parametric regression models (such as Dirichlet regression) for compositional data. The special cases examined in this paper include the Jensen-Shannon, Kullback-Leibler, Hellinger, chi(2) and total variation divergence. Strong advantages of the proposed regression models are a) the absence of parametric assumptions and b) the ability to treat zero values (which commonly occur in practice) naturally. Extensive Monte Carlo simulation studies comparatively assess the performance of the models in terms of bias and an empirical evaluation using real data examining further aspects, such as predictive performance and computational cost. The results reveal that Kullback-Leibler and Jensen-Shannon divergence regression models exhibited high quality performance in multiple directions. Ultimately, penalized versions of the Kullback-Leibler divergence regression are introduced and illustrated using real data rendering this model the optimal model to utilise in practice.
更多查看译文
关键词
compositional data, f-divergence regression models, penalized regression models, Dirichlet regression
AI 理解论文
溯源树
样例
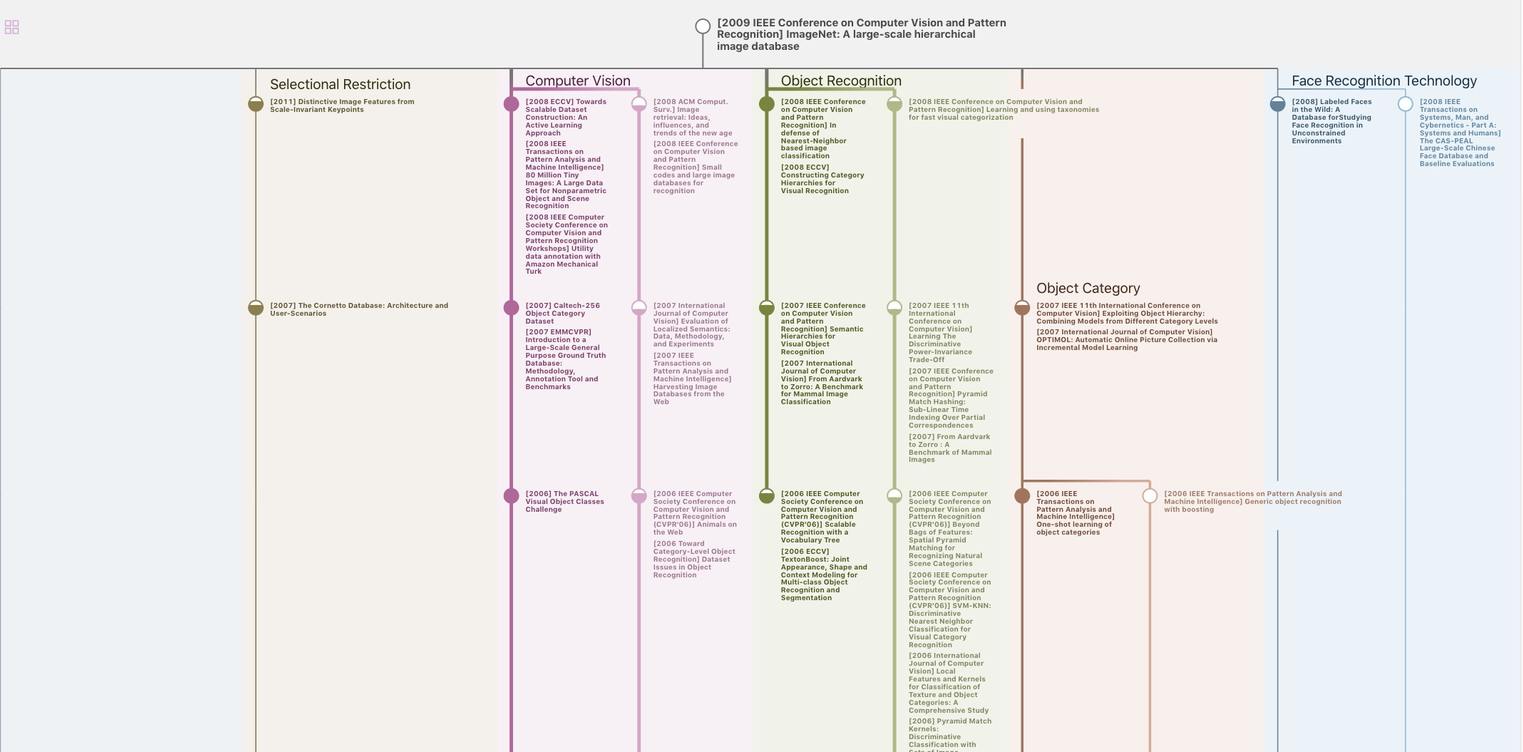
生成溯源树,研究论文发展脉络
Chat Paper
正在生成论文摘要