Comparing Manually Added Research Labels and Automatically Extracted Research Keywords to Identify Specialist Researchers in Learning Analytics: A Case Study Using Google Scholar Researcher Profiles
APPLIED SCIENCES-BASEL(2023)
摘要
Google Scholar (GS) has an interesting feature that allows researchers to manually assign certain research keywords to their profiles, referred to as research labels. These research labels may be used to find out and filter relevant resources, such as publications and authors. However, using manually appended research labels for identification may have limitations in terms of consistency, timeliness, objectivity, and mischaracterization. This paper aims to explore the difference between manually assigned research labels and automatically extracted keywords for identifying specialist Learning Analytics (LA) researchers. For this study, data were collected on 4732 publications from 1236 authors displaying "Learning Analytics" in their public GS profile labels, using their most cited publications since 2011. Our analysis methodology involved various text-mining techniques such as cosine similarity and text matching. The results showed that 446 of the 1236 authors were specialist researchers, 643 were occasional researchers, and 90 were interested researchers. The most interesting finding, using our methodology, was identifying 10 early career researchers independent of their GS citation count. Overall, while manually added research labels may provide some useful information about an author's research interests, they should be used with caution and in conjunction with another source of information such as automatically extracted keywords to identify accurately specialist learning analytics researchers.
更多查看译文
关键词
learning analytics, NLP, text analytics
AI 理解论文
溯源树
样例
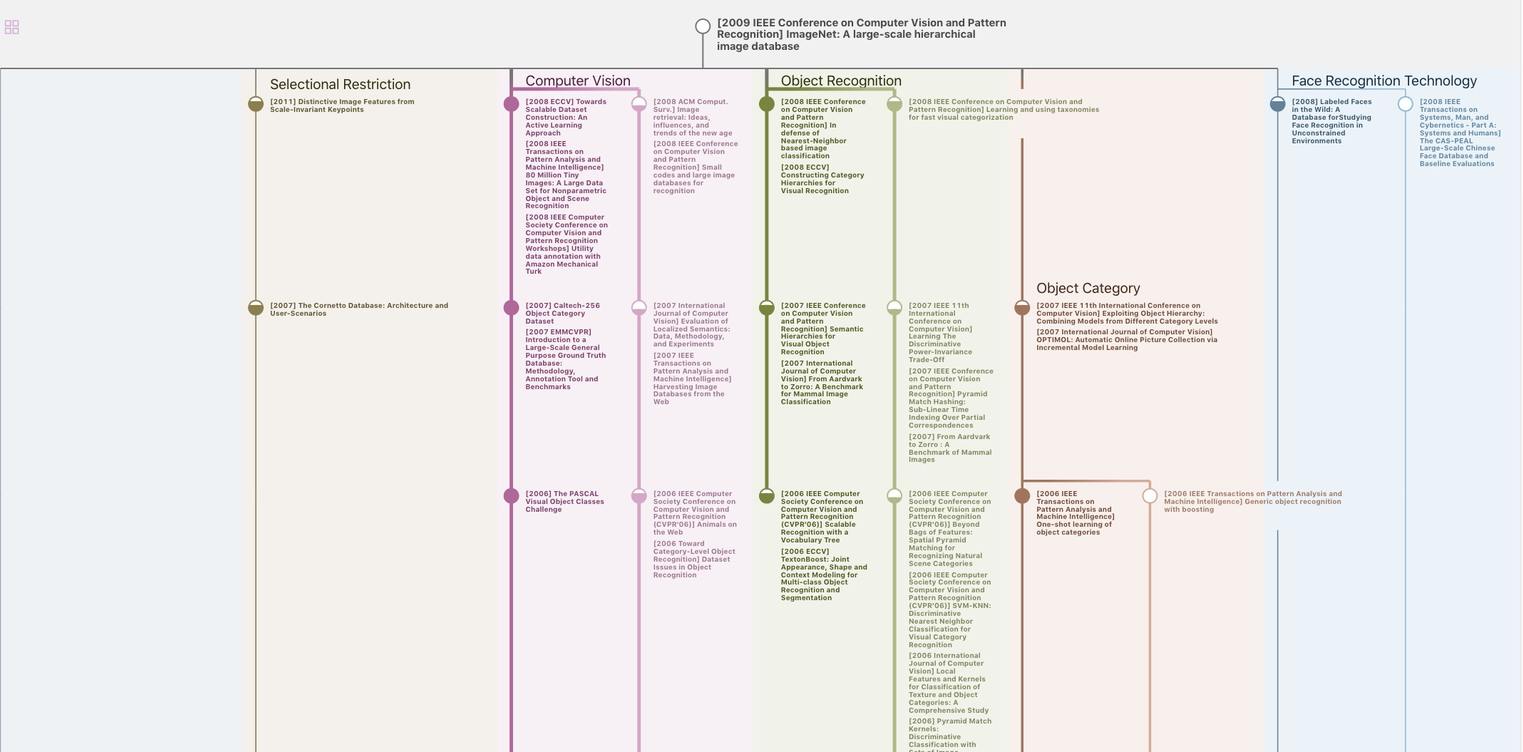
生成溯源树,研究论文发展脉络
Chat Paper
正在生成论文摘要