HGNNLDA: Predicting lncRNA-Drug Sensitivity Associations via a Dual Channel Hypergraph Neural Network
IEEE-ACM TRANSACTIONS ON COMPUTATIONAL BIOLOGY AND BIOINFORMATICS(2023)
摘要
Drug sensitivity is critical for enabling personalized treatment. Many studies have shown that long non-coding RNAs (lncRNAs) are closely related to drug sensitivity because lncRNAs can regulate genes related to drug sensitivity to affect drug efficacy. Exploring lncRNA-drug sensitivity associations has important implications for drug development and disease treatment. However, identifying lncRNA-drug sensitivity associations based on traditional biological approaches is small-scale and time-consuming. In this work, we develop a dual-channel hypergraph neural network-based method named HGNNLDA to infer unknown lncRNA-drug sensitivity associations. To our best knowledge, HGNNLDA is the first computational framework to predict lncRNA-drug sensitivity associations. HGNNLDA applies the hypergraph neural network to obtain high-order neighbor information on the lncRNA hypergraph and the drug hypergraph, respectively, and utilizes a joint update mechanism to generate lncRNA embeddings and drug embeddings. In traditional graphs, an edge contains only two nodes. However, hyperedges in hypergraphs can contain any number of nodes and hypergraphs can well describe the higher-order connectivity of the lncRNA-drug bipartite graphs. The comprehensive experimental results show that HGNNLDA significantly outperforms the other six state-of-the-art models. Case studies on two drugs further illustrate that HGNNLDA is an effective tool to predict lncRNA-drug sensitivity associations.
更多查看译文
关键词
Bipartite graph,hypergraph neural network,lncRNA-drug sensitivity associations
AI 理解论文
溯源树
样例
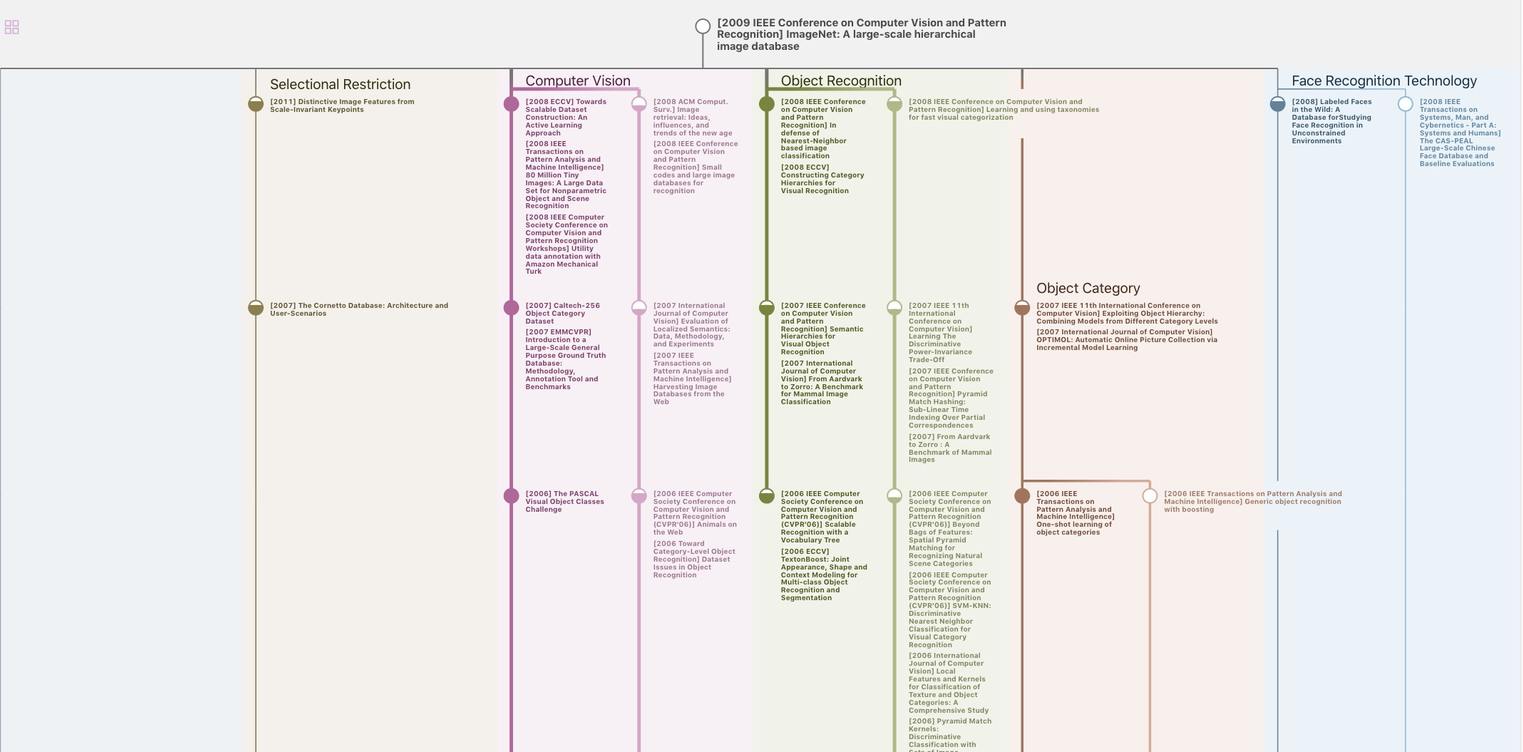
生成溯源树,研究论文发展脉络
Chat Paper
正在生成论文摘要