Application-Oriented Benchmarking of Quantum Generative Learning Using QUARK
CoRR(2023)
摘要
Benchmarking of quantum machine learning (QML) algorithms is challenging due to the complexity and variability of QML systems, e. g., regarding model ansatzes, data sets, training techniques, and hyper-parameter selection. The QUantum computing Application benchmaRK (QUARK) framework simplifies and standardizes benchmarking studies for quantum computing applications. Here, we propose several extensions of QUARK to include the ability to evaluate the training and deployment of quantum generative models. We describe the updated software architecture and illustrate its flexibility through several example applications: (1) We trained different quantum generative models using several circuit ansatzes, data sets, and data transformations. (2) We evaluated our models on GPU and real quantum hardware. (3) We assessed the generalization capabilities of our generative models using a broad set of metrics that capture, e. g., the novelty and validity of the generated data.
更多查看译文
关键词
quantum computing,benchmark,machine learning,generative modeling
AI 理解论文
溯源树
样例
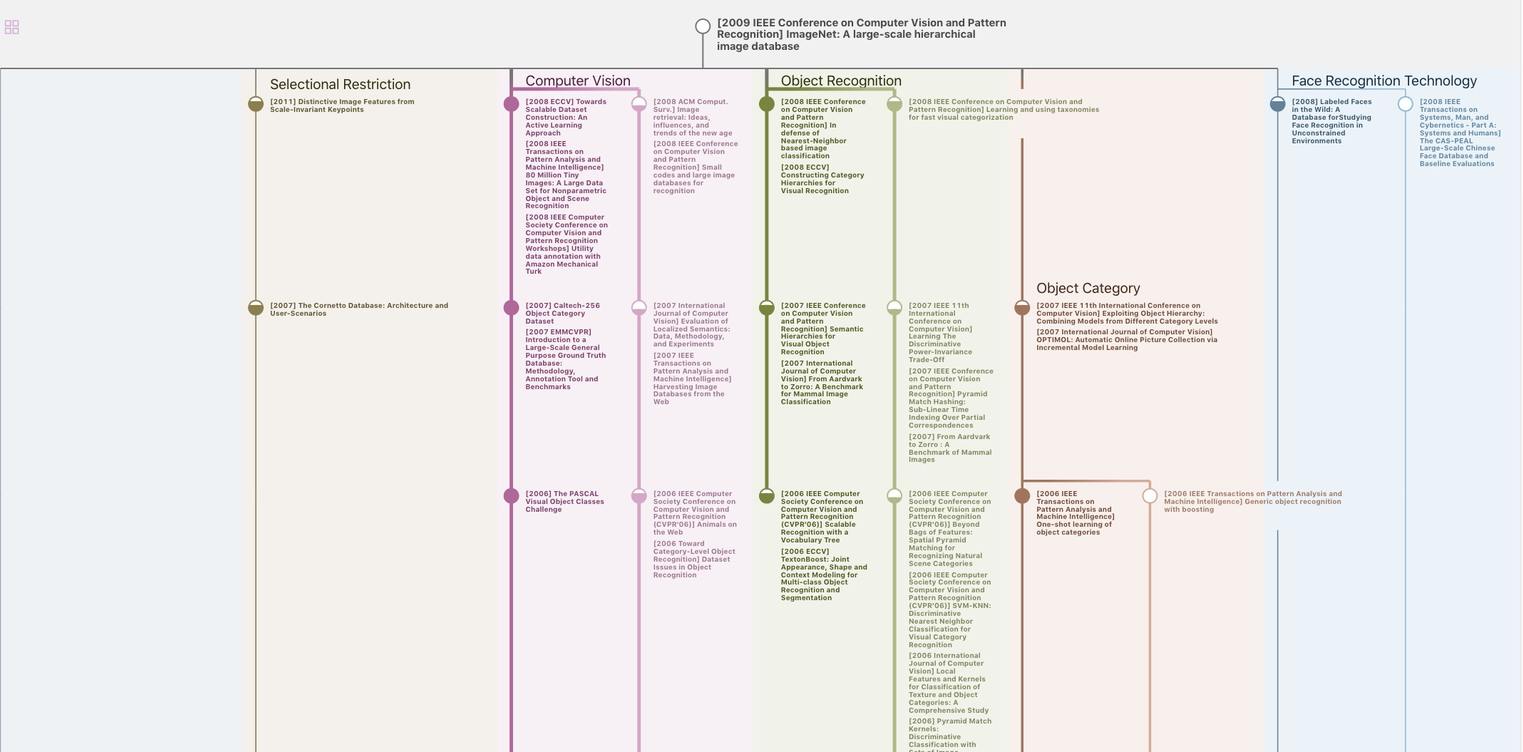
生成溯源树,研究论文发展脉络
Chat Paper
正在生成论文摘要