Real-time Strawberry Detection Based on Improved YOLOv5s Architecture for Robotic Harvesting in open-field environment
CoRR(2023)
摘要
This study proposed a YOLOv5-based custom object detection model to detect strawberries in an outdoor environment. The original architecture of the YOLOv5s was modified by replacing the C3 module with the C2f module in the backbone network, which provided a better feature gradient flow. Secondly, the Spatial Pyramid Pooling Fast in the final layer of the backbone network of YOLOv5s was combined with Cross Stage Partial Net to improve the generalization ability over the strawberry dataset in this study. The proposed architecture was named YOLOv5s-Straw. The RGB images dataset of the strawberry canopy with three maturity classes (immature, nearly mature, and mature) was collected in open-field environment and augmented through a series of operations including brightness reduction, brightness increase, and noise adding. To verify the superiority of the proposed method for strawberry detection in open-field environment, four competitive detection models (YOLOv3-tiny, YOLOv5s, YOLOv5s-C2f, and YOLOv8s) were trained, and tested under the same computational environment and compared with YOLOv5s-Straw. The results showed that the highest mean average precision of 80.3% was achieved using the proposed architecture whereas the same was achieved with YOLOv3-tiny, YOLOv5s, YOLOv5s-C2f, and YOLOv8s were 73.4%, 77.8%, 79.8%, 79.3%, respectively. Specifically, the average precision of YOLOv5s-Straw was 82.1% in the immature class, 73.5% in the nearly mature class, and 86.6% in the mature class, which were 2.3% and 3.7%, respectively, higher than that of the latest YOLOv8s. The model included 8.6*10^6 network parameters with an inference speed of 18ms per image while the inference speed of YOLOv8s had a slower inference speed of 21.0ms and heavy parameters of 11.1*10^6, which indicates that the proposed model is fast enough for real time strawberry detection and localization for the robotic picking.
更多查看译文
关键词
robotic harvesting,improved yolov5s architecture
AI 理解论文
溯源树
样例
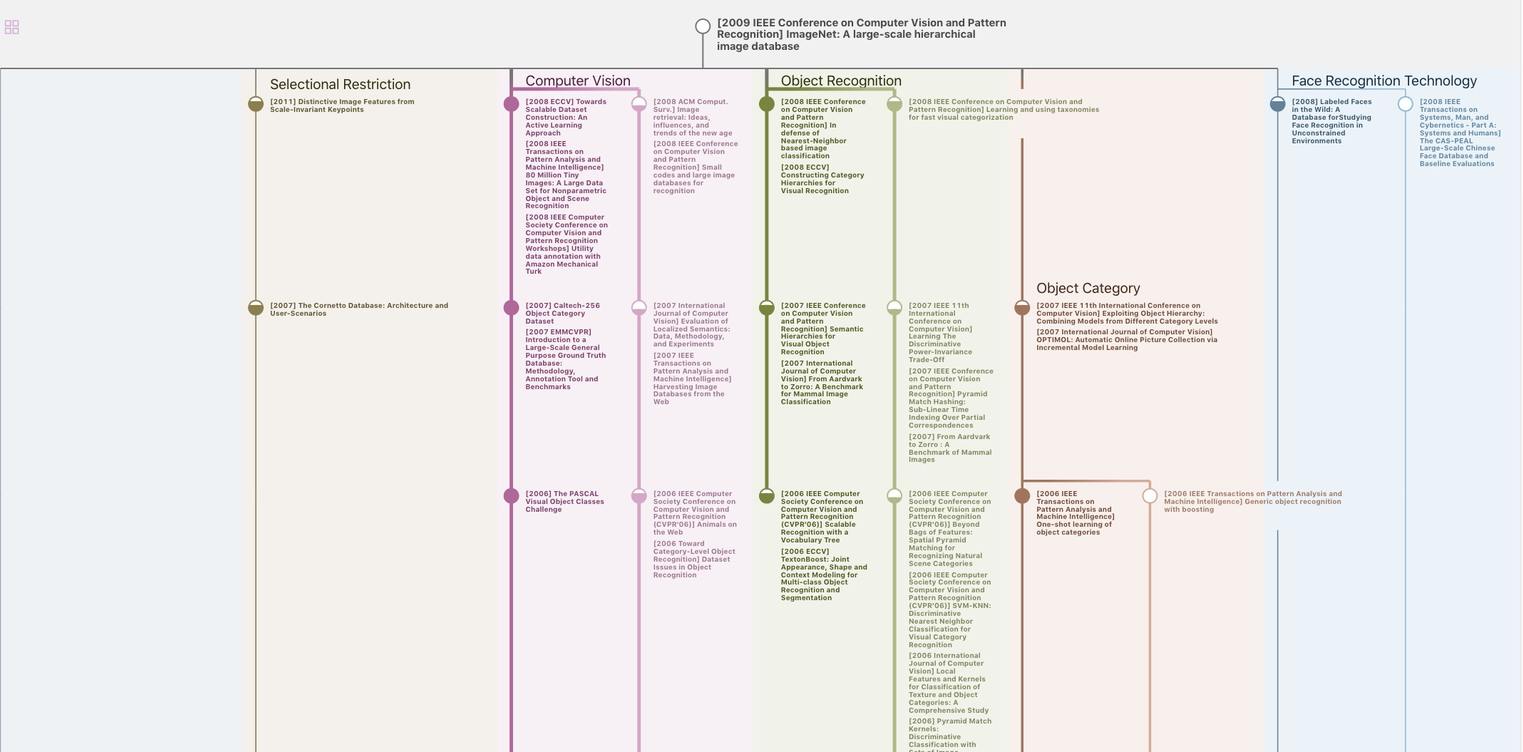
生成溯源树,研究论文发展脉络
Chat Paper
正在生成论文摘要