PUG: Photorealistic and Semantically Controllable Synthetic Data for Representation Learning
NeurIPS(2023)
摘要
Synthetic image datasets offer unmatched advantages for designing and evaluating deep neural networks: they make it possible to (i) render as many data samples as needed, (ii) precisely control each scene and yield granular ground truth labels (and captions), (iii) precisely control distribution shifts between training and testing to isolate variables of interest for sound experimentation. Despite such promise, the use of synthetic image data is still limited -- and often played down -- mainly due to their lack of realism. Most works therefore rely on datasets of real images, which have often been scraped from public images on the internet, and may have issues with regards to privacy, bias, and copyright, while offering little control over how objects precisely appear. In this work, we present a path to democratize the use of photorealistic synthetic data: we develop a new generation of interactive environments for representation learning research, that offer both controllability and realism. We use the Unreal Engine, a powerful game engine well known in the entertainment industry, to produce PUG (Photorealistic Unreal Graphics) environments and datasets for representation learning. In this paper, we demonstrate the potential of PUG to enable more rigorous evaluations of vision models.
更多查看译文
关键词
semantically controllable synthetic data,representation,learning
AI 理解论文
溯源树
样例
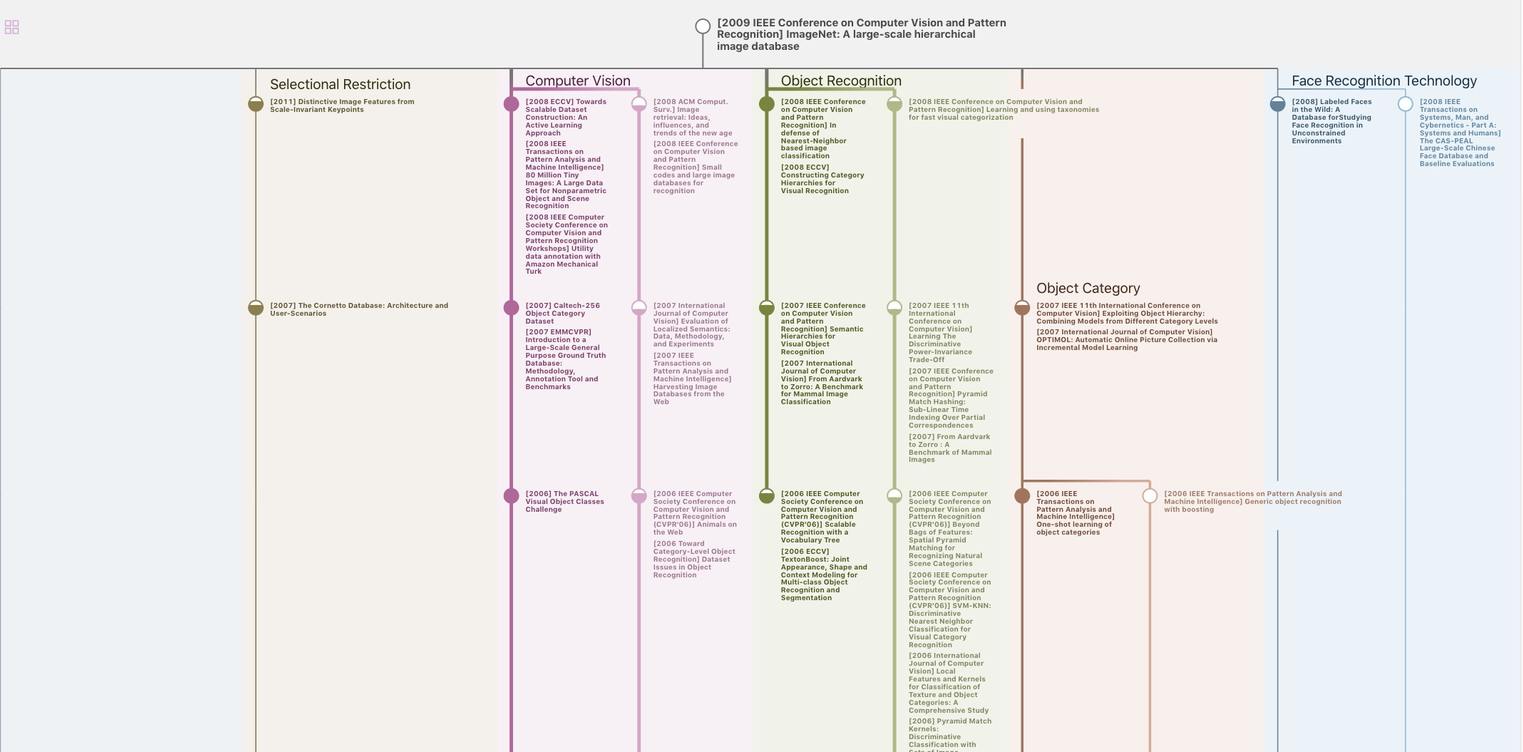
生成溯源树,研究论文发展脉络
Chat Paper
正在生成论文摘要