Exploiting Generalization in Offline Reinforcement Learning via Unseen State Augmentations
CoRR(2023)
摘要
Offline reinforcement learning (RL) methods strike a balance between exploration and exploitation by conservative value estimation -- penalizing values of unseen states and actions. Model-free methods penalize values at all unseen actions, while model-based methods are able to further exploit unseen states via model rollouts. However, such methods are handicapped in their ability to find unseen states far away from the available offline data due to two factors -- (a) very short rollout horizons in models due to cascading model errors, and (b) model rollouts originating solely from states observed in offline data. We relax the second assumption and present a novel unseen state augmentation strategy to allow exploitation of unseen states where the learned model and value estimates generalize. Our strategy finds unseen states by value-informed perturbations of seen states followed by filtering out states with epistemic uncertainty estimates too high (high error) or too low (too similar to seen data). We observe improved performance in several offline RL tasks and find that our augmentation strategy consistently leads to overall lower average dataset Q-value estimates i.e. more conservative Q-value estimates than a baseline.
更多查看译文
关键词
offline reinforcement learning,state augmentations,generalization,unseen
AI 理解论文
溯源树
样例
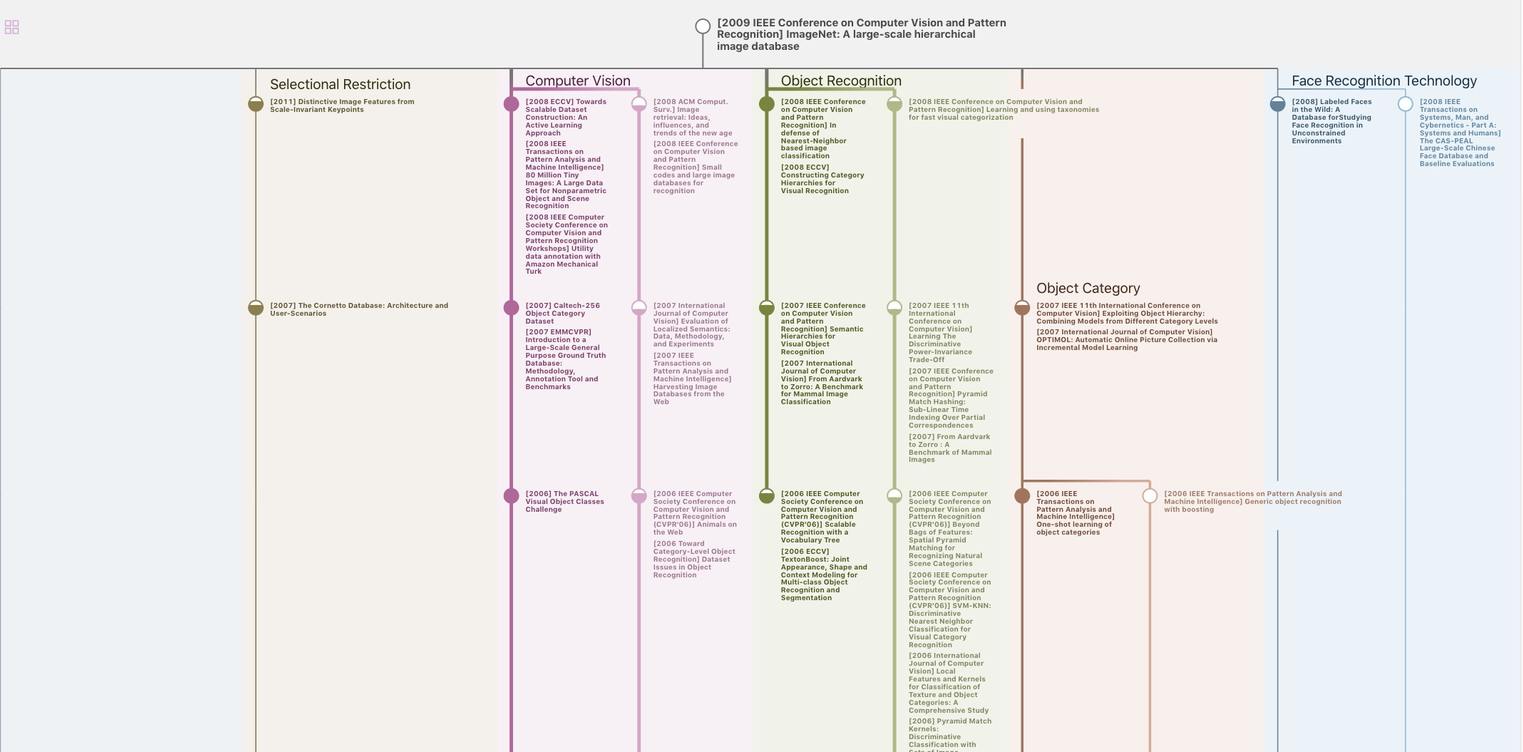
生成溯源树,研究论文发展脉络
Chat Paper
正在生成论文摘要