Goodness-of-Fit of Attributed Probabilistic Graph Generative Models
CoRR(2023)
摘要
Probabilistic generative models of graphs are important tools that enable representation and sampling. Many recent works have created probabilistic models of graphs that are capable of representing not only entity interactions but also their attributes. However, given a generative model of random attributed graph(s), the general conditions that establish goodness of fit are not clear a-priori. In this paper, we define goodness of fit in terms of the mean square contingency coefficient for random binary networks. For this statistic, we outline a procedure for assessing the quality of the structure of a learned attributed graph by ensuring that the discrepancy of the mean square contingency coefficient (constant, or random) is minimal with high probability. We apply these criteria to verify the representation capability of a probabilistic generative model for various popular types of graph models.
更多查看译文
关键词
probabilistic,graph,models,goodness-of-fit
AI 理解论文
溯源树
样例
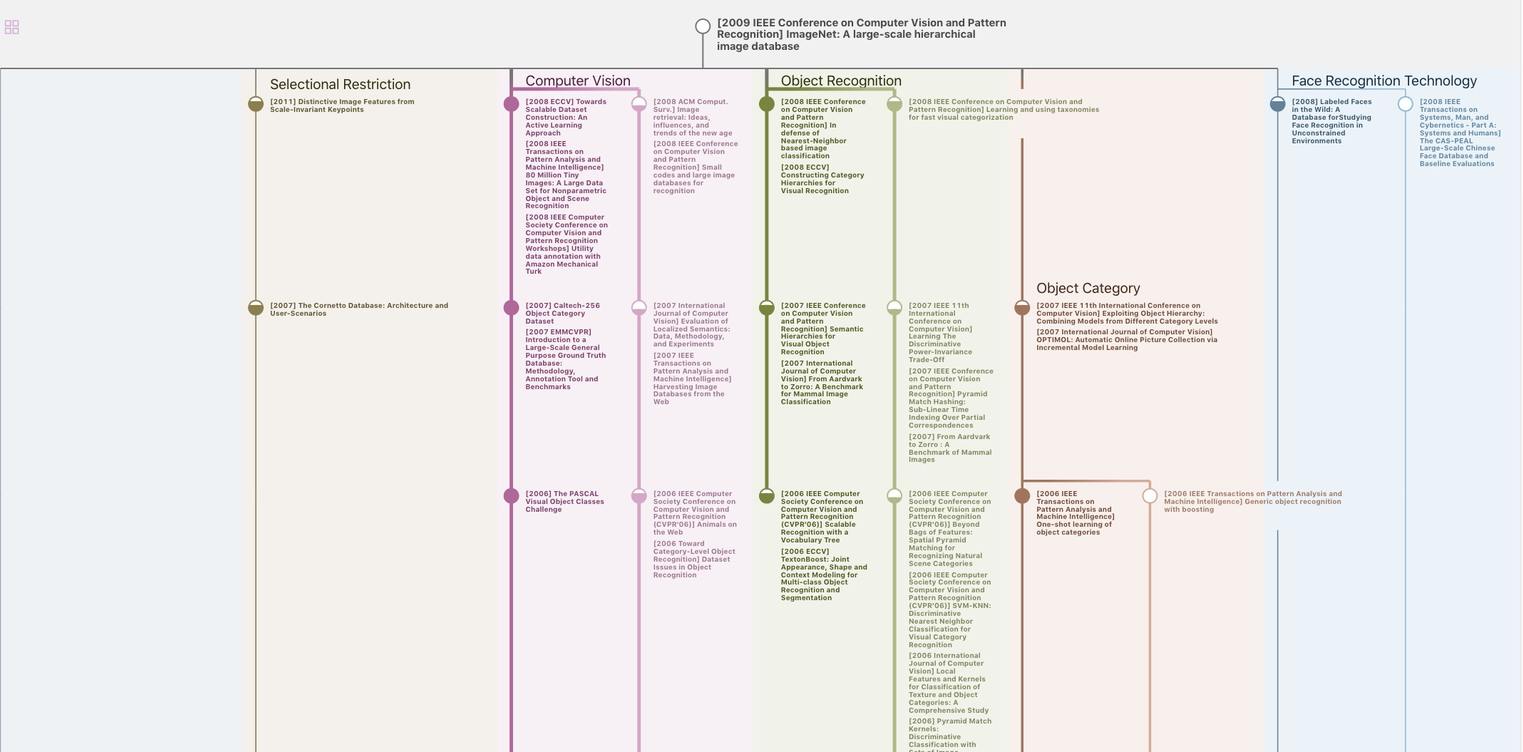
生成溯源树,研究论文发展脉络
Chat Paper
正在生成论文摘要